Variance Alignment Score: A Simple But Tough-to-Beat Data Selection Method for Multimodal Contrastive Learning
CoRR(2024)
摘要
In recent years, data selection has emerged as a core issue for large-scale
visual-language model pretraining, especially on noisy web-curated datasets.
One widely adopted strategy assigns quality scores such as CLIP similarity for
each sample and retains the data pairs with the highest scores. However, these
approaches are agnostic of data distribution and always fail to select the most
informative samples. To solve this problem, we propose a simple yet
theoretically principled metric named Variance Alignment Score (VAS), which has
the form ⟨Σ_test, Σ_i⟩. Here,
Σ_test represents the target (cross-)covariance matrix we aim
to align, potentially based on prior knowledge, while Σ_i denotes the
tensor product of single or multi-modal representations for the i-th sample.
We further design a new data selection method that maximizes the total VAS. We
provide theoretical analysis in a simplified setting to demonstrate the
theoretical advantage of VAS over random or other existing data selection.
Experimentally, applying VAS and CLIP scores together can outperform baselines
by a margin of 1.3% average on 38 evaluation sets for noisy dataset DataComp
and 2.5% on VTAB for high-quality dataset CC12M. Additionally, our ablation
study also shows visual features are better than text for calculating VAS, and
the related classical experimental design methods may fail under this context.
更多查看译文
AI 理解论文
溯源树
样例
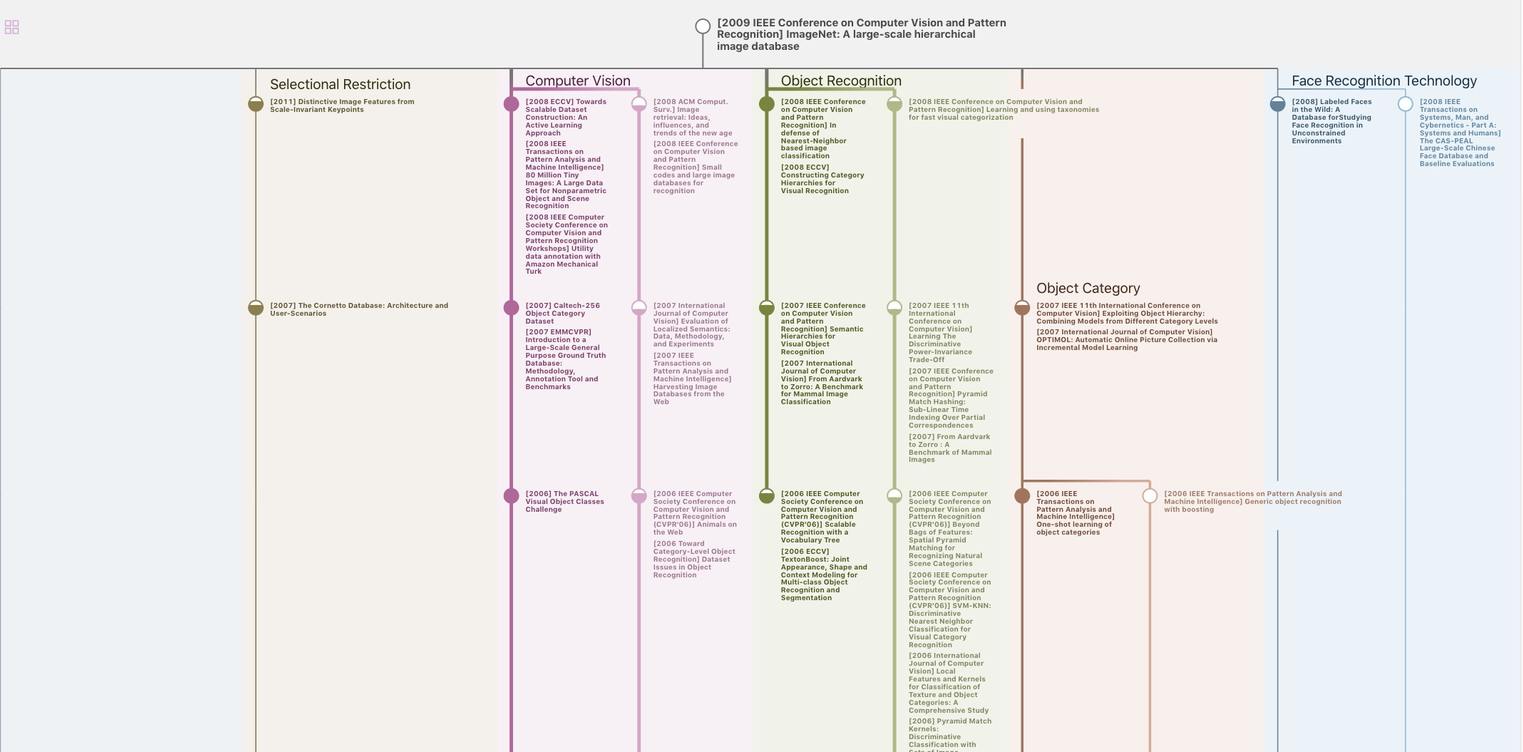
生成溯源树,研究论文发展脉络
Chat Paper
正在生成论文摘要