Debugging Malware Classification Models Based on Event Logs with Explainable AI
2023 23RD IEEE INTERNATIONAL CONFERENCE ON DATA MINING WORKSHOPS, ICDMW 2023(2023)
摘要
As machine learning models find broader applications in cybersecurity, the importance of model explainability becomes more evident. In the area of malware detection, where the consequence of misclassification can be severe, explainability becomes crucial. AI explainers not only help understand the reasons behind malware classifications but also assist in finetuning models to improve detection accuracy. Additionally, AI explainers can serve as a valuable tool for error detection, ensuring accountability, and mitigating potential biases. In this paper, we demonstrate how AI explainers can play a vital role in identifying issues in data collection and enhancing our comprehension of the model's classification results. Our analysis of explanation results reveals several issues within the data collection process, including event loss and the presence of environment-specific information. Additionally, we have identified mislabelled samples based on the explanation results and shared lessons learned from our data collection efforts.
更多查看译文
关键词
explainable AI,malware detection,SHAP,TreeSHAP,ETW,XAI,Random Forest
AI 理解论文
溯源树
样例
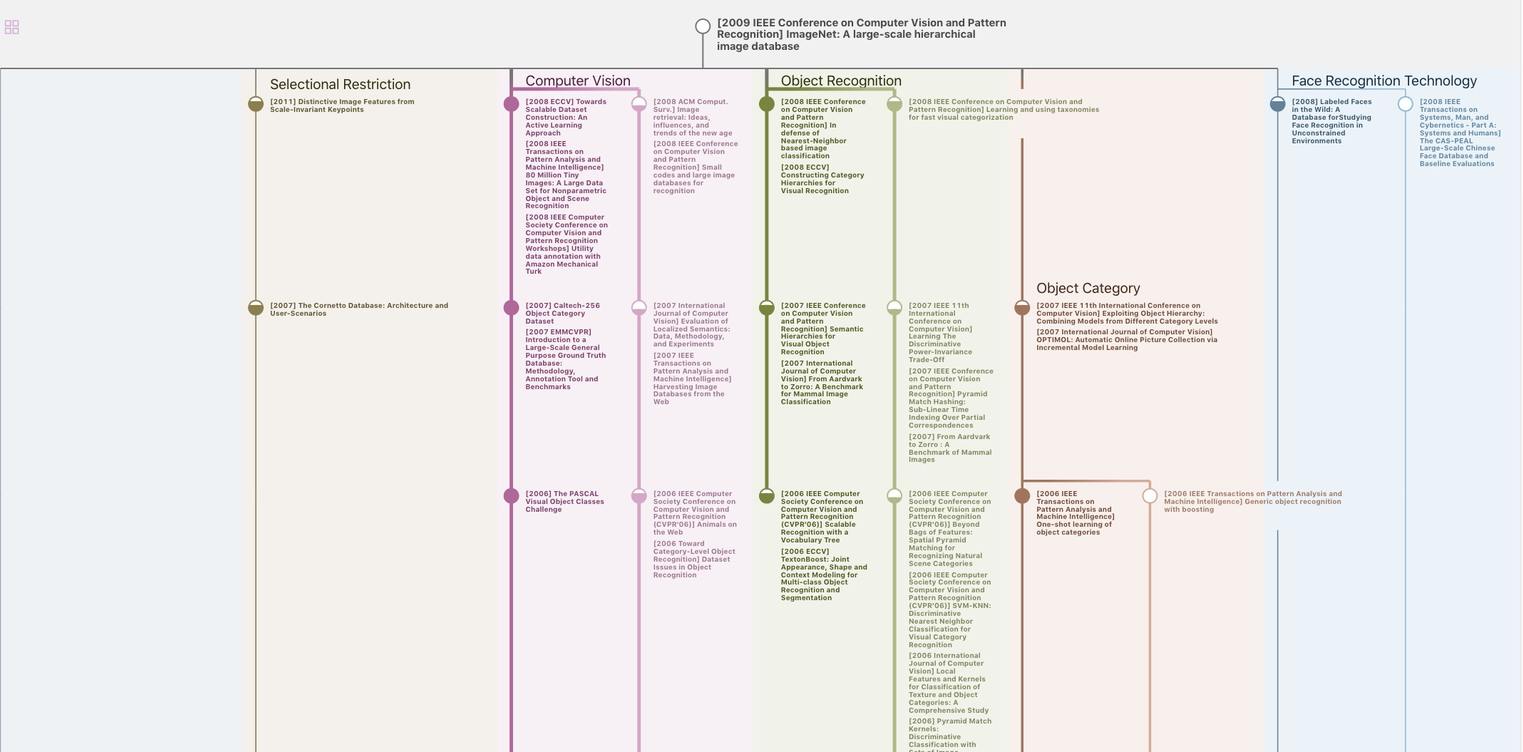
生成溯源树,研究论文发展脉络
Chat Paper
正在生成论文摘要