GITA: Graph to Visual and Textual Integration for Vision-Language Graph Reasoning
CoRR(2024)
摘要
Large Language Models (LLMs) are increasingly used for various tasks with
graph structures. Though LLMs can process graph information in a textual
format, they overlook the rich vision modality, which is an intuitive way for
humans to comprehend structural information and conduct general graph
reasoning. The potential benefits and capabilities of representing graph
structures as visual images (i.e., visual graph) are still
unexplored. To fill the gap, we innovatively propose an end-to-end framework,
called Graph to vIsual and Textual
IntegrAtion (GITA), which firstly incorporates visual graphs into
general graph reasoning. Besides, we establish Graph-based
Vision-Language Question Answering
(GVLQA) dataset from existing graph data, which is the first vision-language
dataset for general graph reasoning purposes. Extensive experiments on the
GVLQA dataset and five real-world datasets show that GITA outperforms
mainstream LLMs in terms of general graph reasoning capabilities. Moreover, We
highlight the effectiveness of the layout augmentation on visual graphs and
pretraining on the GVLQA dataset.
更多查看译文
AI 理解论文
溯源树
样例
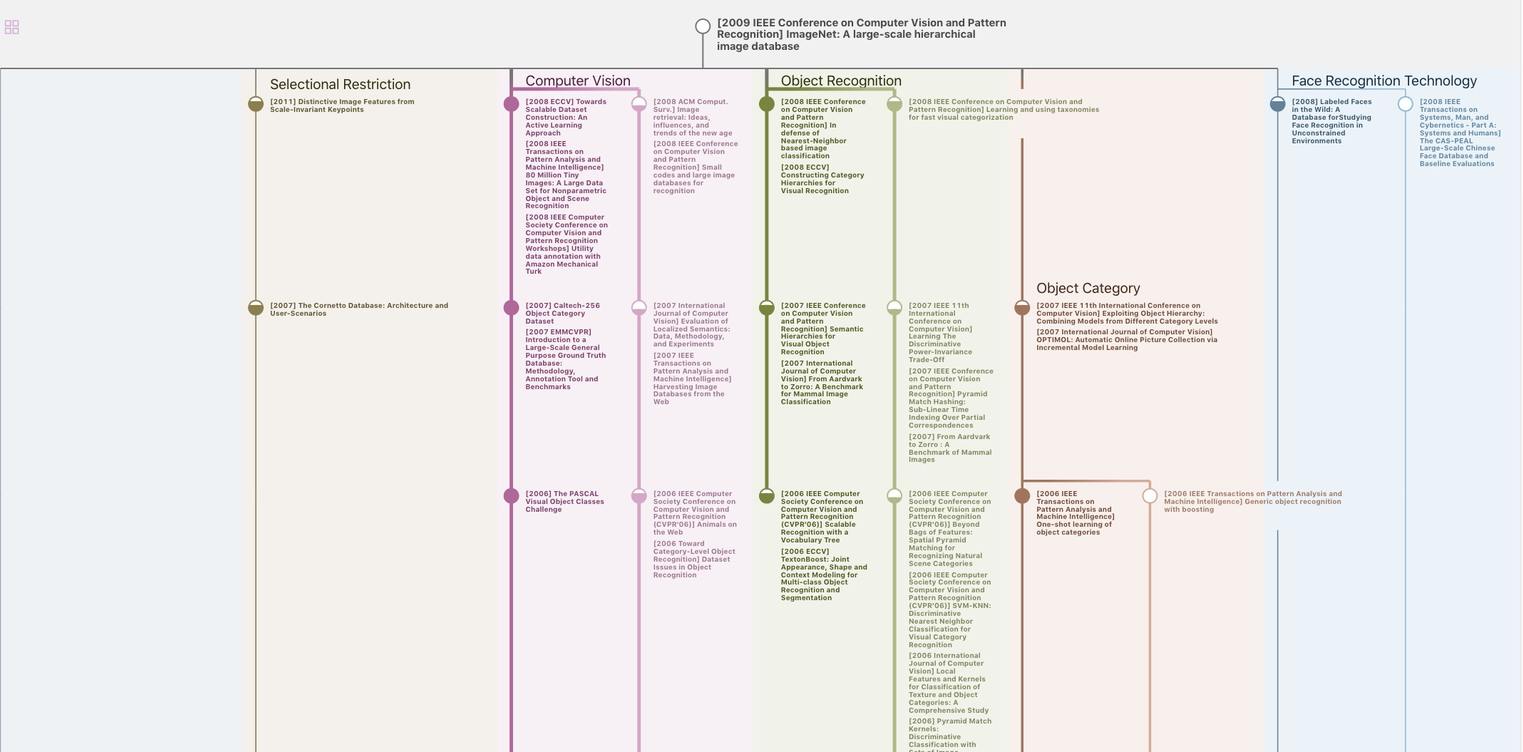
生成溯源树,研究论文发展脉络
Chat Paper
正在生成论文摘要