Graph Collaborative Optimization for Sequential Recommendation
23RD IEEE INTERNATIONAL CONFERENCE ON DATA MINING, ICDM 2023(2023)
摘要
Sequential recommendation is to predict the next. item by capturing the item transformation in the user historical sequences. Although existing methods have achieved convincing results in reconunendation tasks, they ignore the relation between discontinuous items within sequences or those across different sequences. In this paper, we focus on how to fully capture the potential item relation within and across sequences. Specifically, we propose a novel graph collaborative optimization-based method named GOSR, which not only comprehensively explores the real item relation from many perspectives by constructing and optimizing the item relation graph but also collaborates user-item interaction graph to model the interactive behaviors of users and items. Our model converts the loose item sequences to a tight item relation graph and continuously optimize the graph by comprehensively considering the item relation within and across sequences. That is beneficial to dynamically capture the actual item relation. Furthermore, we employ the attention module to extract the long short-term preferences and characters of users and items by collaborating the user-item interaction graph. Finally, we use the relined representations of users and items to predict the next item that a user is most likely to interact with. To the best of our knowledge, this is the first attempt to explore the item relation by constructing and optimizing the item relation graph for better recommendation. Extensive experiments on three popular benchmark datasets demonstrate that GOSR outperforms several state-of-the-art methods.
更多查看译文
关键词
Sequential Recommendation,Item Relation Graph,Graph Neural Network,Graph Optimization.
AI 理解论文
溯源树
样例
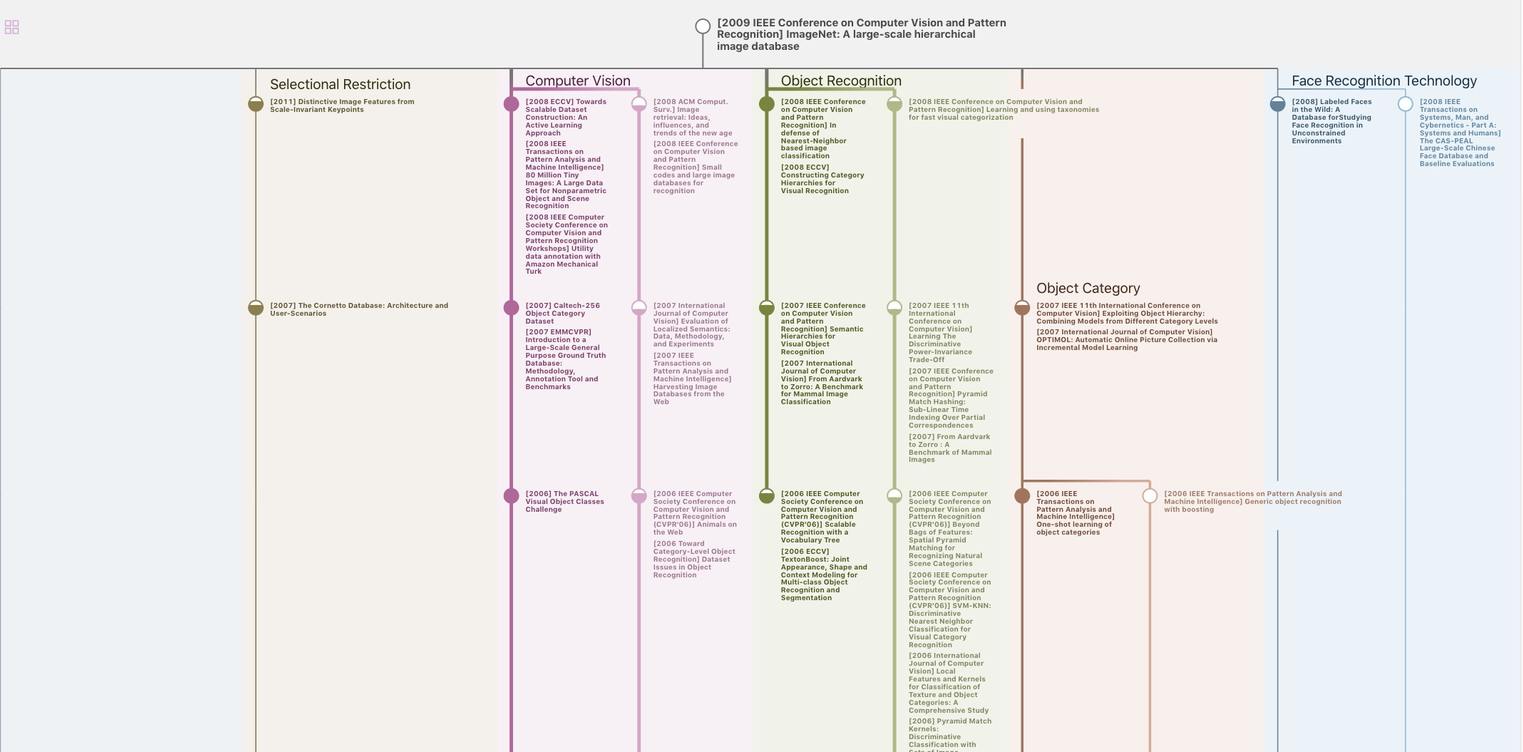
生成溯源树,研究论文发展脉络
Chat Paper
正在生成论文摘要