ATTA: Adversarial Task -transferable Attacks on Autonomous Driving Systems
23RD IEEE INTERNATIONAL CONFERENCE ON DATA MINING, ICDM 2023(2023)
摘要
Deep learning (DL) based perception models have enabled the possibility of current autonomous driving systems (ADS). However, various studies have pointed out that the DL models inside the ADS perception modules are vulnerable to adversarial attacks which can easily manipulate these DL models' predictions. In this paper, we propose a more practical adversarial attack against the ADS perception module. Particularly, instead of targeting one of the Di, models inside the ADS perception module, we propose to use one universal patch to mislead multiple DL models inside the ADS perception module simultaneously which leads to a higher chance of system-wide malfunction. We achieve such a goal by attacking the attention of DL models as a higher level of feature representation rather than traditional gradient-based attacks. We successfully generate a universal patch containing malicious perturbations that can attract multiple victim DL models' attention to further induce their prediction errors. We verify our attack with extensive experiments on a typical ADS perception module structure with live famous datasets and also physical world scenes(1).
更多查看译文
关键词
Deep learning,adversarial attack,autonomous driving system,computer vision.
AI 理解论文
溯源树
样例
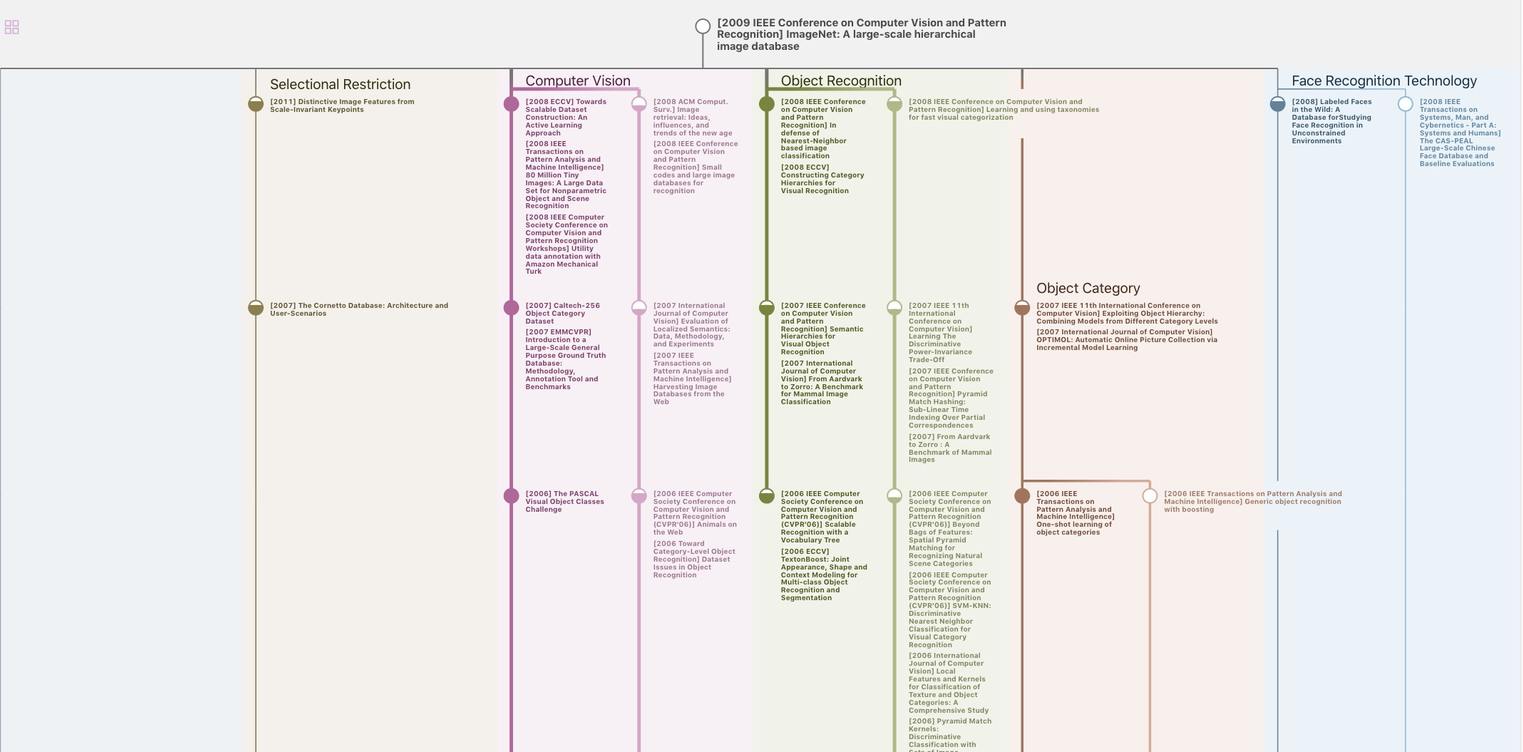
生成溯源树,研究论文发展脉络
Chat Paper
正在生成论文摘要