UniHENN: Designing More Versatile Homomorphic Encryption-based CNNs without im2col
CoRR(2024)
摘要
Homomorphic encryption enables computations on encrypted data without
decryption, which is crucial for privacy-preserving cloud services. However,
deploying convolutional neural networks (CNNs) with homomorphic encryption
encounters significant challenges, particularly in converting input data into a
two-dimensional matrix for convolution, typically achieved using the im2col
technique. While efficient, this method limits the variety of deployable CNN
models due to compatibility constraints with the encrypted data structure.
UniHENN, a homomorphic encryption-based CNN architecture, eliminates the need
for im2col, ensuring compatibility with a diverse range of CNN models using
homomorphic encryption. Our experiments demonstrate that UniHENN surpasses the
leading 2D CNN inference architecture, PyCrCNN, in inference time, as evidenced
by its performance on the LeNet-1 dataset, where it averages 30.090
seconds–significantly faster than PyCrCNN's 794.064 seconds. Furthermore,
UniHENN outperforms TenSEAL, which employs im2col, in processing concurrent
images, an essential feature for high-demand cloud applications. The
versatility of UniHENN is proven across various CNN architectures, including 1D
and six different 2D CNNs, highlighting its flexibility and efficiency. These
qualities establish UniHENN as a promising solution for privacy-preserving,
cloud-based CNN services, addressing the increasing demand for scalable,
secure, and efficient deep learning in cloud computing environments.
更多查看译文
AI 理解论文
溯源树
样例
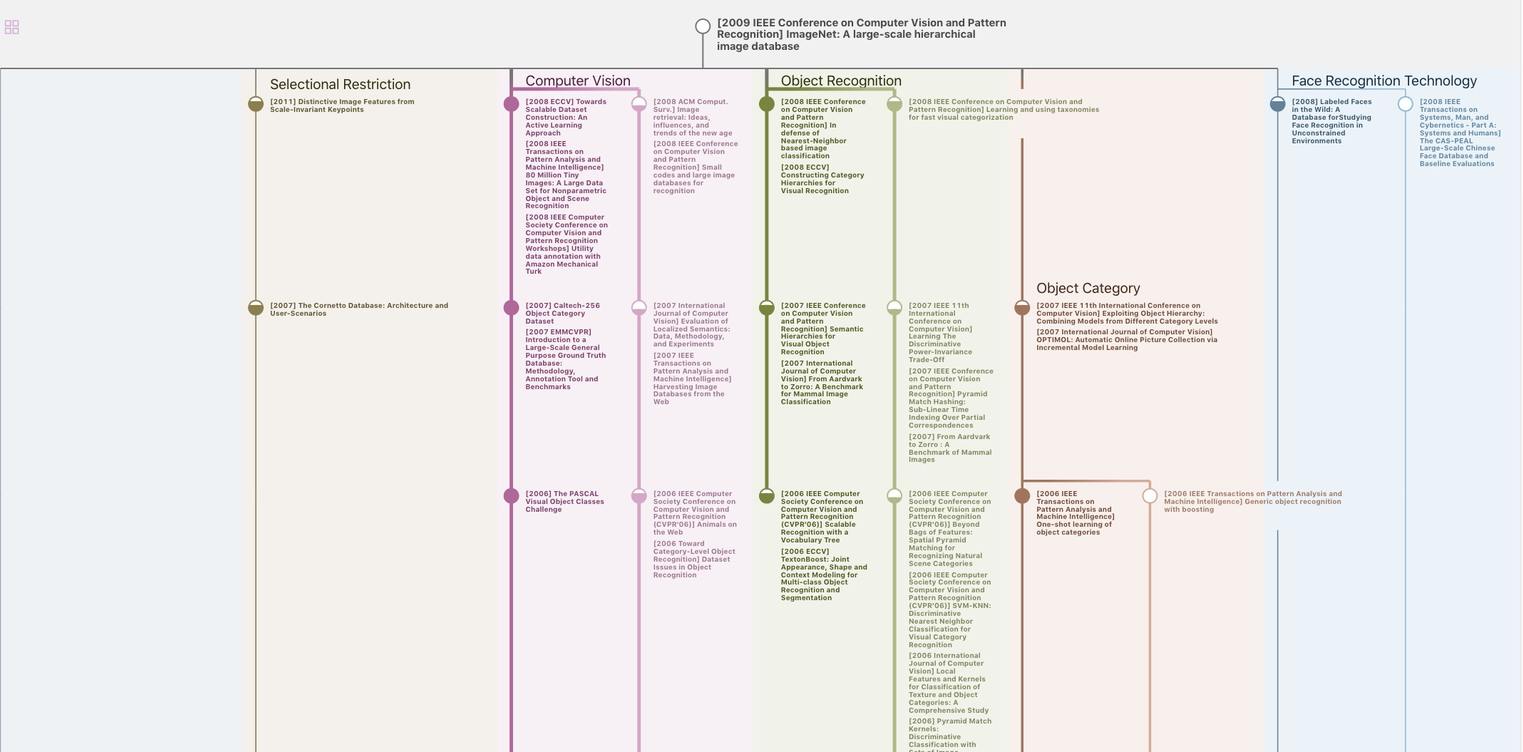
生成溯源树,研究论文发展脉络
Chat Paper
正在生成论文摘要