Pathway Evolution Through a Bottlenecking-Debottlenecking Strategy and Machine Learning-Aided Flux Balancing
Advanced science (Weinheim, Baden-Wurttemberg, Germany)(2024)
摘要
The evolution of pathway enzymes enhances the biosynthesis of high-value chemicals, crucial for pharmaceutical, and agrochemical applications. However, unpredictable evolutionary landscapes of pathway genes often hinder successful evolution. Here, the presence of complex epistasis is identifued within the representative naringenin biosynthetic pathway enzymes, hampering straightforward directed evolution. Subsequently, a biofoundry-assisted strategy is developed for pathway bottlenecking and debottlenecking, enabling the parallel evolution of all pathway enzymes along a predictable evolutionary trajectory in six weeks. This study then utilizes a machine learning model, ProEnsemble, to further balance the pathway by optimizing the transcription of individual genes. The broad applicability of this strategy is demonstrated by constructing an Escherichia coli chassis with evolved and balanced pathway genes, resulting in 3.65 g L-1 naringenin. The optimized naringenin chassis also demonstrates enhanced production of other flavonoids. This approach can be readily adapted for any given number of enzymes in the specific metabolic pathway, paving the way for automated chassis construction in contemporary biofoundries. A biofoundry-assisted strategy for pathway bottlenecking and debottlenecking enables the parallel evolution of all pathway enzymes along a predictable evolutionary trajectory. A machine learning model can further relax the epistasis of the evolved pathway by optimizing the corresponding promoter combinations. Above strategy contributes to an Escherichia coli chassis with evolved and balanced pathway genes. image
更多查看译文
关键词
biofoundry,directed evolution,machine learning,pathway debottlenecking
AI 理解论文
溯源树
样例
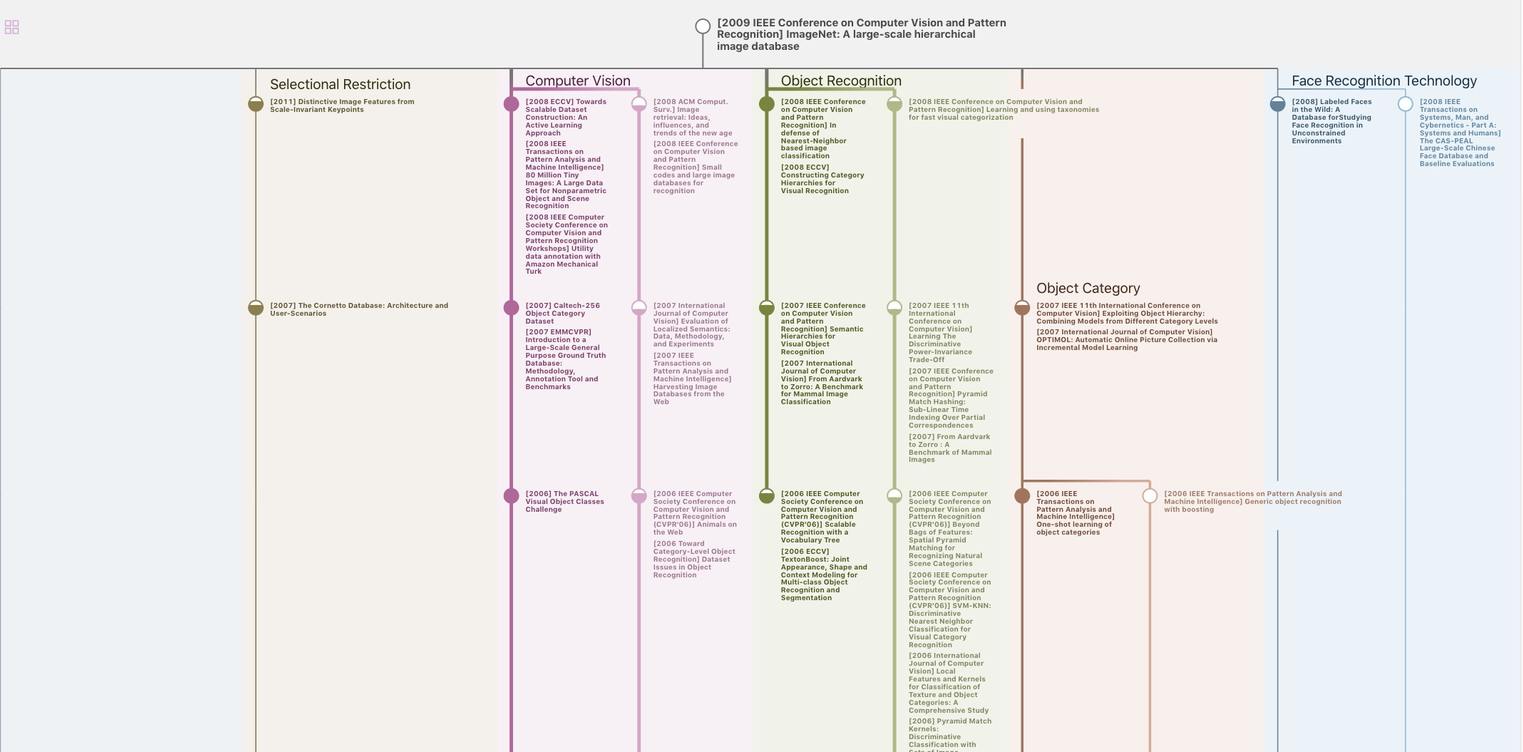
生成溯源树,研究论文发展脉络
Chat Paper
正在生成论文摘要