Improving on mapping long-term surface water with a novel framework based on the Landsat imagery series.
Journal of environmental management(2024)
摘要
Surface water plays a crucial role in the ecological environment and societal development. Remote sensing detection serves as a significant approach to understand the temporal and spatial change in surface water series (SWS) and to directly construct long-term SWS. Limited by various factors such as cloud, cloud shadow, and problematic satellite sensor monitoring, the existent surface water mapping datasets might be short and incomplete due to losing raw information on certain dates. Improved algorithms are desired to increase the completeness and quality of SWS datasets. The present study proposes an automated framework to detect SWS, based on the Google Earth Engine and Landsat satellite imagery. This framework incorporates implementing a raw image filtering algorithm to increase available images, thereby expanding the completeness. It improves OTSU thresholding by replacing anomaly thresholds with the median value, thus enhancing the accuracy of SWS datasets. Gaps caused by Landsat7 ETM + SLC-off are respired with the random forest algorithm and morphological operations. The results show that this novel framework effectively expands the long-term series of SWS for three surface water bodies with distinct geomorphological patterns. The evaluation of confusion matrices suggests the good performance of extracting surface water, with the overall accuracy ranging from 0.96 to 0.97, and user's accuracy between 0.96 and 0.98, producer's accuracy ranging from 0.83 to 0.89, and Matthews correlation coefficient ranging from 0.87 to 0.9 for several spectral water indices (NDWI, MNDWI, ANNDWI, and AWEI). Compared with the Global Reservoirs Surface Area Dynamics (GRSAD) dataset, our constructed datasets promote greater completeness of SWS datasets by 27.01%-91.89% for the selected water bodies. The proposed framework for detecting SWS shows good potential in enlarging and completing long-term global-scale SWS datasets, capable of supporting assessments of surface-water-related environmental management and disaster prevention.
更多查看译文
AI 理解论文
溯源树
样例
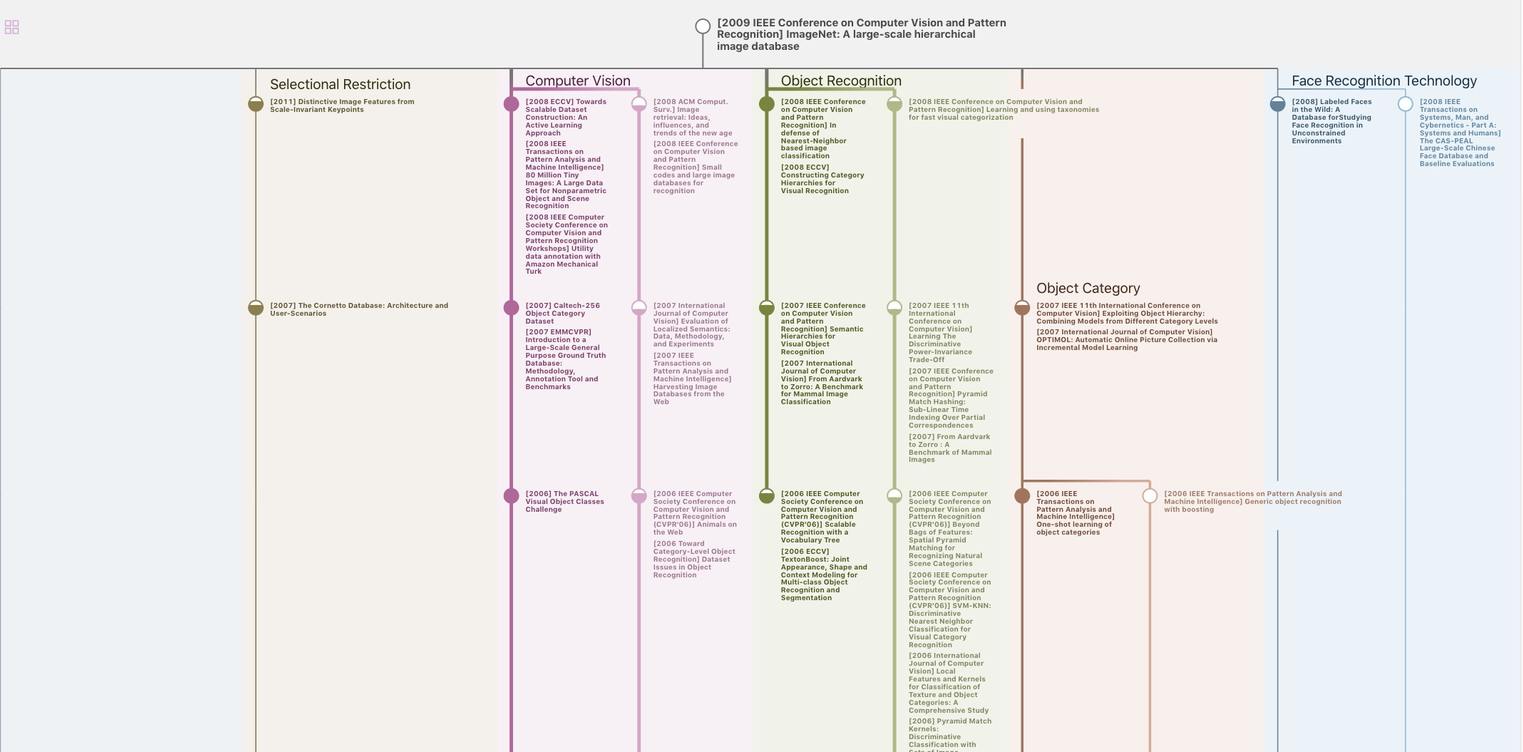
生成溯源树,研究论文发展脉络
Chat Paper
正在生成论文摘要