Multilinear Kernel Regression and Imputation via Manifold Learning
CoRR(2024)
摘要
This paper introduces a novel nonparametric framework for data imputation,
coined multilinear kernel regression and imputation via the manifold assumption
(MultiL-KRIM). Motivated by manifold learning, MultiL-KRIM models data features
as a point cloud located in or close to a user-unknown smooth manifold embedded
in a reproducing kernel Hilbert space. Unlike typical manifold-learning routes,
which seek low-dimensional patterns via regularizers based on graph-Laplacian
matrices, MultiL-KRIM builds instead on the intuitive concept of tangent spaces
to manifolds and incorporates collaboration among point-cloud neighbors
(regressors) directly into the data-modeling term of the loss function.
Multiple kernel functions are allowed to offer robustness and rich
approximation properties, while multiple matrix factors offer low-rank
modeling, integrate dimensionality reduction, and streamline computations with
no need of training data. Two important application domains showcase the
functionality of MultiL-KRIM: time-varying-graph-signal (TVGS) recovery, and
reconstruction of highly accelerated dynamic-magnetic-resonance-imaging (dMRI)
data. Extensive numerical tests on real and synthetic data demonstrate
MultiL-KRIM's remarkable speedups over its predecessors, and outperformance
over prevalent "shallow" data-imputation techniques, with a more intuitive and
explainable pipeline than deep-image-prior methods.
更多查看译文
关键词
Imputation,regression,kernel,manifold,graph,MRI
AI 理解论文
溯源树
样例
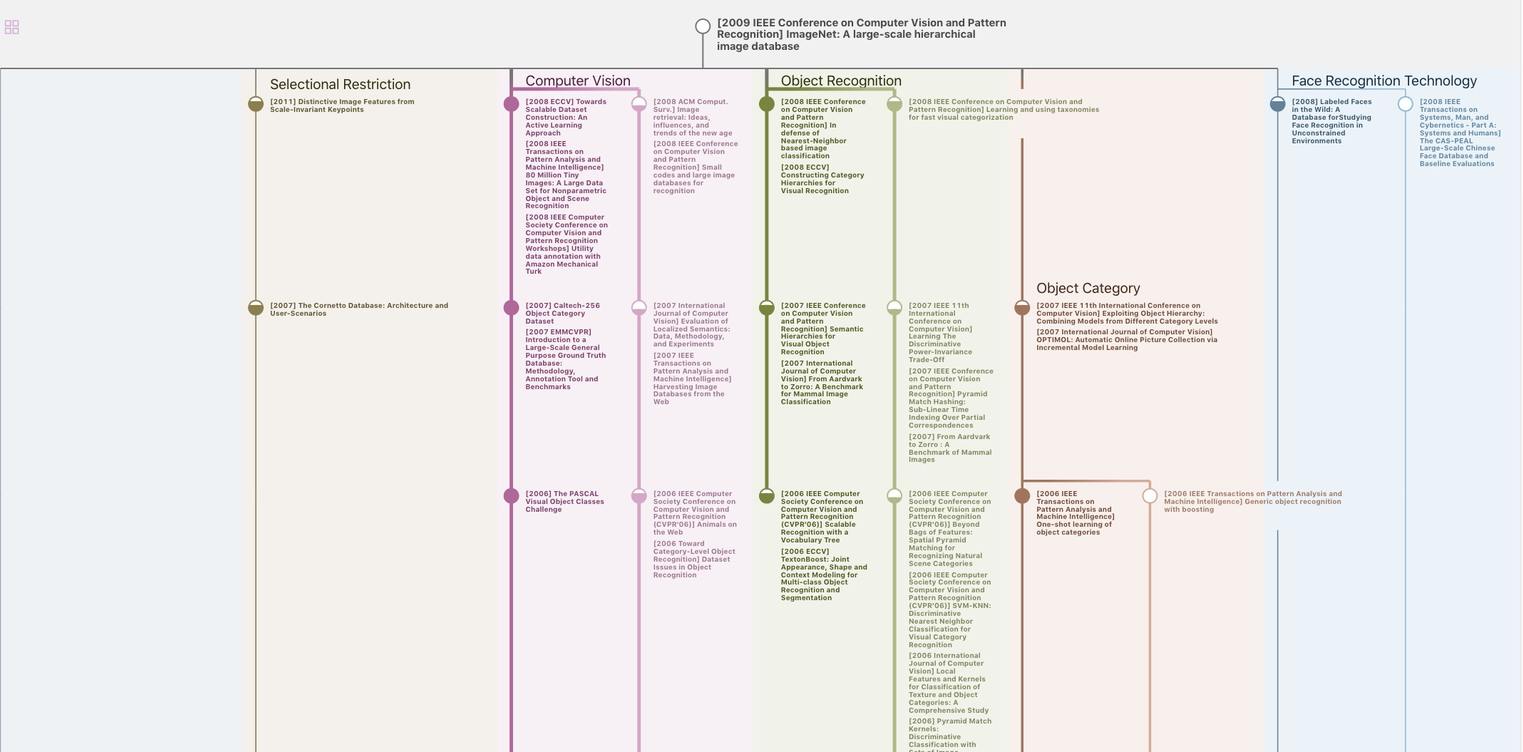
生成溯源树,研究论文发展脉络
Chat Paper
正在生成论文摘要