Take CARE: Improving Inherent Robustness of Spiking Neural Networks with Channel-wise Activation Recalibration Module
23RD IEEE INTERNATIONAL CONFERENCE ON DATA MINING, ICDM 2023(2023)
摘要
Spiking Neural Networks (SNNs) are considered the next generation of deep neural networks for their computation efficiency and biological plausibility. Still, SNN models can be fooled with adversarial perturbations and noises. There is an urgent need for building a robust SNN model that can be deployed in safety-critical domains. Recent works successfully proposed some defense methods inspired by those designed for traditional deep neural network models. However, these methods neglect the inherent robustness of SNN models, which has been proven by previous studies. In this paper, we dedicate ourselves to improving the inherent robustness of SNN without additional training. To do that, we unveil that the success of most attacks relies on obfuscating the model activation. Inspired by this phenomenon, we propose a spiking neural network framework Channel-wise Activation Recalibration (CARE) to improve SNN inherent robustness, which is named CARENet. By analyzing the model activation pattern, we prove that the CARE module has a strong capability of activation preservation. We evaluate our method on three benchmarks. Under diverse attacks, including hybrid attacks using multiple attacks, our method shows significant accuracy gains compared to baselines. Furthermore, our framework achieves competitive performance on natural benchmarks.
更多查看译文
关键词
spiking neural network,object classification,adversarial defense.
AI 理解论文
溯源树
样例
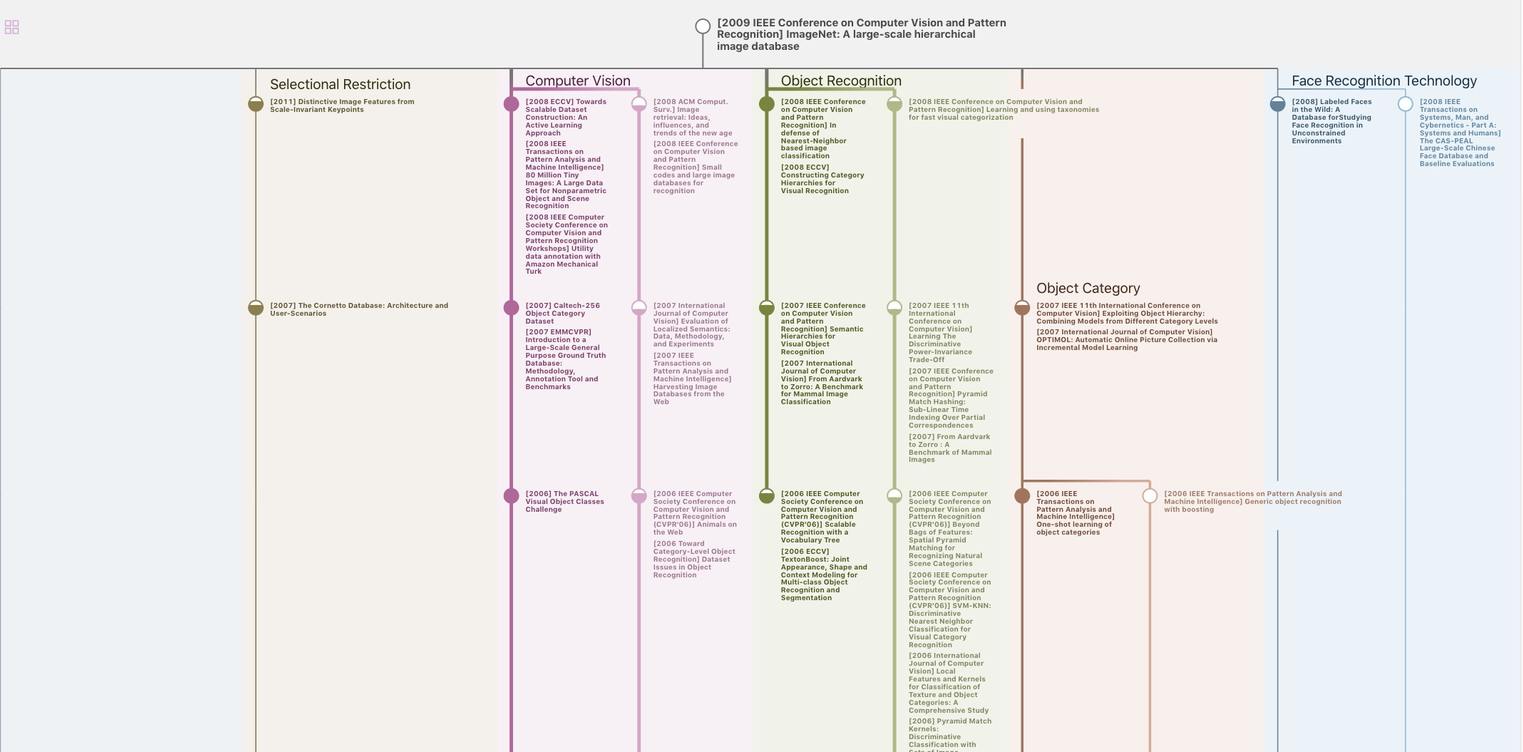
生成溯源树,研究论文发展脉络
Chat Paper
正在生成论文摘要