Named Entity Recognition in the Moroccan Dialect.
2023 7th IEEE Congress on Information Science and Technology (CiSt)(2023)
摘要
Named entity recognition (NER) in modern standard Arabic (MSA) has made great strides by leveraging representations from pretrained language models. However, dialectal Arabic (DA) has not seen the same level of advancement. This work aims to contribute to research efforts in DA by focusing on NER in the Moroccan Dialect (MD) called Darija. Our proposed approach in building NER models for MD consists of finetuning four pretrained language models in different variations of Arabic using data in MD and using their representations as input to classifiers with several neural network architectures. We namely experiment with six different network architectures using bidirectional encoder representations from transformers (BERT) for the input representation layer, bidirectional long short-term memory (BiLSTM) and bidirectional gated recurrent unit (BiGRU) networks for context encoding, and Softmax and conditional random field (CRF) layers for tag decoding. Our models are tested on both data in pure Darija and mixed data in MSA and Darija. Our results show that AraBERT-BiGRU-CRF outperforms the baseline CAMeLBERT-ner-msa on Darija data by 19.70% achieving an F1-score of 68.40%, and the AraBERT-CRF model exceeds the baseline by 4.67% on mixed data with an F1-score of 74.56%. To the best of our knowledge, this is the first work that addresses NER in MD.
更多查看译文
关键词
named entity recognition,Moroccan Dialect,deep learning,BERT,CRF,BiLSTM,BiGRU
AI 理解论文
溯源树
样例
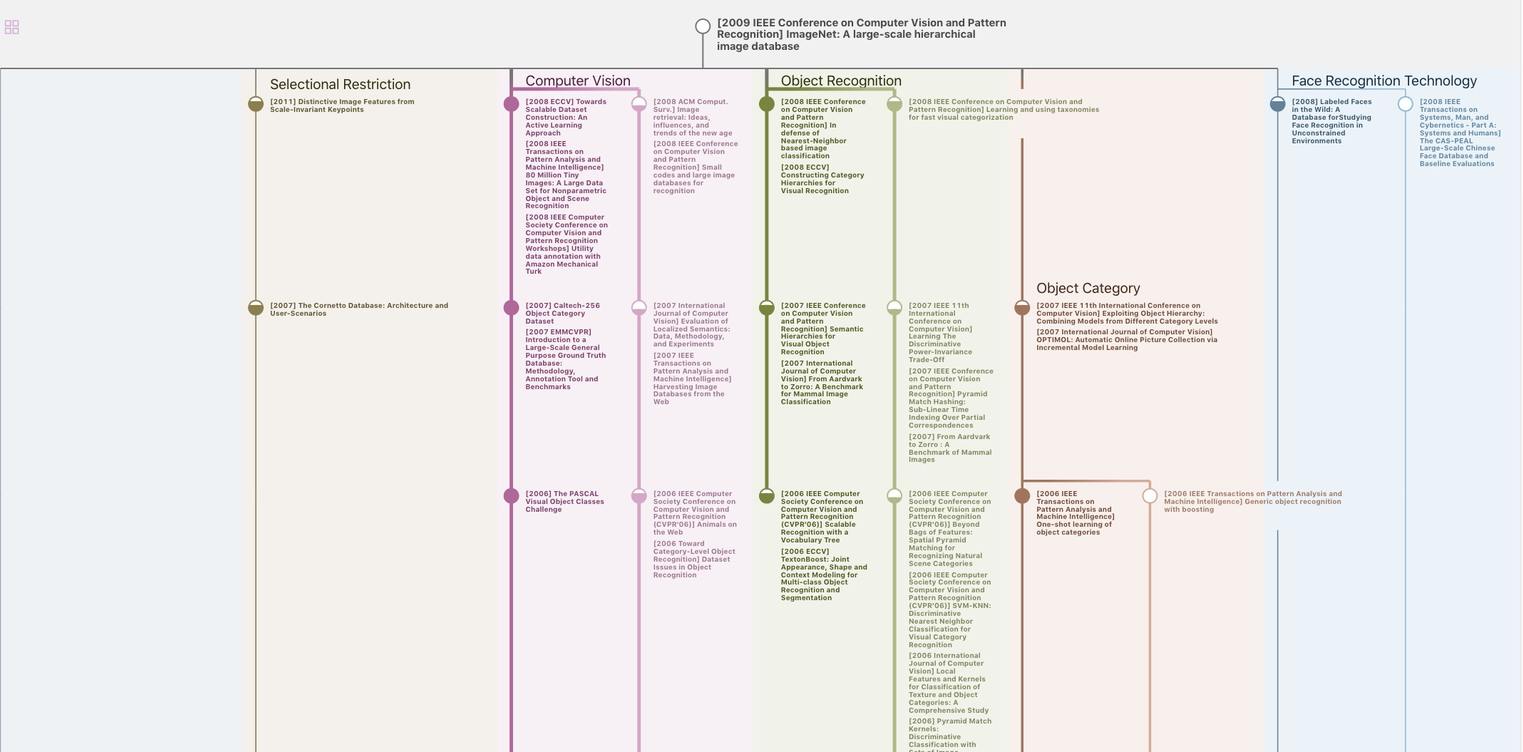
生成溯源树,研究论文发展脉络
Chat Paper
正在生成论文摘要