List-aware Reranking-Truncation Joint Model for Search and Retrieval-augmented Generation
CoRR(2024)
摘要
The results of information retrieval (IR) are usually presented in the form
of a ranked list of candidate documents, such as web search for humans and
retrieval-augmented generation for large language models (LLMs). List-aware
retrieval aims to capture the list-level contextual features to return a better
list, mainly including reranking and truncation. Reranking finely re-scores the
documents in the list. Truncation dynamically determines the cut-off point of
the ranked list to achieve the trade-off between overall relevance and avoiding
misinformation from irrelevant documents. Previous studies treat them as two
separate tasks and model them separately. However, the separation is not
optimal. First, it is hard to share the contextual information of the ranking
list between the two tasks. Second, the separate pipeline usually meets the
error accumulation problem, where the small error from the reranking stage can
largely affect the truncation stage. To solve these problems, we propose a
Reranking-Truncation joint model (GenRT) that can perform the two tasks
concurrently. GenRT integrates reranking and truncation via generative paradigm
based on encoder-decoder architecture. We also design the novel loss functions
for joint optimization to make the model learn both tasks. Sharing parameters
by the joint model is conducive to making full use of the common modeling
information of the two tasks. Besides, the two tasks are performed concurrently
and co-optimized to solve the error accumulation problem between separate
stages. Experiments on public learning-to-rank benchmarks and open-domain Q&A
tasks show that our method achieves SOTA performance on both reranking and
truncation tasks for web search and retrieval-augmented LLMs.
更多查看译文
AI 理解论文
溯源树
样例
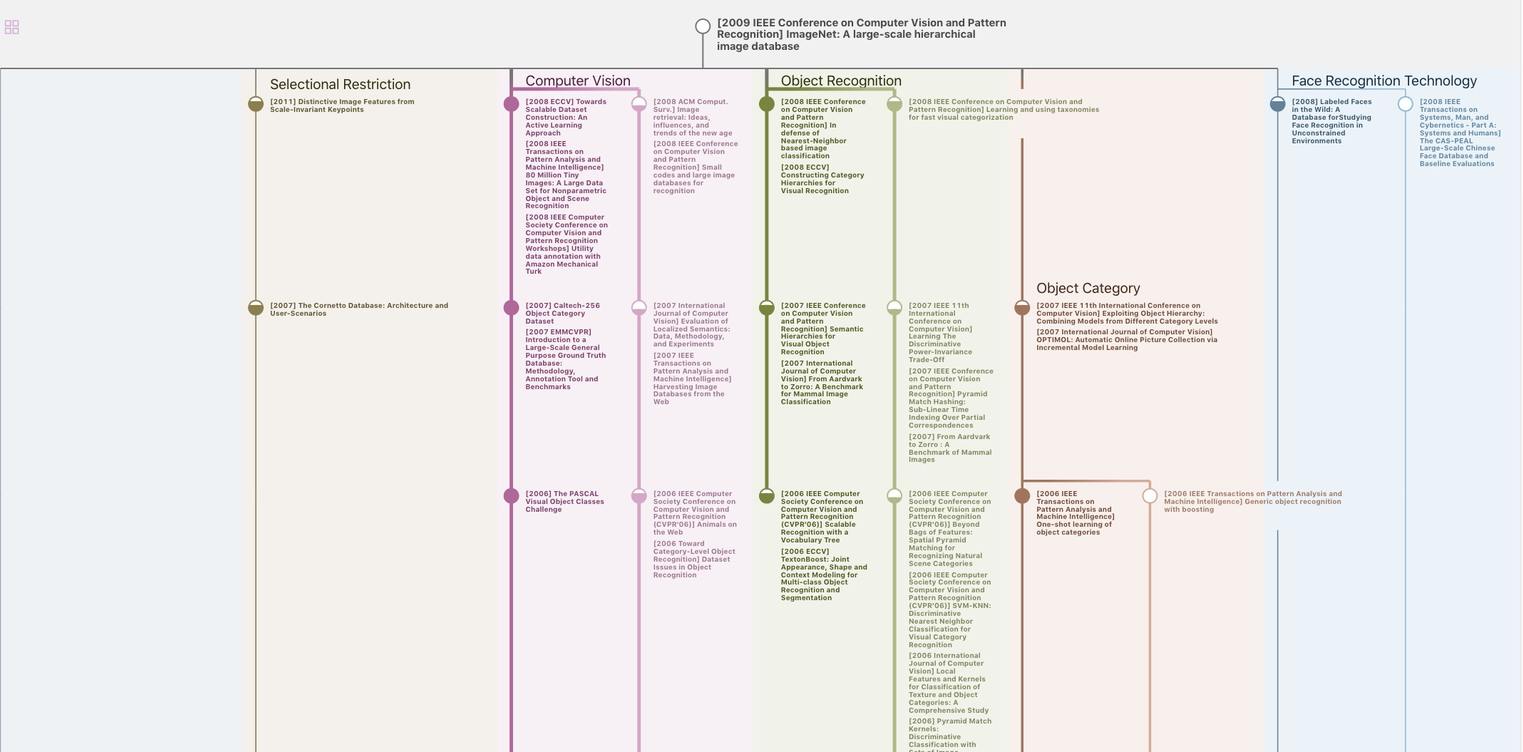
生成溯源树,研究论文发展脉络
Chat Paper
正在生成论文摘要