Fed-mSSA: A Federated Approach for Spatio-Temporal Data Modeling Using Multivariate Singular Spectrum Analysis
23RD IEEE INTERNATIONAL CONFERENCE ON DATA MINING, ICDM 2023(2023)
摘要
In modern cyber-physical systems, the vast inter-connected processes generated from sensor networks necessitate advanced modeling techniques to exploit decentralized data considering edge computation and data access issues. As sensors emit correlated real -life time series, successful forecasting hinges on revealing the spatio-temporal structures and qualities of data. Matrix Estimation -based (ME) methods, as state-of-the-art techniques, excel at denoising and forecasting high-dimensional correlated time series by representing spatio-temporal data as a temporal matrix. However, ME methods face challenges in handling the decentralized data and access restrictions, due to existing licensing agreements and the inherent burden of centralized modeling. To address this limitation, we propose the Federated Multivariate Singular Spectrum Analysis (Fed-mSSA), a federated matrix estimation-based framework, to denoise and predict correlated time series in the presence of noisy and decentralized data. Specifically, we introduce a novel consensus optimization problem to jointly learn the low-rank matrix representation, capturing spatio-temporal patterns to recover latent states and missing data. Furthermore, we present a federated prediction method that privately and efficiently extracts non-linear temporal dynamics using the denoised temporal matrix. Our results show that our proposed framework achieves state-of-the-art prediction performance in a distributed setting, particularly in the presence of missing data.
更多查看译文
关键词
n/a
AI 理解论文
溯源树
样例
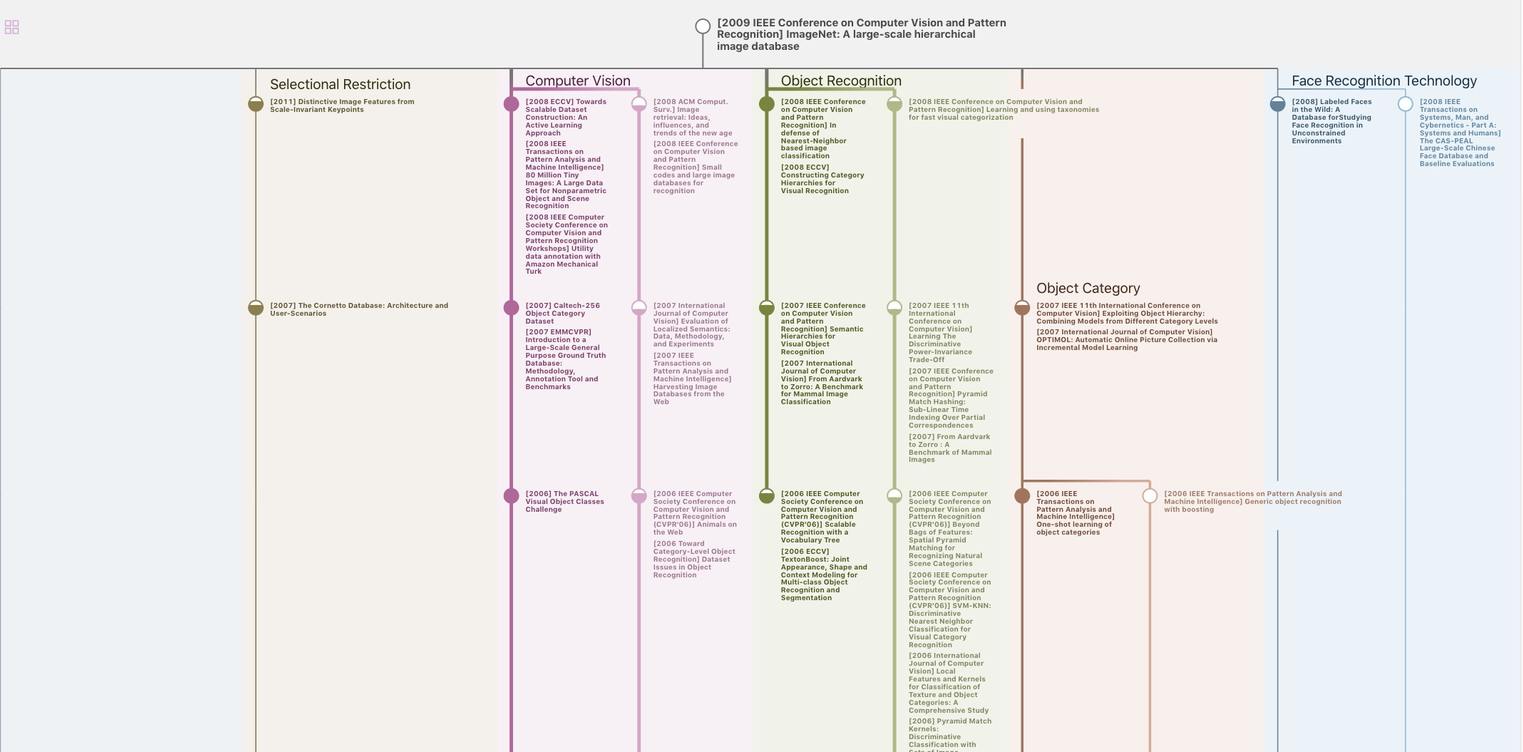
生成溯源树,研究论文发展脉络
Chat Paper
正在生成论文摘要