Deep learning path-like collective variable for enhanced sampling molecular dynamics
arxiv(2024)
摘要
Several enhanced sampling techniques rely on the definition of collective
variables to effectively explore free energy landscapes. Existing variables
that describe the progression along a reactive pathway offer an elegant
solution but face a number of limitations. In this paper, we address these
challenges by introducing a new path-like collective variable called the
`Deep-locally-non-linear-embedding', which is inspired by principles of the
locally linear embedding technique and is trained on a reactive trajectory. The
variable mimics the ideal reaction coordinate by automatically generating a
non-linear combination of features through a differentiable generalized
autoencoder that combines a neural network with a continuous k-nearest-neighbor
selection. Among the key advantages of this method is its capability to
automatically choose the metric for searching neighbors and to learn the path
from state A to state B without the need to handpick landmarks a priori. We
demonstrate the effectiveness of DeepLNE by showing that the progression along
the path variable closely approximates the ideal reaction coordinate in toy
models such as the Müller-Brown-potential and alanine dipeptide. We then use
it in molecular dynamics simulations of an RNA tetraloop, where we highlight
its capability to accelerate transitions and converge the free energy of
folding.
更多查看译文
AI 理解论文
溯源树
样例
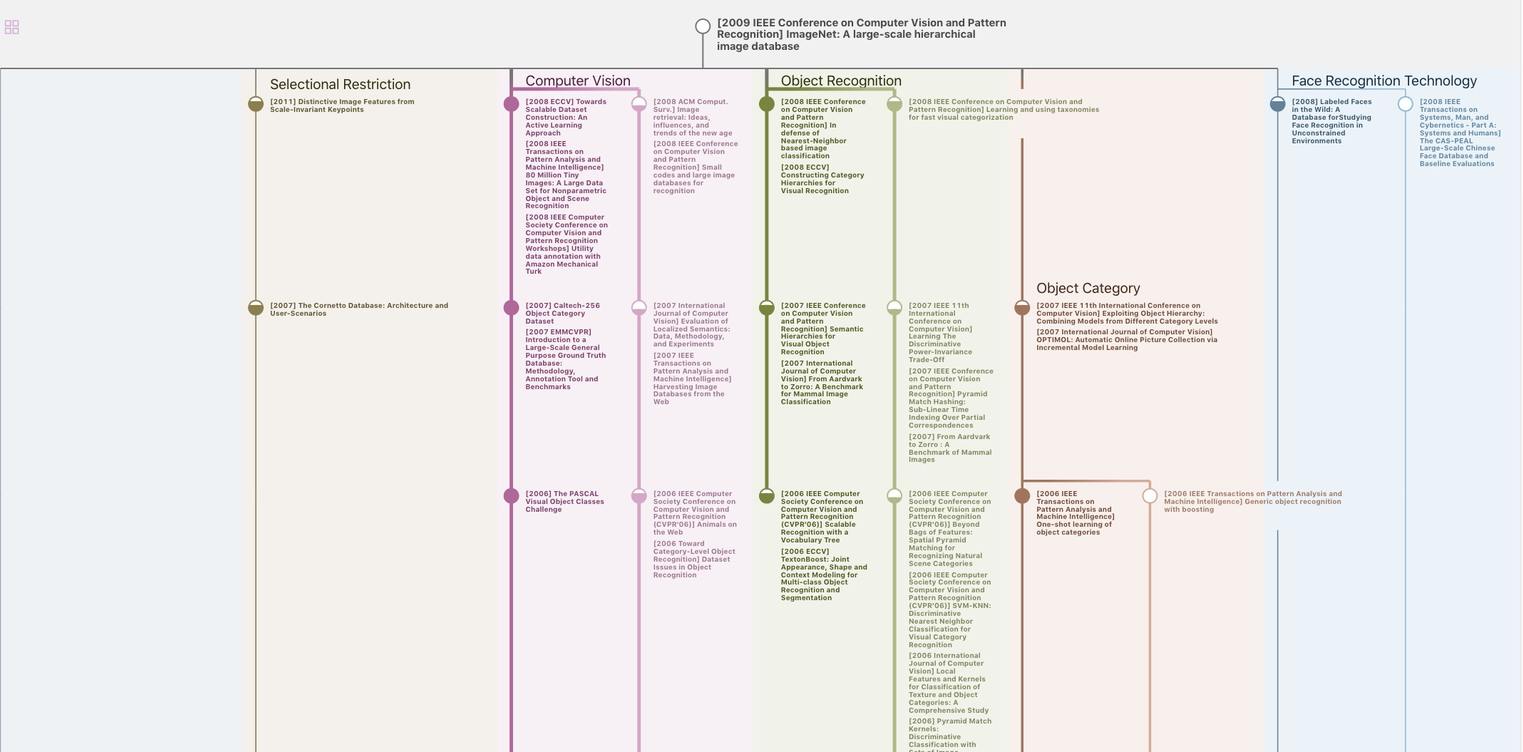
生成溯源树,研究论文发展脉络
Chat Paper
正在生成论文摘要