The Issue of Baselines in Explainability Methods
2023 23RD IEEE INTERNATIONAL CONFERENCE ON DATA MINING WORKSHOPS, ICDMW 2023(2023)
摘要
As the complexity of neural network architectures continues to rise, the need for interpretable machine learning models becomes increasingly crucial. Many interpretability methods leverage baselines to interpret predictions and generate insightful explanations. However, the selection of baselines introduces complexities that can significantly influence the accuracy and reliability of explanations. In this paper, we delve into the critical role of baselines in interpretability methods, focusing on two prominent techniques: SHAP and Integrated Gradients. Through an in-depth analysis, we uncover the limitations of conventional baselines, including potential biases and their inability to capture some feature importances in tabular datasets. To address these challenges, we propose a novel embedding layer that incorporates the idea of the baseline through learning a particular neutral reference point. Through experiments on popular tabular datasets, including Adult, Compas, German, and Heloc, we demonstrate an improvement in computing attribution values while offering valuable insights into model explainability.
更多查看译文
关键词
interpretability,explainability,feature importance,embedding layer
AI 理解论文
溯源树
样例
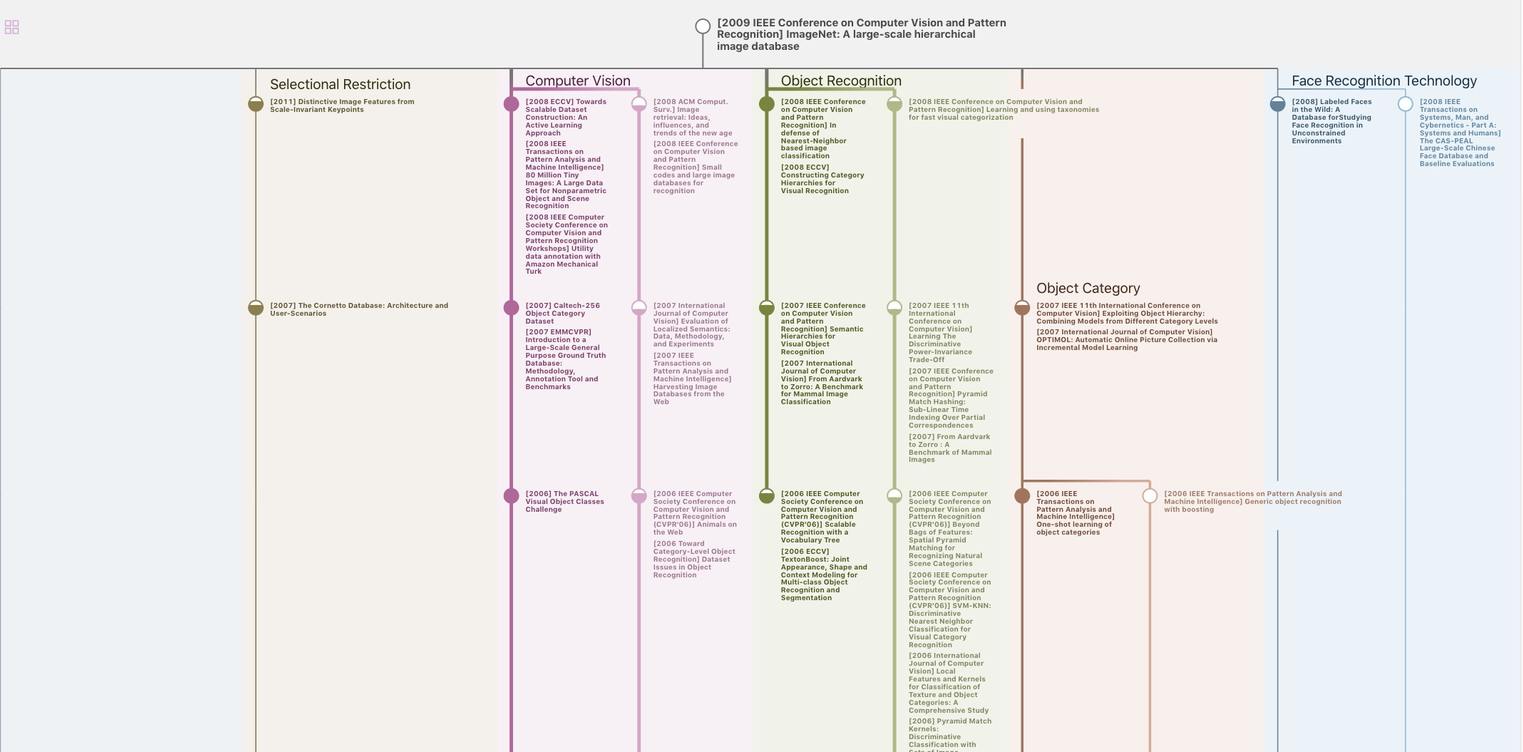
生成溯源树,研究论文发展脉络
Chat Paper
正在生成论文摘要