Graph Open-Set Recognition via Entropy Message Passing
23RD IEEE INTERNATIONAL CONFERENCE ON DATA MINING, ICDM 2023(2023)
摘要
Graph Neural Networks (GNNs) have achieved great success in semi-supervised node classification. These methods usually assume the closed -set setting and classify unlabeled nodes to known classes. However, in reality, there exits some unknown classes due to biased -sampling, distribution shifts, anomaly, etc. Therefore, it is important to identify unknown classes while classifying known classes, which is defined as Graph Open -Set Recognition (GOSR). To alleviate this problem, we propose Entropy Message Passing (EMP) for GOSR, which takes into account the graph structure information when identifying unknown classes and automatically determines the discrimination threshold. To be specific, we calculate the likelihood of a node belonging to unknown class through entropy propagation. Then, we transform the threshold selection into entropy clustering to identify the unknown class nodes. Finally, we classify the remaining nodes. Experimental evaluations on six benchmark graph datasets demonstrate that our method outperforms stateof-the-art baseline methods in unknown class detection and graph open-set recognition tasks. Especially in unknown class detection, our method has achieved a significant reduction in FPR@95 ranging from 12.62% to 55.88%.
更多查看译文
关键词
Graph Neural Networks,Open-Set Recognition,OOD Detection,Node Classification
AI 理解论文
溯源树
样例
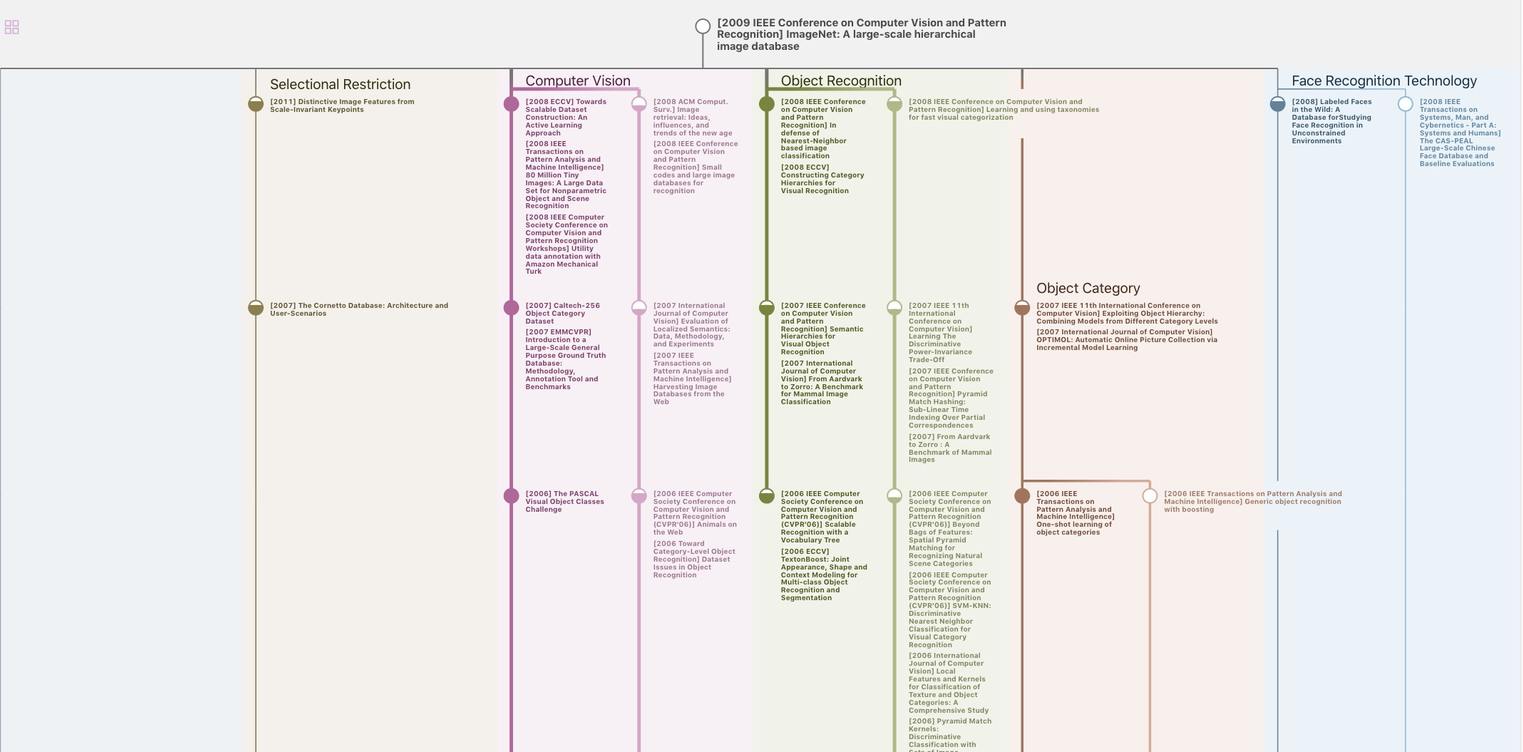
生成溯源树,研究论文发展脉络
Chat Paper
正在生成论文摘要