Rethinking Skill Extraction in the Job Market Domain using Large Language Models
CoRR(2024)
摘要
Skill Extraction involves identifying skills and qualifications mentioned in
documents such as job postings and resumes. The task is commonly tackled by
training supervised models using a sequence labeling approach with BIO tags.
However, the reliance on manually annotated data limits the generalizability of
such approaches. Moreover, the common BIO setting limits the ability of the
models to capture complex skill patterns and handle ambiguous mentions. In this
paper, we explore the use of in-context learning to overcome these challenges,
on a benchmark of 6 uniformized skill extraction datasets. Our approach
leverages the few-shot learning capabilities of large language models (LLMs) to
identify and extract skills from sentences. We show that LLMs, despite not
being on par with traditional supervised models in terms of performance, can
better handle syntactically complex skill mentions in skill extraction tasks.
更多查看译文
AI 理解论文
溯源树
样例
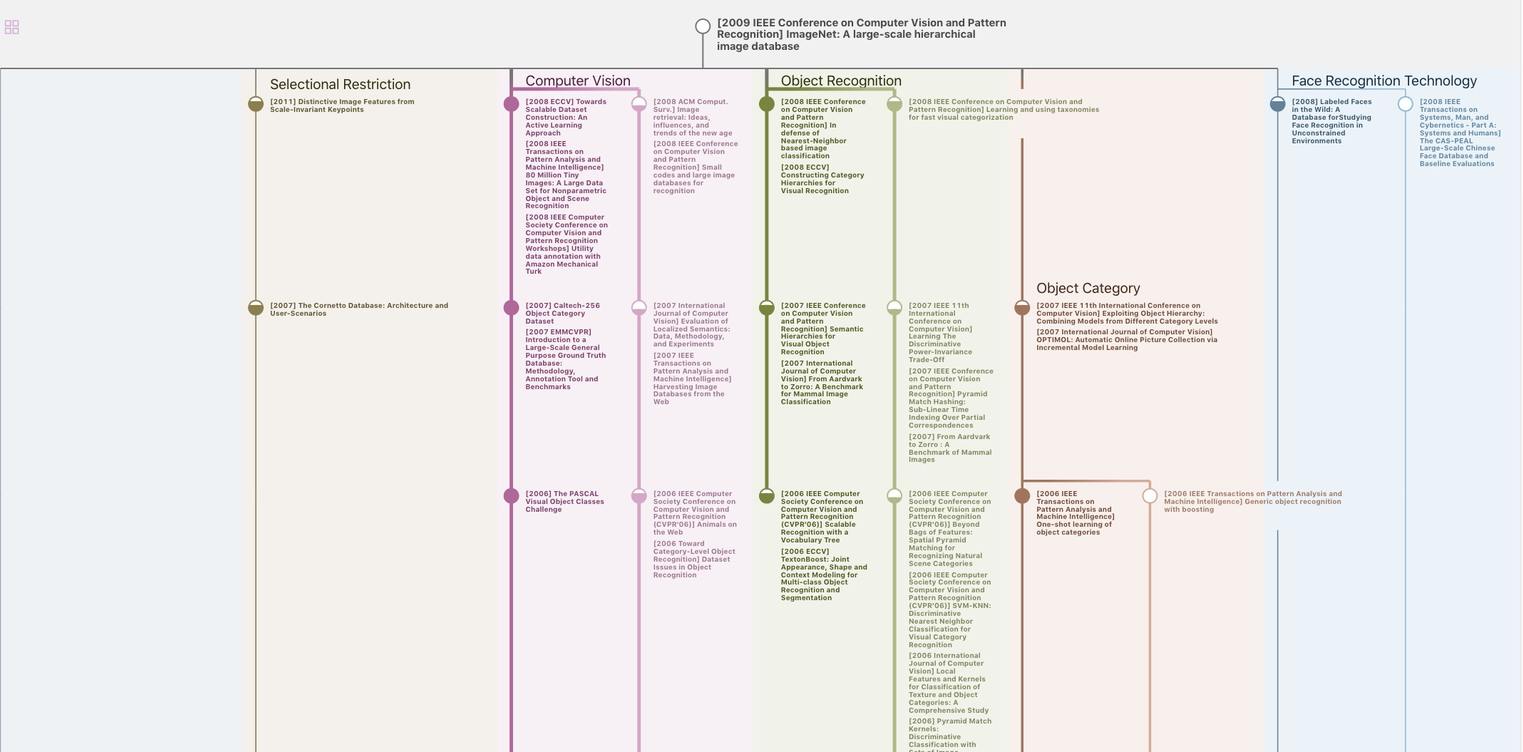
生成溯源树,研究论文发展脉络
Chat Paper
正在生成论文摘要