Towards Generalizability of Multi-Agent Reinforcement Learning in Graphs with Recurrent Message Passing.
AAMAS '24 Proceedings of the 23rd International Conference on Autonomous Agents and Multiagent Systems(2024)
摘要
Graph-based environments pose unique challenges to multi-agent reinforcementlearning. In decentralized approaches, agents operate within a given graph andmake decisions based on partial or outdated observations. The size of theobserved neighborhood limits the generalizability to different graphs andaffects the reactivity of agents, the quality of the selected actions, and thecommunication overhead. This work focuses on generalizability and resolves thetrade-off in observed neighborhood size with a continuous information flow inthe whole graph. We propose a recurrent message-passing model that iterateswith the environment's steps and allows nodes to create a global representationof the graph by exchanging messages with their neighbors. Agents receive theresulting learned graph observations based on their location in the graph. Ourapproach can be used in a decentralized manner at runtime and in combinationwith a reinforcement learning algorithm of choice. We evaluate our methodacross 1000 diverse graphs in the context of routing in communication networksand find that it enables agents to generalize and adapt to changes in thegraph.
更多查看译文
AI 理解论文
溯源树
样例
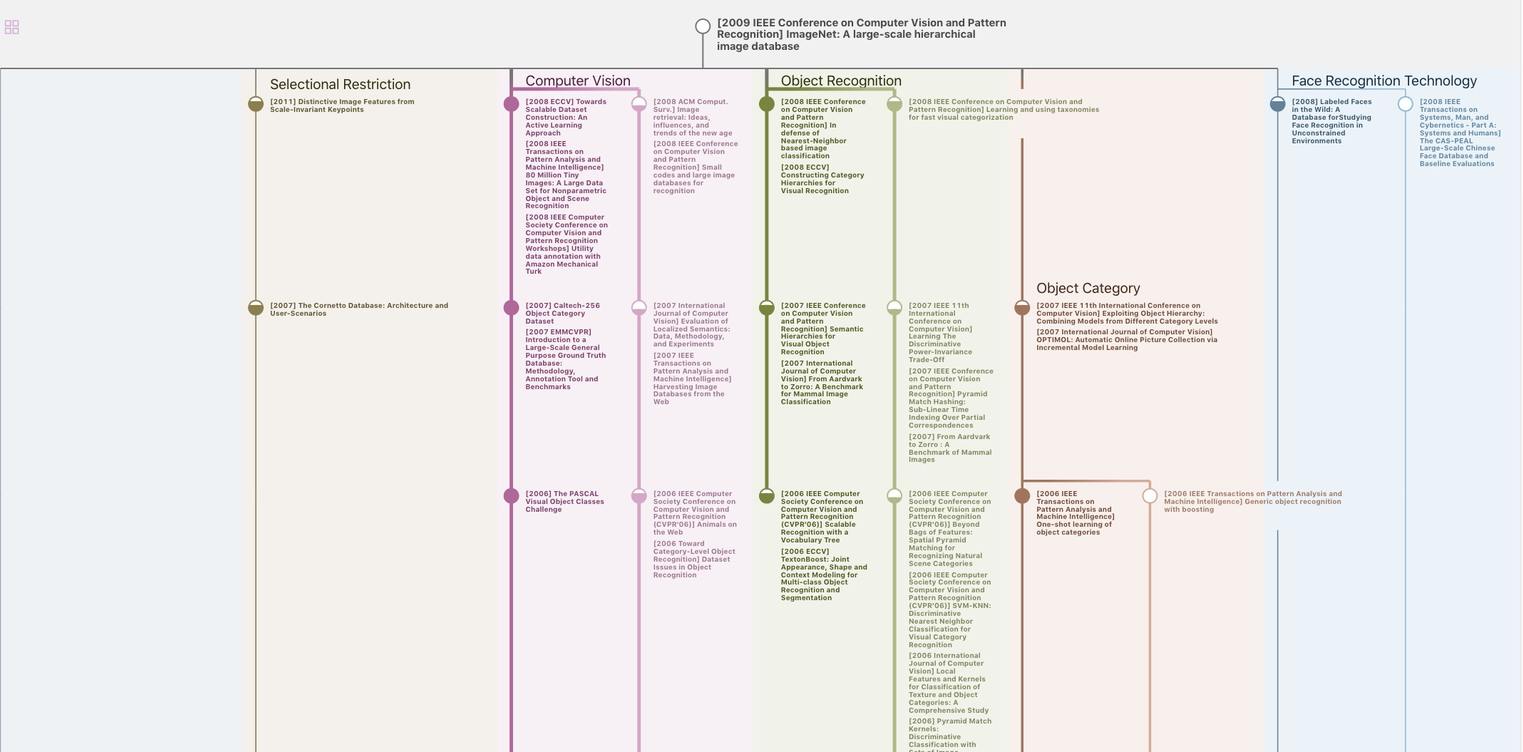
生成溯源树,研究论文发展脉络
Chat Paper
正在生成论文摘要