SimEXT: Self-supervised Representation Learning for Extreme Values in Time Series
23RD IEEE INTERNATIONAL CONFERENCE ON DATA MINING, ICDM 2023(2023)
摘要
Forecasting extreme values in time series is an important but challenging problem as the extreme values are rarely observed even when a large amount of historical data is available. The modeling of extreme values requires a specific focus on estimating the tail distribution of the time series, whose statistical properties may differ from the distribution of its non extreme values. To overcome this challenge, we present a novel self-supervised learning framework, SimEXT, to learn a robust representation of the lime series that preserves the fidelity of its tail distribution. The framework employs a combination of contrastive learning and a reconstruction-based autoencoder architecture to facilitate robust representation learning of the temporal patterns associated with the extreme events. SimEXT also incorporates a wavelet -based data augmentation technique with a distribution-based loss function to prioritize the learning of extreme value distribution. We provide probabilistic guarantees on the wavelet based augmentation that enables the wavelet coefficients to be perturbed during data augmentation without significantly altering the extreme values of the time series. Experimental results on real-world datasets show that SimEXT can effectively learn a robust representation of the time series to boost the performance of downstream tasks for forecasting block maxima values.
更多查看译文
关键词
forecasting,time series,extreme values
AI 理解论文
溯源树
样例
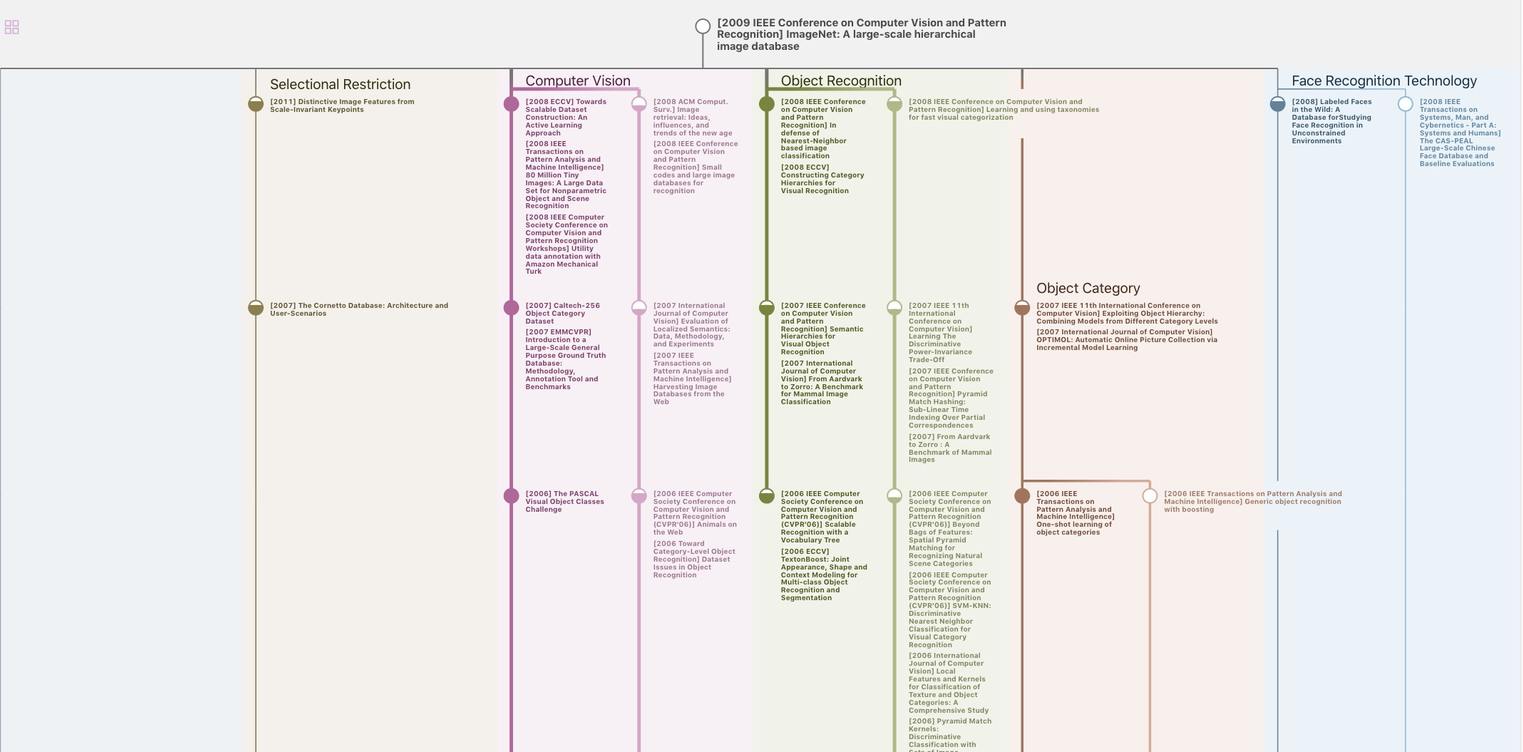
生成溯源树,研究论文发展脉络
Chat Paper
正在生成论文摘要