A Few-shot Learning Approach for Multi-Task CT Image Classification
2023 14th International Conference on Electrical and Electronics Engineering (ELECO)(2023)
摘要
Few-Shot Learning (FSL) demonstrates significant promise in addressing the limitations of traditional deep learning, especially those pertaining to data scarcity. This specific limitation is a common issue in the medical field, especially in the case of new diseases. In this study, we employed an FSL approach by training it on a custom medical dataset comprised of Computed Tomography (CT) images encompassing diverse medical tasks. To showcase the efficacy of FSL in the medical domain, we utilized the ProtoNet both with and without LASTShot. Furthermore, we conducted training using the ISIC 2018 dataset to evaluate their performance on a different type of medical imagery. For the CT multi-task dataset, the ProtoNet method delivered promising outcomes, achieving accuracy rates of 80%, 96%, and 92% in 1-shot, 5-shot, and 10-shot scenarios, respectively. Additionally, our results on the ISIC dataset underscored the significance of a broader range of tasks for robust FSL performance.
更多查看译文
关键词
Image Classification,Few-shot Learning,Deep Learning,Medical Field,Medical Datasets,Traditional Deep Learning,Loss Function,Target Class,Representative Class,Challenging Dataset,Support Set,Strong Classifier,Query Point,Presence Of Organs,Segmentation Challenge,Meta Learning,Few-shot Classification,Prototypical Network
AI 理解论文
溯源树
样例
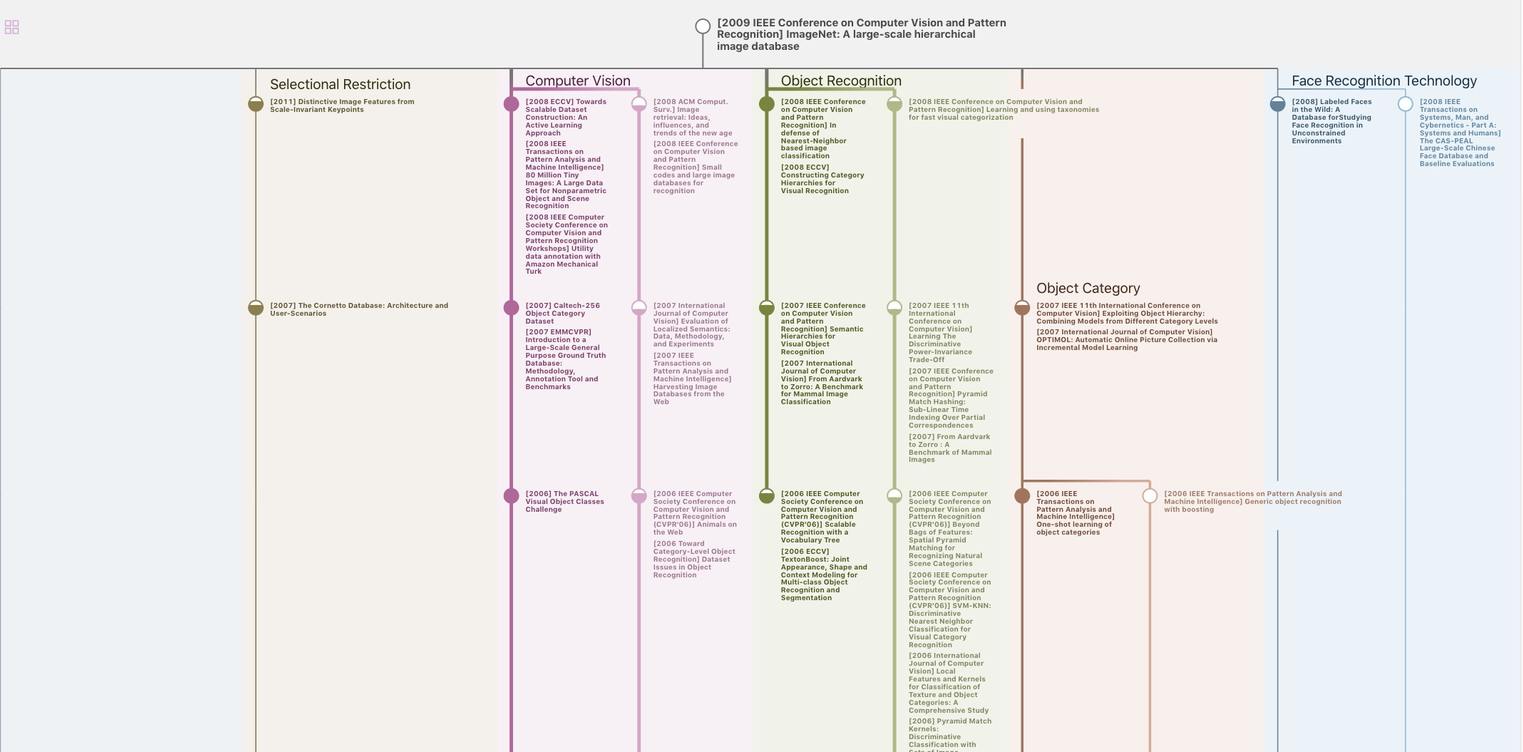
生成溯源树,研究论文发展脉络
Chat Paper
正在生成论文摘要