Predicting Machine Translation Performance on Low-Resource Languages: The Role of Domain Similarity
CoRR(2024)
摘要
Fine-tuning and testing a multilingual large language model is expensive and
challenging for low-resource languages (LRLs). While previous studies have
predicted the performance of natural language processing (NLP) tasks using
machine learning methods, they primarily focus on high-resource languages,
overlooking LRLs and shifts across domains. Focusing on LRLs, we investigate
three factors: the size of the fine-tuning corpus, the domain similarity
between fine-tuning and testing corpora, and the language similarity between
source and target languages. We employ classical regression models to assess
how these factors impact the model's performance. Our results indicate that
domain similarity has the most critical impact on predicting the performance of
Machine Translation models.
更多查看译文
AI 理解论文
溯源树
样例
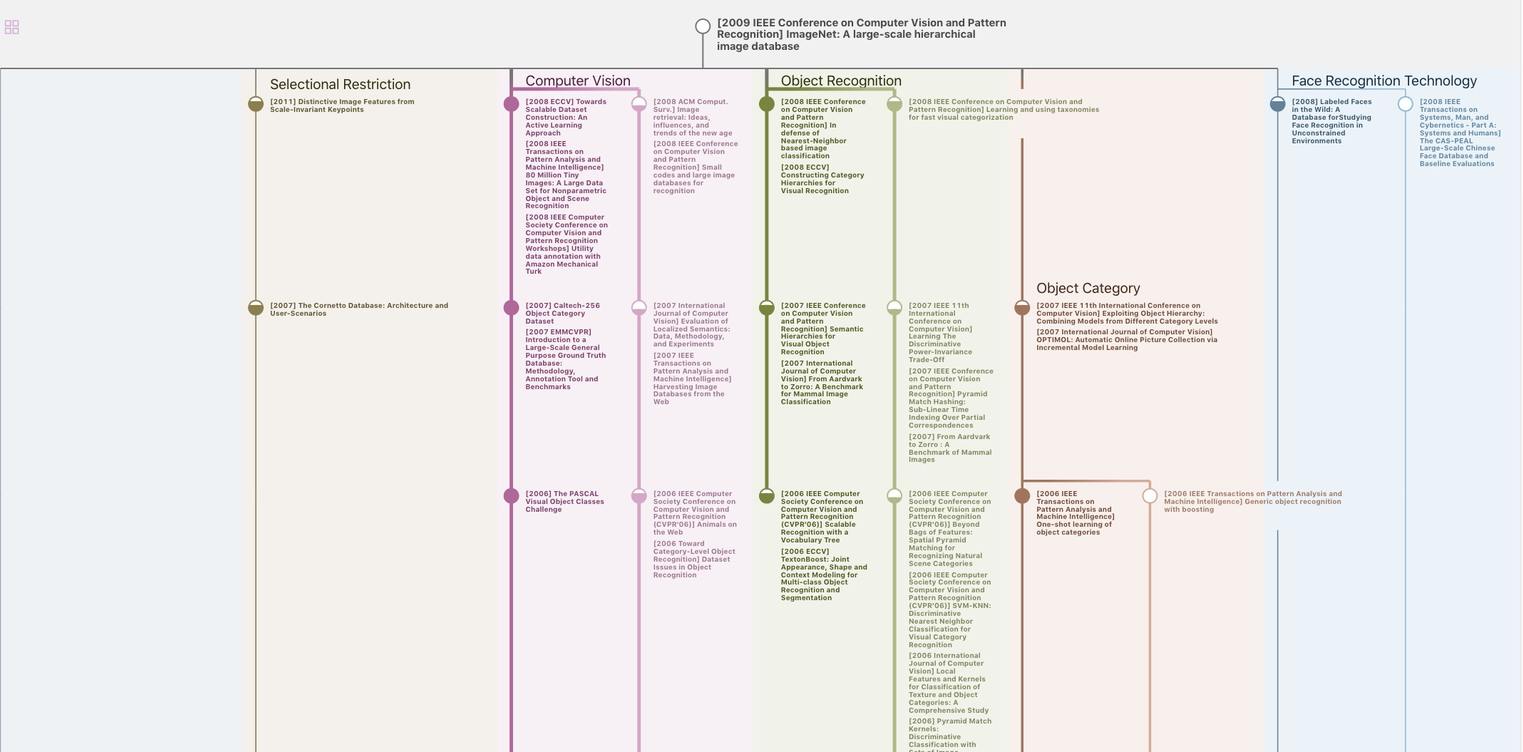
生成溯源树,研究论文发展脉络
Chat Paper
正在生成论文摘要