Toward an end-to-end implicit addressee modeling for dialogue disentanglement
Multimedia Tools and Applications(2024)
摘要
Multi-party conversations are a practical and challenging scenario with more than two sessions entangled with each other. Therefore, it is necessary to disentangle a whole conversation into several sessions to help listeners decide which session each utterance is part of to respond to it appropriately. This task is referred to as dialogue disentanglement. Most existing methods focus on message-pair modeling and clustering in two-step methods, which are sensitive to the noise classification pairs and result in poor clustering performance. To address this challenge, we propose a contrastive learning framework named IAM for end-to-end implicit addressee modeling. To be more specific, IAM makes utterances in different sessions mutually exclusive to identify the sessions of utterances better. Then a clustering method is adopted to generate predicted clustering labels. Moreover, to alleviate the lack of massive annotated data, we introduce a strategy to select pseudo samples for unsupervised training without manual annotations. Comprehensive experiments conducted on the Movie Dialogue and IRC datasets demonstrate that IAM achieves state-of-the-art in both supervised and unsupervised manners.
更多查看译文
关键词
Dialogue disentanglement,Contrastive learning,Clustering
AI 理解论文
溯源树
样例
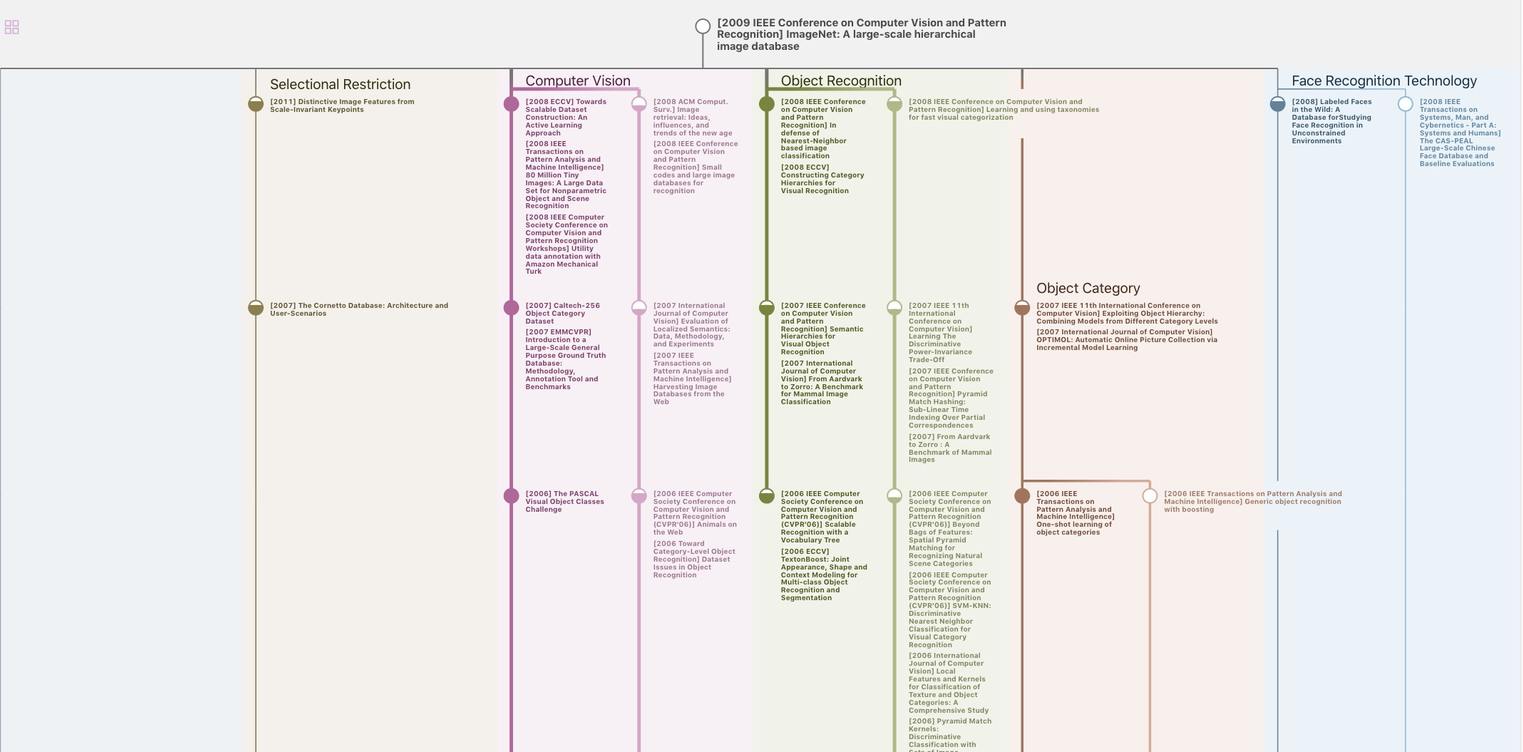
生成溯源树,研究论文发展脉络
Chat Paper
正在生成论文摘要