Prediction of CBR by Deep Artificial Neural Networks with Hyperparameter Optimization by Simulated Annealing
Indian Geotechnical Journal(2024)
摘要
The construction of pavements requires the complete identification of the soils in place and of the added materials. This identification consists in determining the class of the soils and in evaluating their bearing capacity through the California bearing ratio (CBR) index. Obtaining the CBR index is very costly in terms of time and financial resources, especially when it is a large-scale project. It thus leaves prospects of obtaining it by simpler processes; hence, it arises the need to find simpler processes compared to classical processes. This study develops models for predicting the CBR index from physical properties that are less complex to obtain, based on deep neural networks. To achieve this, three databases were used. A first database consists of the proportion of fines, the Atterberg limits and the Proctor references of the soils. A second database uses the methylene blue value instead of the Atterberg limits, and a third database uses only the proportion of fines and the Proctor soil reference. On each of the databases, a deep neural network model was developed using dense layers, regularization layers, residual blocks and parallelization in TensorFlow to predict the CBR value. Each model was formed by combining several deep neural networks developed according to specific architectures. To expedite training, the simulated annealing method was employed to optimize hyperparameters and define the optimal configuration for each network. The predictions obtained are correlated with the true values from 83.6 to 96.5%. In terms of performance, the models have a mean deviation ranging from 3.74 to 5.96%, a maximum deviation ranging from 12.43 to 16.2% and a squared deviation ranging from 0.781 to 2.189. The results suggest that the variable VBS has a negative impact on the accuracy of the networks in predicting the CBR index. The developed models respect the confidence threshold (± 10%) and can be used to set up a local or regional geotechnical platform.
更多查看译文
关键词
CBR prediction,Deep artificial neural networks,Clustering,Data augmentation,TensorFlow
AI 理解论文
溯源树
样例
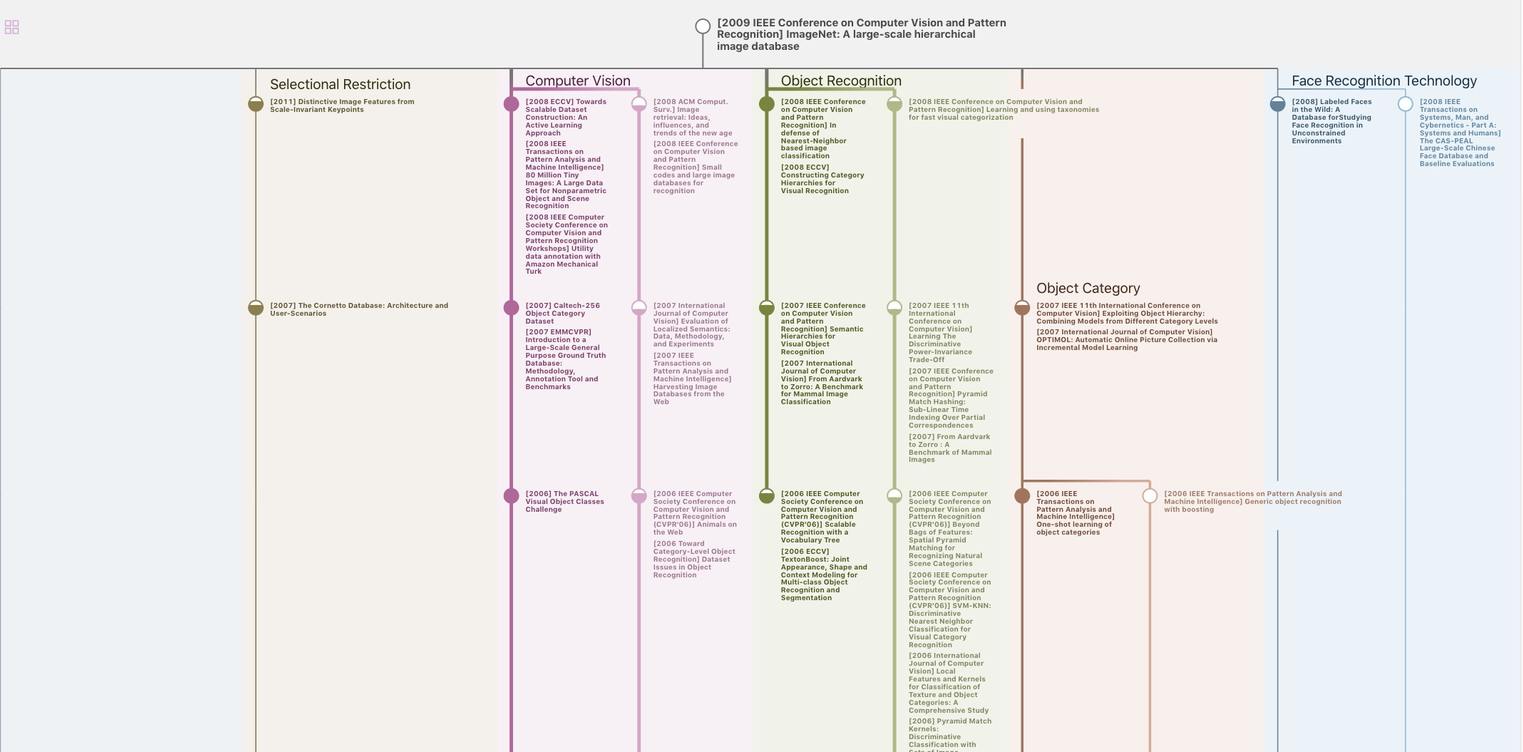
生成溯源树,研究论文发展脉络
Chat Paper
正在生成论文摘要