Joint Label Propagation, Graph and Latent Subspace Estimation for Semi-supervised Classification
Cognitive Computation(2024)
摘要
Obtaining labeled images and samples is a very expensive process and can require intensive labor. At the same time, there are often not enough labeled samples to train an effective classifier. Graph-based semi-supervised methods have attracted much attention in the field because they can use both labeled and unlabeled data. In this letter, we suggest a novel graph-based semi-supervised learning approach that takes full advantage of a small set of labeled samples and a large set of unlabeled samples. We first explain the concept of graph-based semi-supervised learning. Our central proposal is to jointly estimate a low-rank graph together with a latent subspace and soft labels. The proposed system exploits the synergy between the graph information and the latent data representation. This extends and enriches the supervision information in the semi-supervised context and produces a good discriminative linear mapping between the original space and the latent subspace. Several experiments were performed with five image datasets using state-of-the-art methods. The results of the study reveal several noteworthy findings. The proposed framework generally outperforms recent competing approaches in estimating labels and linear embeddings, especially for large datasets such as MNIST. The superiority is maintained even for minimally labeled images, but the recognition accuracy does not always increase. However, in a few cases, the proposed method may be outperformed by other method when the number of labeled samples per class is only one (the dataset UMIST). When we set the number of labeled samples per class to 2, we find that the proposed method consistently performs better than the competing methods. For the extended Yale dataset, the accuracy of the proposed method is 67.08%. This is 1.68% higher than the accuracy of the nearest competing method and an impressive 30.08% higher than the accuracy of the farthest competing method. In the case of the FacePix dataset, the accuracy of the proposed method is 58.08%. This is 2.58% higher than the nearest competing method and 13.58% higher than the farthest competing method. For the UMIST dataset, the accuracy reaches 54.2%, outperforming the nearest competing method by 0.9% and the farthest competing method by 7.5%. We have introduced a novel graph-based semi-supervised method capable of jointly predicting the soft labels and linear embedding and constructing a data graph. The main contribution in this work is the integration of the graph estimation into the objective function of the model. By computing the graph structure while estimating the semi-supervised model, a more optimal solution can be obtained. The synergistic use of data features and adaptive soft labels has indeed contributed to the estimation of a good discriminant model.
更多查看译文
关键词
Semi-supervised learning,Discriminant embedding,Soft labels,Graph construction,Graph-based embedding,Image categorization,Pattern recognition
AI 理解论文
溯源树
样例
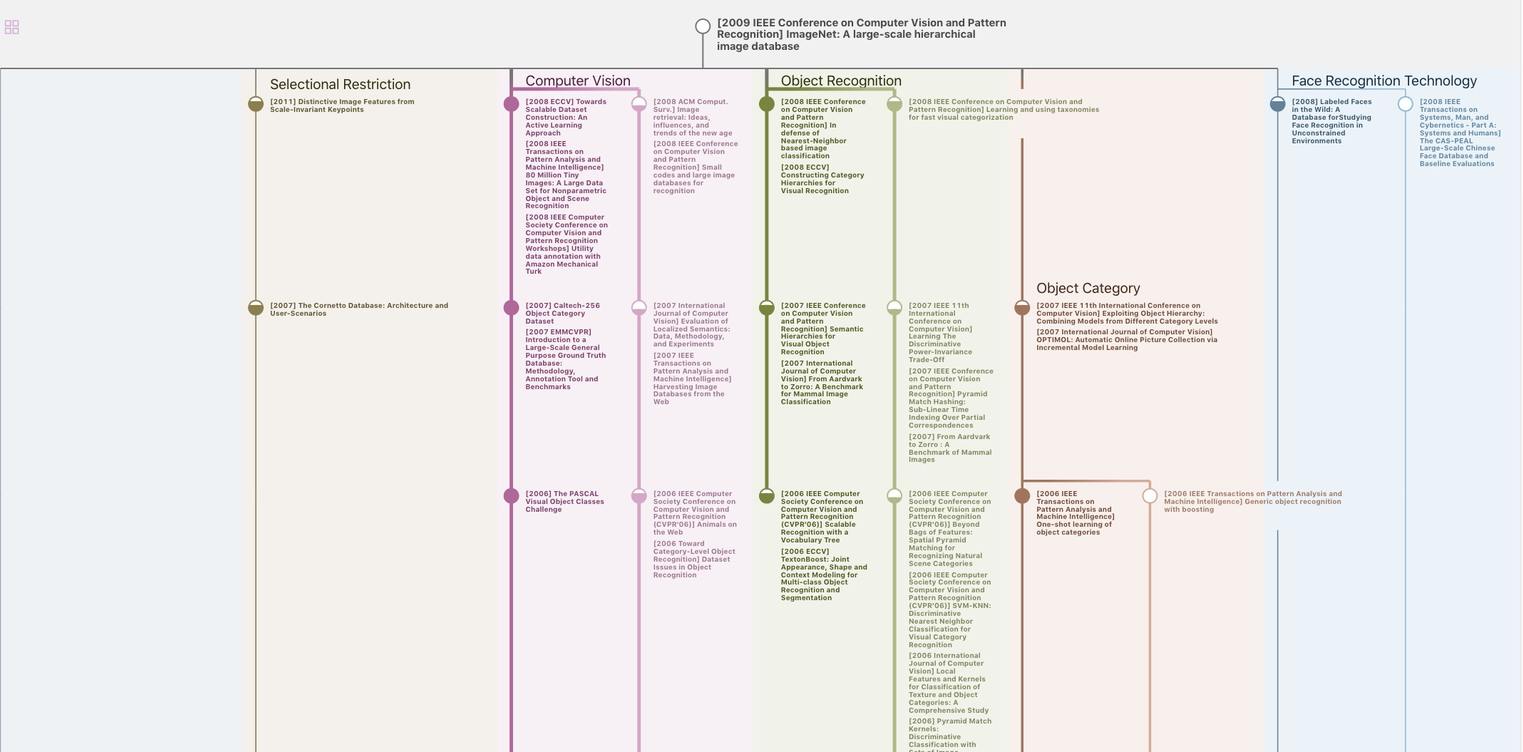
生成溯源树,研究论文发展脉络
Chat Paper
正在生成论文摘要