An Efficient Detection Model Based on Improved YOLOv5s for Abnormal Surface Features of Fish
Mathematical biosciences and engineering(2024)
摘要
Detecting abnormal surface features is an important method for identifying abnormal fish. However, existing methods face challenges in excessive subjectivity, limited accuracy, and poor real-time performance. To solve these challenges, a real-time and accurate detection model of abnormal surface features of in-water fish is proposed, based on improved YOLOv5s. The specific enhancements include: 1) We optimize the complete intersection over union and non-maximum suppression through the normalized Gaussian Wasserstein distance metric to improve the model's ability to detect tiny targets. 2) We design the DenseOne module to enhance the reusability of abnormal surface features, and introduce MobileViTv2 to improve detection speed, which are integrated into the feature extraction network. 3) According to the ACmix principle, we fuse the omni-dimensional dynamic convolution and convolutional block attention module to solve the challenge of extracting deep features within complex backgrounds. We carried out comparative experiments on 160 validation sets of in-water abnormal fish, achieving precision, recall, mAP50, mAP50:95 and frames per second (FPS) of 99.5, 99.1, 99.1, 73.9% and 88 FPS, respectively. The results of our model surpass the baseline by 1.4, 1.2, 3.2, 8.2% and 1 FPS. Moreover, the improved model outperforms other state-of-the-art models regarding comprehensive evaluation indexes.
更多查看译文
关键词
abnormal surface features of fish,yolov5s,normalized gaussian wasserstein distance metric,mobilevitv2 module,densone module,acmix,odc-cbam
AI 理解论文
溯源树
样例
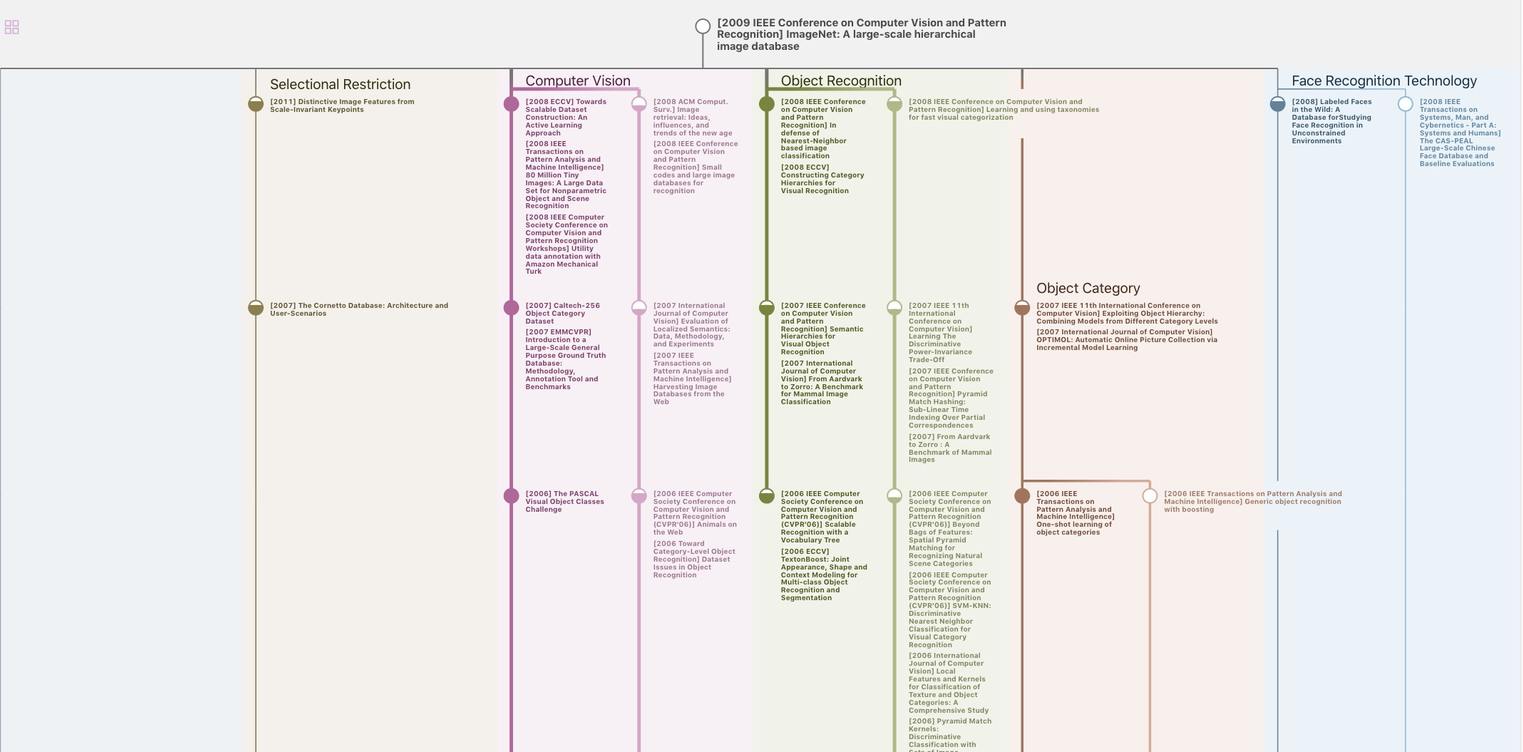
生成溯源树,研究论文发展脉络
Chat Paper
正在生成论文摘要