Enhancing GNN-based Fraud Detector via Semantic Extraction and Max-Representation-Margin.
2023 IEEE International Conference on Data Mining (ICDM)(2023)
摘要
Fraud detection aims to identify fraudsters from normal users. In graph environments, both fraudsters and normal users are modeled as nodes, while edges represent the connections between them. However, fraudulent nodes in the real world often camouflage themselves by establishing numerous fake connections with normal nodes, making them challenging to be identified. Existing fraud detection methods struggle to address this issue, they utilize graph neural networks to aggregate normal informations from normal neighbors, which leads to the smoothing of the fraudulent information. Furthermore, these methods exhibit poor generalization performance as they are unable to detect new fraudsters which not present in the training process. To overcome these limitations, this paper proposes GFAN, a novel model based on Graph Feature enhAncement Network. Specifically, GFAN introduces a specific semantic extraction module to screen and delete fake connections by evaluating the confidence level of edge presence. Additionally, GFAN provides a representation enhanced co-training module that highlights camouflaged fraudulent representations by training the small sphere and large margin support vector data description. Experimental results show that GFAN outperforms other competitive graph-based fraud detectors on public datasets. The GFAN code is available at: https://github.com/scu-kdde/OAM-GFAN-2023.
更多查看译文
关键词
Fraud detection,graph neural networks,heterophily,semantic extraction,SSLM-SVDD
AI 理解论文
溯源树
样例
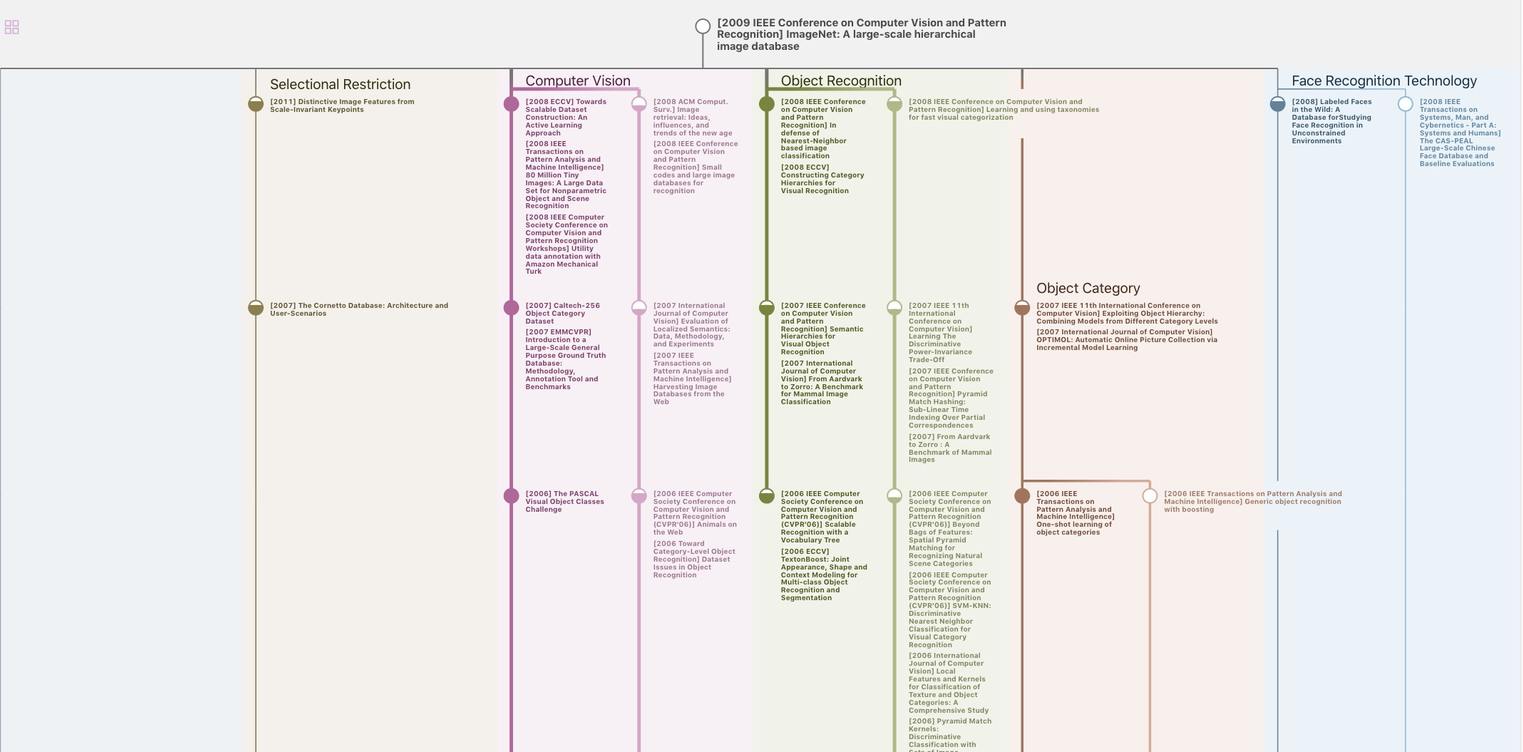
生成溯源树,研究论文发展脉络
Chat Paper
正在生成论文摘要