Real-Time Flow Scheduling in Industrial 5G New Radio.
2023 IEEE Real-Time Systems Symposium (RTSS)(2023)
摘要
Among the many industrial wireless solution candidates, 5G New Radio (NR) has drawn significant attention in recent years due to its capabilities to support ultra-high-speed communication, ultra-low latency, and massive connectivity. Despite its great potential, 5G NR also brings significant complexity in scheduling industrial data flows to meet their hard real-time requirements. In this paper, we first leverage a real-world 5G RAN testbed to benchmark the downlink throughput and explore the impact of modulation and coding scheme (MCS) selection on the network performance. We then formulate a real-time flow scheduling problem in industrial 5G NR, which features per-flow real-time schedulability guarantees through time-frequency-space resource allocation. We propose a novel two-phase scheduling framework, named 5G-TPS, to construct the schedule that meets the deadlines of all the flows. To adapt to dynamic channel conditions, 5G-TPS enables online schedule adjustment for affected flows to meet their timing requirements. To evaluate the performance of 5G-TPS, we present a case study of a motion control panel use case and perform extensive experiments. The results show that 5G-TPS can achieve schedulability ratios comparable to the Satisfiability Modulo Theory (SMT)-based exact solution and outperform many other state-of-the-art scheduling approaches, including the built-in 5G NR schedulers.
更多查看译文
关键词
Real-time Flow,Flow Scheduling,Industrial 5G,Resource Allocation,Dynamic Conditions,Extensive Experiments,Network Performance,Exact Solution,Channel State,Time Requirements,Scheduling Approach,Ultra-low Latency,Scheduling Framework,5G New Radio,Data Rate,Time Domain,Frequency Domain,Large Networks,Data Streams,User Equipment,Resource Block,Transmission Time Interval,Ultra-reliable Low-latency Communications,Entire Bandwidth,High Data Rate,Feasible Schedule,Number Of Streams,Subband,Channel Quality
AI 理解论文
溯源树
样例
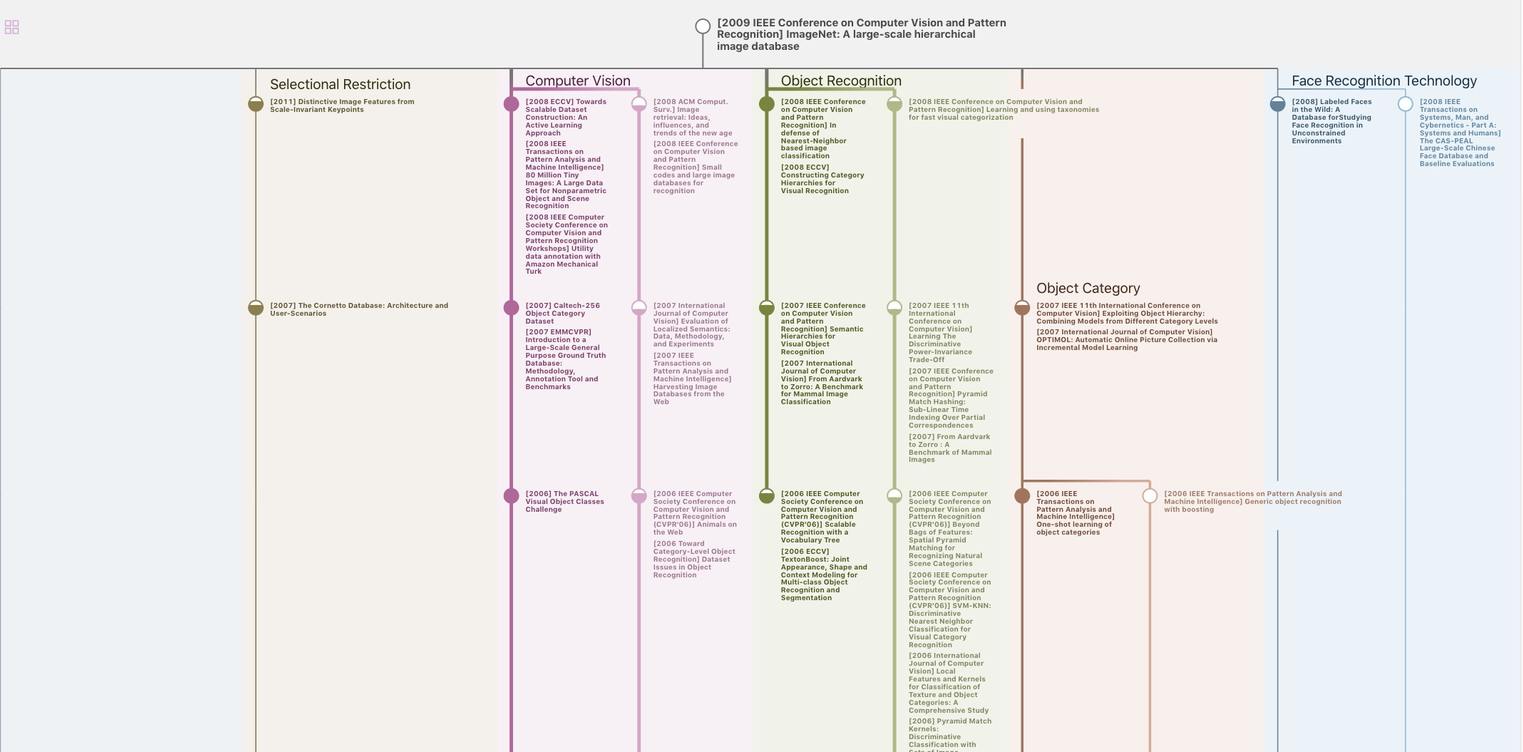
生成溯源树,研究论文发展脉络
Chat Paper
正在生成论文摘要