Taylor Videos for Action Recognition
Computing Research Repository (CoRR)(2024)
Australian National University | Curtin University
Abstract
Effectively extracting motions from video is a critical and long-standingproblem for action recognition. This problem is very challenging becausemotions (i) do not have an explicit form, (ii) have various concepts such asdisplacement, velocity, and acceleration, and (iii) often contain noise causedby unstable pixels. Addressing these challenges, we propose the Taylor video, anew video format that highlights the dominate motions (e.g., a waving hand) ineach of its frames named the Taylor frame. Taylor video is named after Taylorseries, which approximates a function at a given point using important terms.In the scenario of videos, we define an implicit motion-extraction functionwhich aims to extract motions from video temporal block. In this block, usingthe frames, the difference frames, and higher-order difference frames, weperform Taylor expansion to approximate this function at the starting frame. Weshow the summation of the higher-order terms in the Taylor series gives usdominant motion patterns, where static objects, small and unstable motions areremoved. Experimentally we show that Taylor videos are effective inputs topopular architectures including 2D CNNs, 3D CNNs, and transformers. When usedindividually, Taylor videos yield competitive action recognition accuracycompared to RGB videos and optical flow. When fused with RGB or optical flowvideos, further accuracy improvement is achieved.
MoreTranslated text
Key words
Action Recognition
PDF
View via Publisher
AI Read Science
AI Summary
AI Summary is the key point extracted automatically understanding the full text of the paper, including the background, methods, results, conclusions, icons and other key content, so that you can get the outline of the paper at a glance.
Example
Background
Key content
Introduction
Methods
Results
Related work
Fund
Key content
- Pretraining has recently greatly promoted the development of natural language processing (NLP)
- We show that M6 outperforms the baselines in multimodal downstream tasks, and the large M6 with 10 parameters can reach a better performance
- We propose a method called M6 that is able to process information of multiple modalities and perform both single-modal and cross-modal understanding and generation
- The model is scaled to large model with 10 billion parameters with sophisticated deployment, and the 10 -parameter M6-large is the largest pretrained model in Chinese
- Experimental results show that our proposed M6 outperforms the baseline in a number of downstream tasks concerning both single modality and multiple modalities We will continue the pretraining of extremely large models by increasing data to explore the limit of its performance
Try using models to generate summary,it takes about 60s
Must-Reading Tree
Example
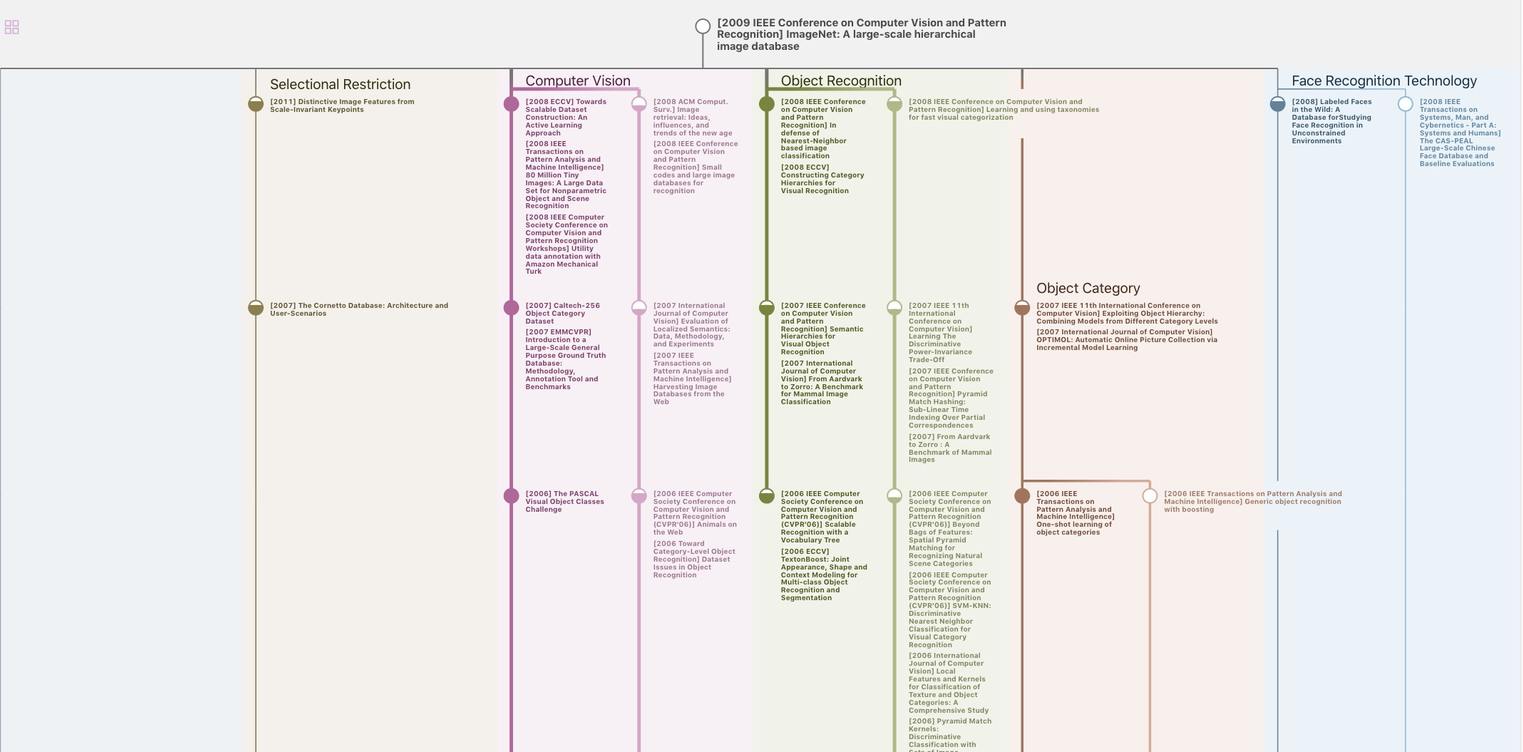
Generate MRT to find the research sequence of this paper
Related Papers
Data Disclaimer
The page data are from open Internet sources, cooperative publishers and automatic analysis results through AI technology. We do not make any commitments and guarantees for the validity, accuracy, correctness, reliability, completeness and timeliness of the page data. If you have any questions, please contact us by email: report@aminer.cn
Chat Paper
去 AI 文献库 对话