Transductive Reward Inference on Graph
CoRR(2024)
摘要
In this study, we present a transductive inference approach on that reward
information propagation graph, which enables the effective estimation of
rewards for unlabelled data in offline reinforcement learning. Reward inference
is the key to learning effective policies in practical scenarios, while direct
environmental interactions are either too costly or unethical and the reward
functions are rarely accessible, such as in healthcare and robotics. Our
research focuses on developing a reward inference method based on the
contextual properties of information propagation on graphs that capitalizes on
a constrained number of human reward annotations to infer rewards for
unlabelled data. We leverage both the available data and limited reward
annotations to construct a reward propagation graph, wherein the edge weights
incorporate various influential factors pertaining to the rewards.
Subsequently, we employ the constructed graph for transductive reward
inference, thereby estimating rewards for unlabelled data. Furthermore, we
establish the existence of a fixed point during several iterations of the
transductive inference process and demonstrate its at least convergence to a
local optimum. Empirical evaluations on locomotion and robotic manipulation
tasks validate the effectiveness of our approach. The application of our
inferred rewards improves the performance in offline reinforcement learning
tasks.
更多查看译文
AI 理解论文
溯源树
样例
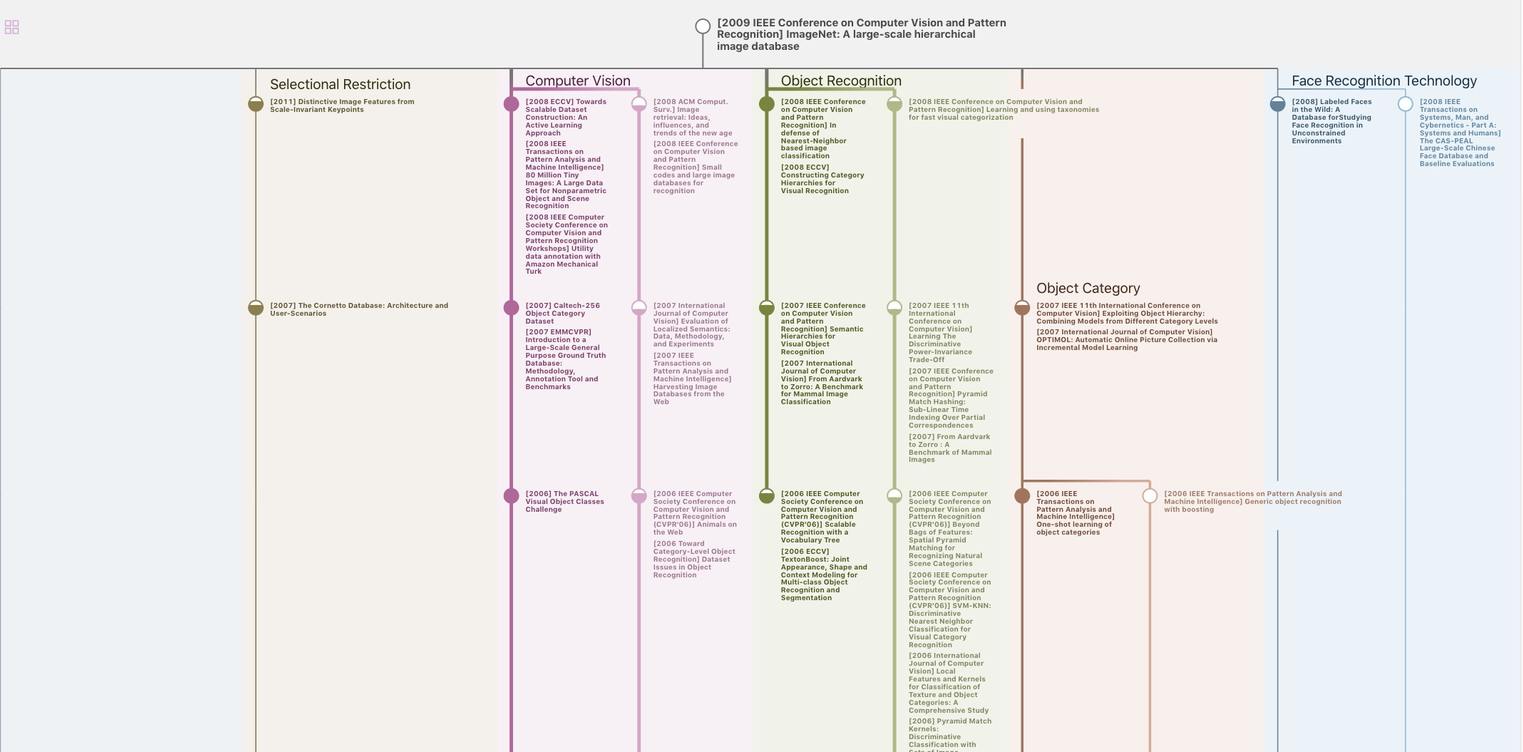
生成溯源树,研究论文发展脉络
Chat Paper
正在生成论文摘要