Can Neural Networks Distinguish High-school Level Mathematical Concepts?
23RD IEEE INTERNATIONAL CONFERENCE ON DATA MINING, ICDM 2023(2023)
摘要
Processing symbolic mathematics algorithmically is an important field of research. It has applications in computer algebra systems and supports researchers as well as applied mathematicians in their daily work. Recently, exploring the ability of neural networks to grasp mathematical concepts has received special attention. One complex task for neural networks is to understand the relation of two mathematical expressions to each other. Despite the advances in learning mathematical relationships, previous studies are limited by small-scale datasets, relatively simple formula construction by few axiomatic rules and even artifacts in the data. With this work, we aim at overcoming these limitations and provide a deeper insight into the representation power of neural networks for classifying mathematical relations. We introduce a novel data generation algorithm to allow for more complex formula compositions and fully include mathematical fields up to high-school level. We research several tree-based and sequential neural architectures for classifying mathematical relations and conduct a systematic analysis of the models against rule-based as well as neural baselines with a focus on varying dataset complexity, generalization abilities, and understanding of syntactical patterns. Our findings show the potential of deep learning models to distinguish high-school level mathematical concepts.
更多查看译文
关键词
learning mathematical relations,mathematical syntax understanding,deep learning
AI 理解论文
溯源树
样例
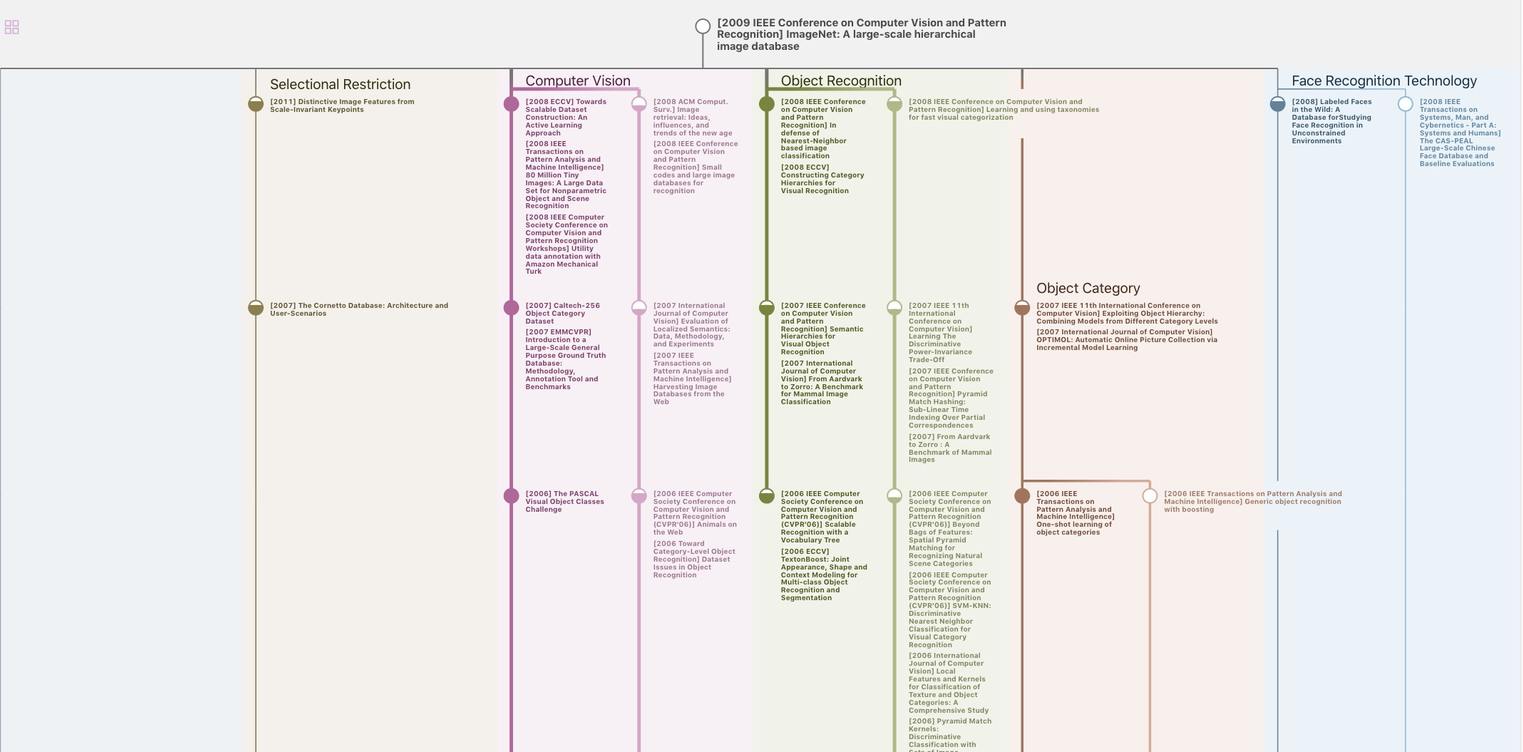
生成溯源树,研究论文发展脉络
Chat Paper
正在生成论文摘要