On Gauge Freedom, Conservativity and Intrinsic Dimensionality Estimation in Diffusion Models
ICLR(2024)
摘要
Diffusion models are generative models that have recently demonstratedimpressive performances in terms of sampling quality and density estimation inhigh dimensions. They rely on a forward continuous diffusion process and abackward continuous denoising process, which can be described by atime-dependent vector field and is used as a generative model. In the originalformulation of the diffusion model, this vector field is assumed to be thescore function (i.e. it is the gradient of the log-probability at a given timein the diffusion process). Curiously, on the practical side, most studies ondiffusion models implement this vector field as a neural network function anddo not constrain it be the gradient of some energy function (that is, moststudies do not constrain the vector field to be conservative). Even though somestudies investigated empirically whether such a constraint will lead to aperformance gain, they lead to contradicting results and failed to provideanalytical results. Here, we provide three analytical results regarding theextent of the modeling freedom of this vector field. {Firstly, we propose anovel decomposition of vector fields into a conservative component and anorthogonal component which satisfies a given (gauge) freedom. Secondly, fromthis orthogonal decomposition, we show that exact density estimation and exactsampling is achieved when the conservative component is exactly equals to thetrue score and therefore conservativity is neither necessary nor sufficient toobtain exact density estimation and exact sampling. Finally, we show that whenit comes to inferring local information of the data manifold, constraining thevector field to be conservative is desirable.
更多查看译文
关键词
gauge freedom,conservativitym intrinsic dimensionality estimation,diffusion models,explainable AI,theory
AI 理解论文
溯源树
样例
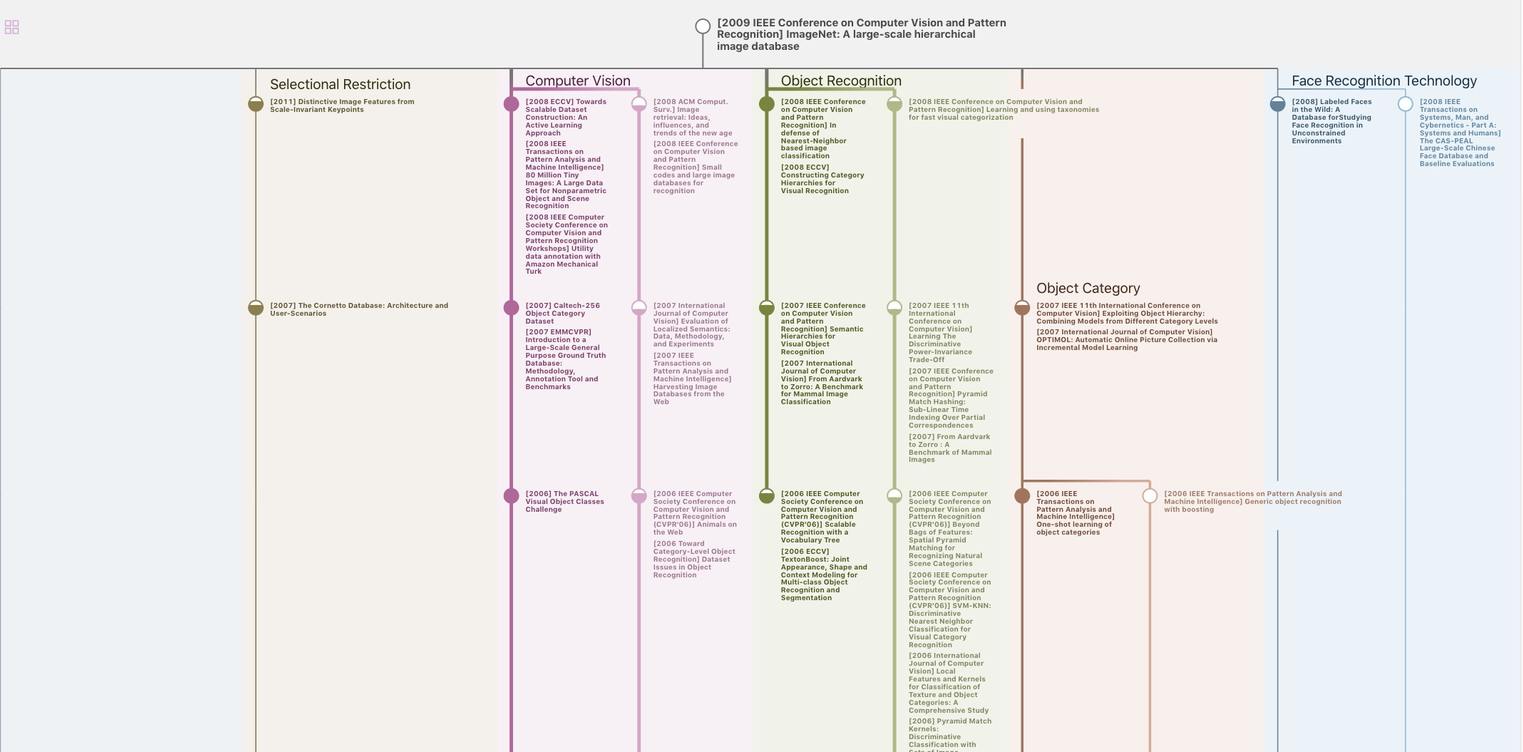
生成溯源树,研究论文发展脉络
Chat Paper
正在生成论文摘要