Objective and Subjective Evaluation of Speech Enhancement Methods in the UDASE Task of the 7th CHiME Challenge
Computer Speech & Language(2025)
摘要
Supervised models for speech enhancement are trained using artificiallygenerated mixtures of clean speech and noise signals. However, the synthetictraining conditions may not accurately reflect real-world conditionsencountered during testing. This discrepancy can result in poor performancewhen the test domain significantly differs from the synthetic training domain.To tackle this issue, the UDASE task of the 7th CHiME challenge aimed toleverage real-world noisy speech recordings from the test domain forunsupervised domain adaptation of speech enhancement models. Specifically, thistest domain corresponds to the CHiME-5 dataset, characterized by realmulti-speaker and conversational speech recordings made in noisy andreverberant domestic environments, for which ground-truth clean speech signalsare not available. In this paper, we present the objective and subjectiveevaluations of the systems that were submitted to the CHiME-7 UDASE task, andwe provide an analysis of the results. This analysis reveals a limitedcorrelation between subjective ratings and several supervised nonintrusiveperformance metrics recently proposed for speech enhancement. Conversely, theresults suggest that more traditional intrusive objective metrics can be usedfor in-domain performance evaluation using the reverberant LibriCHiME-5 datasetdeveloped for the challenge. The subjective evaluation indicates that allsystems successfully reduced the background noise, but always at the expense ofincreased distortion. Out of the four speech enhancement methods evaluatedsubjectively, only one demonstrated an improvement in overall quality comparedto the unprocessed noisy speech, highlighting the difficulty of the task. Thetools and audio material created for the CHiME-7 UDASE task are shared with thecommunity.
更多查看译文
关键词
CHiME challenge,Multi-speaker conversational speech,Speech enhancement,Unsupervised domain adaptation,ITU-T P.835 listening test
AI 理解论文
溯源树
样例
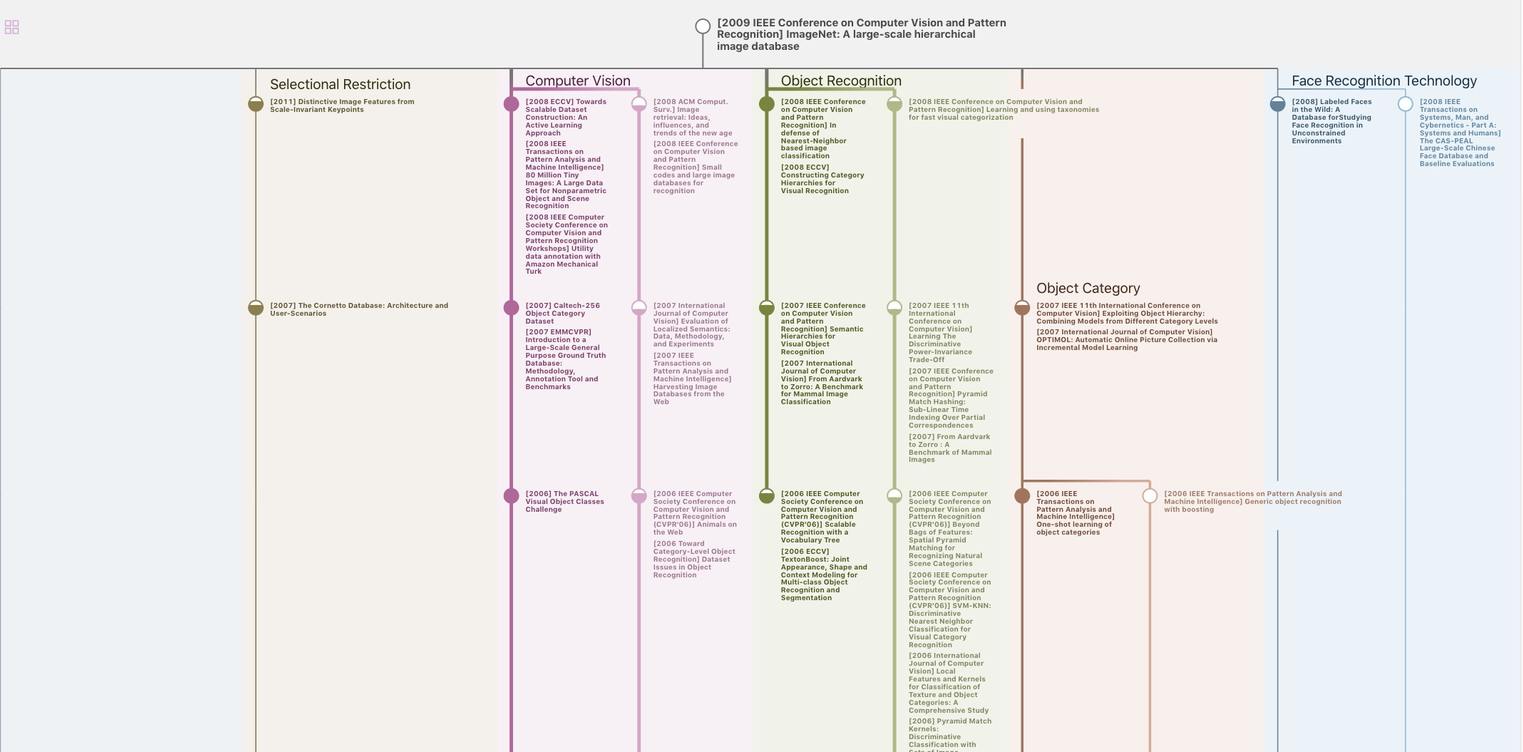
生成溯源树,研究论文发展脉络
Chat Paper
正在生成论文摘要