Multilevel Contrastive Graph Masked Autoencoders for Unsupervised Graph-Structure Learning.
IEEE transactions on neural networks and learning systems(2024)
摘要
Unsupervised graph-structure learning (GSL) which aims to learn an effective graph structure applied to arbitrary downstream tasks by data itself without any labels' guidance, has recently received increasing attention in various real applications. Although several existing unsupervised GSL has achieved superior performance in different graph analytical tasks, how to utilize the popular graph masked autoencoder to sufficiently acquire effective supervision information from the data itself for improving the effectiveness of learned graph structure has been not effectively explored so far. To tackle the above issue, we present a multilevel contrastive graph masked autoencoder (MCGMAE) for unsupervised GSL. Specifically, we first introduce a graph masked autoencoder with the dual feature masking strategy to reconstruct the same input graph-structured data under the original structure generated by the data itself and learned graph-structure scenarios, respectively. And then, the inter-and intra-class contrastive loss is introduced to maximize the mutual information in feature and graph-structure reconstruction levels simultaneously. More importantly, the above inter-and intra-class contrastive loss is also applied to the graph encoder module for further strengthening their agreement at the feature-encoder level. In comparison to the existing unsupervised GSL, our proposed MCGMAE can effectively improve the training robustness of the unsupervised GSL via different-level supervision information from the data itself. Extensive experiments on three graph analytical tasks and eight datasets validate the effectiveness of the proposed MCGMAE.
更多查看译文
关键词
Graph neural networks (GNNs),node classification,node clustering,unsupervised graph-structure learning (GSL)
AI 理解论文
溯源树
样例
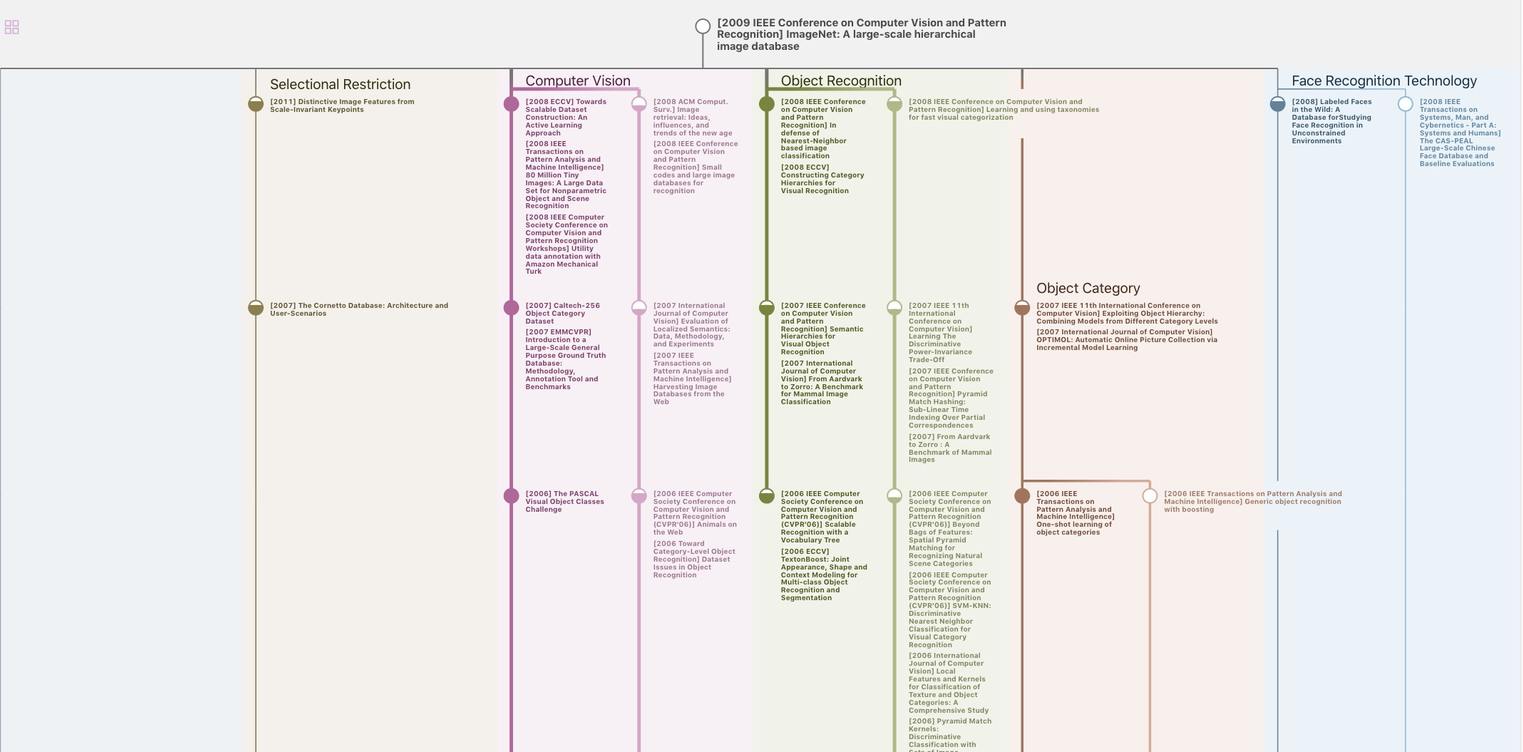
生成溯源树,研究论文发展脉络
Chat Paper
正在生成论文摘要