Pixel Reduction of High-Resolution Image Using Principal Component Analysis
Journal of the Indian Society of Remote Sensing(2024)
摘要
A high-definition picture needs more storage space and occupies the memory. For a model to run efficiently, we need to provide a good-quality image, but as we know, we should load a lot of data to obtain accurate results. While it is often applied for compressing or reducing the dimensionality of high-resolution images, it is not specifically designed for pixel reduction. However, we can use principal component analysis (PCA) to achieve pixel reduction by treating the image as a matrix and applying PCA on its pixel values. It is important to note that while PCA can reduce the dimensionality of an image, it does not necessarily reduce the storage size unless the image has a high number of pixels compared to the number of components retained. Experimental results indicate that principal component analysis (PCA) proves effective in addressing these challenges by efficiently reducing the dimensionality of image data while retaining the principal properties of the original image. This reduction in dimensionality not only mitigates data transmission issues but also results in more efficient storage utilization. Additionally, reducing the number of pixels through PCA may result in some loss of detail and image quality. For this reason, we choose PCA, an efficient algorithm for reducing high-dimensional data. In machine learning, a picture with so many pixels will be considered high-dimensional data. The principal component analysis is a decomposition algorithm. It is a fundamental decomposition algorithm which reduces the dimensions in a dataset. Discovering the new variables, referred to as principal components, serves to streamline the solution to the eigenvalue/eigenvectors problem. PCA can be characterized as an adaptive data analysis technology since these variables are crafted to adjust to diverse data types and structures. Comparative analysis proves that the proposed method is more efficient. The study converts the image's pixel dimension from the original data, attaining a pixel rate of 3,155,200-to-100-pixel rate. Therefore, converting a 9,465,600-byte image to a 300-byte image, pixel is usually 3 bytes. Further research will be investigating an alternative dimension reduction approach for solving nonlinear problem space with correlated variables.
更多查看译文
关键词
Image compression,Principal component analysis,Dimensionality reduction,Open CV (Open-Source Computer Vision Library),Background masking
AI 理解论文
溯源树
样例
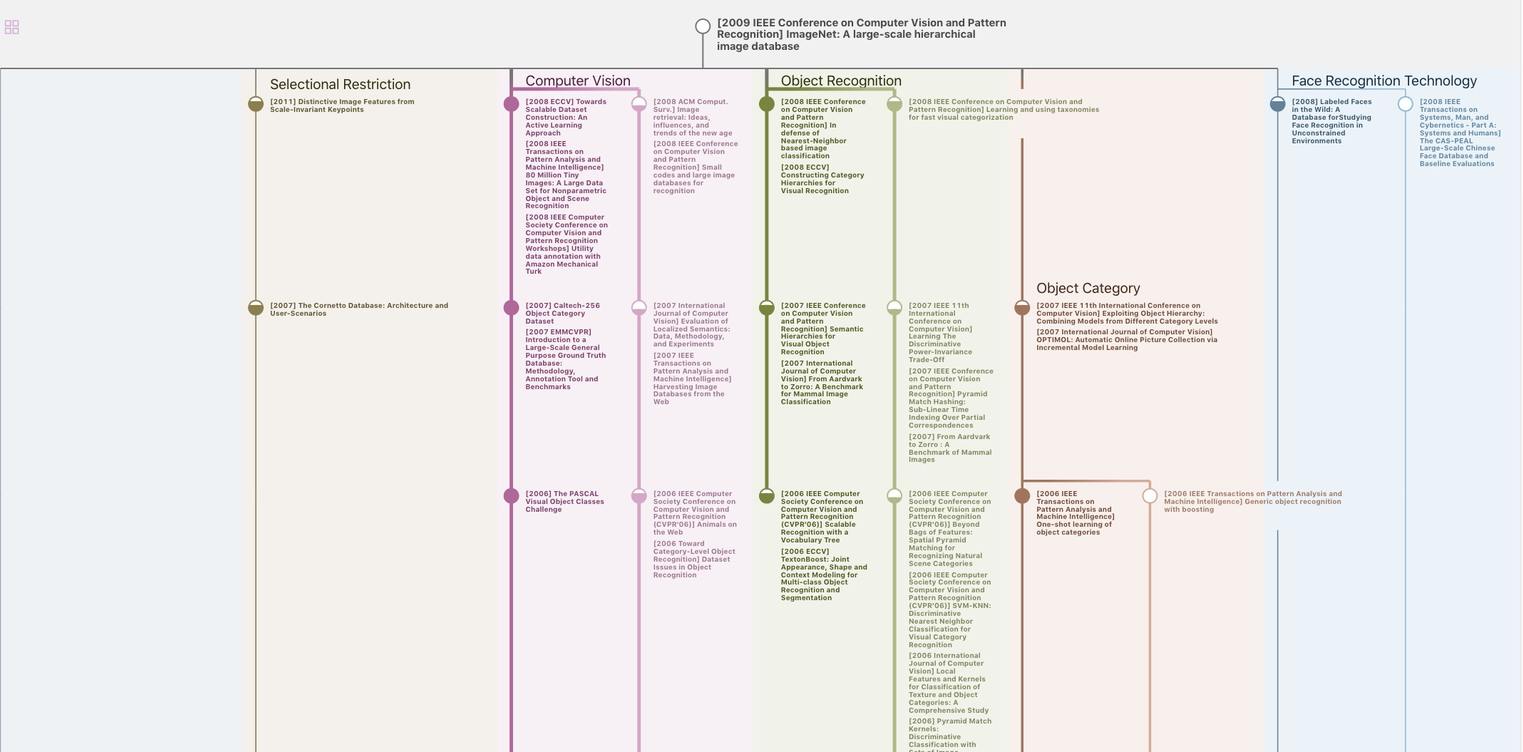
生成溯源树,研究论文发展脉络
Chat Paper
正在生成论文摘要