Imbalanced data fault diagnosis of rolling bearings using enhanced relative generative adversarial network
Journal of Mechanical Science and Technology(2024)
摘要
Rolling bearings, as integral components of rotating machinery, play a crucial role in ensuring the safe and stable operation of equipment. However, due to the inherent imbalance in the collected actual data, the accuracy of fault diagnosis is significantly compromised. An enhanced relative generative adversarial network (ERGAN) data augmentation model is proposed to improve fault diagnosis capability in imbalanced datasets. This paper introduces a relative loss function with a gradient penalty to enhance training stability and effectively address the mode collapse issue. Additionally, the generator and discriminator are reconstructed using one-dimensional convolutional layers and spectral normalization layers, which improves the quality of generated samples and effectively avoid gradient issues. Finally, an improved deep convolutional diagnostic model (DCNN) is established using variable convolutional kernels for fault classification. Experimental results demonstrate that, compared to other methods, this approach exhibits superior performance in imbalanced fault diagnosis tasks.
更多查看译文
关键词
Fault diagnosis,Generative adversarial network,Imbalanced data,Rolling bearings
AI 理解论文
溯源树
样例
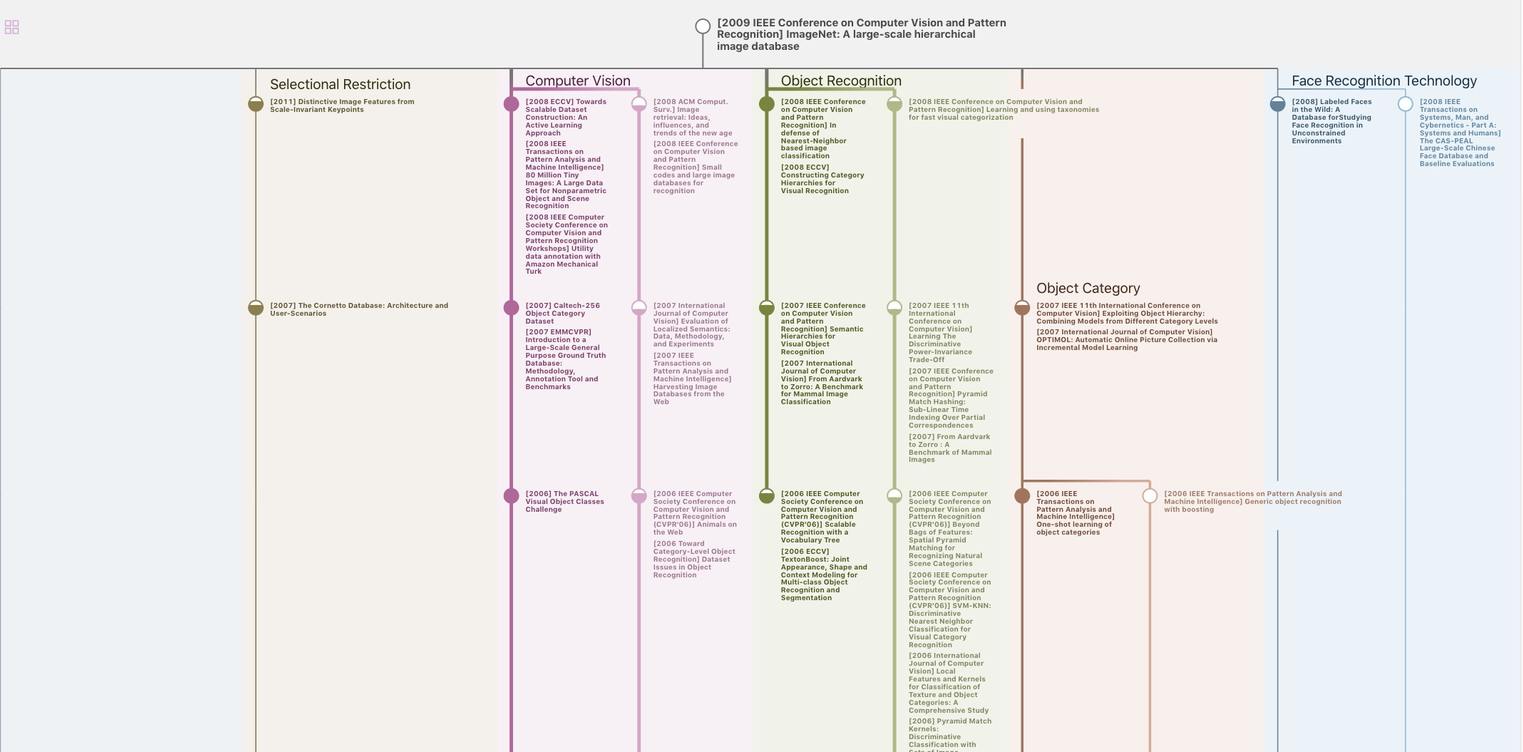
生成溯源树,研究论文发展脉络
Chat Paper
正在生成论文摘要