An early screening model for preeclampsia: utilizing zero-cost maternal predictors exclusively
Hypertension Research(2024)
摘要
To provide a reliable, low-cost screening model for preeclampsia, this study developed an early screening model in a retrospective cohort (25,709 pregnancies) and validated in a validation cohort (1760 pregnancies). A data augmentation method (α-inverse weighted-GMM + RUS) was applied to a retrospective cohort before 10 machine learning models were simultaneously trained on augmented data, and the optimal model was chosen via sensitivity (at a false positive rate of 10%). The AdaBoost model, utilizing 16 predictors, was chosen as the final model, achieving a performance beyond acceptable with Area Under the Receiver Operating Characteristic Curve of 0.8008 and sensitivity of 0.5190. All predictors were derived from clinical characteristics, some of which were previously unreported (such as nausea and vomiting in pregnancy and menstrual cycle irregularity). Compared to previous studies, our model demonstrated superior performance, exhibiting at least a 50% improvement in sensitivity over checklist-based approaches, and a minimum of 28% increase over multivariable models that solely utilized maternal predictors. We validated an effective approach for preeclampsia early screening incorporating zero-cost predictors, which demonstrates superior performance in comparison to similar studies. We believe the application of the approach in combination with high performance approaches could substantially increase screening participation rate among pregnancies. Machine learning model for early preeclampsia screening, using 16 zero-cost predictors derived from clinical characteristics, was built on a 10-year Chinese cohort. The model outperforms similar research by at least 28%; validated on an independent cohort.
更多查看译文
关键词
Preeclampsia,Early screening,Machine learning,Zero-cost predictors,Data augmentation
AI 理解论文
溯源树
样例
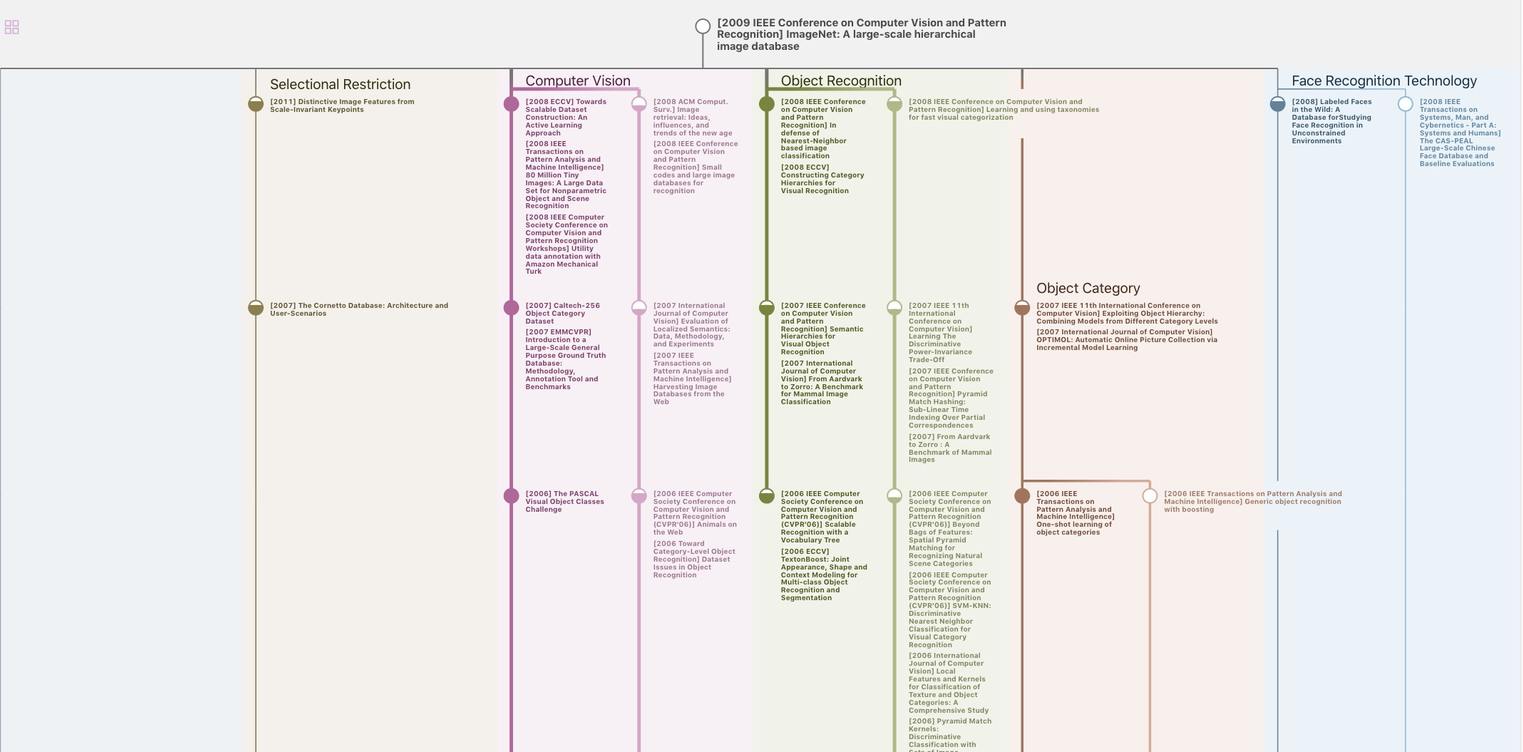
生成溯源树,研究论文发展脉络
Chat Paper
正在生成论文摘要