LMIE-BERT: A Learnable Method for Inter-Layer Ensembles to Accelerate Inference of BERT-Style Pre-trained Models.
International Conference on Big Data Computing and Communications(2023)
摘要
Pre-trained models have brought tremendous accuracy improvements to Natural Language Processing(NLP) and Computer Vision tasks, but they suffer from slow inference speed due to the heavy model, which hinders their deployment in production. The early exit methods have been proposed to accelerate the inference speed of large pre-trained models. However, these methods will lose control of accuracy at higher speed ratios. In order to balance the trade-off between model speed and accuracy better, we propose a novel early-exit mechanism called LMIE-BERT. To achieve this, we introduce a learnable method for inter-layer ensemble strategy in the internal classifier, it trains the model to fit the information from both the previous and current layers, which enables the early exit method to get more robust results. The experimental results demonstrate that LMIE-BERT can maintain over 90% of the accuracy of the original model while achieving a 4× inference speed up in multiple tasks. Our method is ahead of other early exit methods in terms of model accuracy for the same speed ratio.
更多查看译文
关键词
BERT,Model Compression,Early Exit
AI 理解论文
溯源树
样例
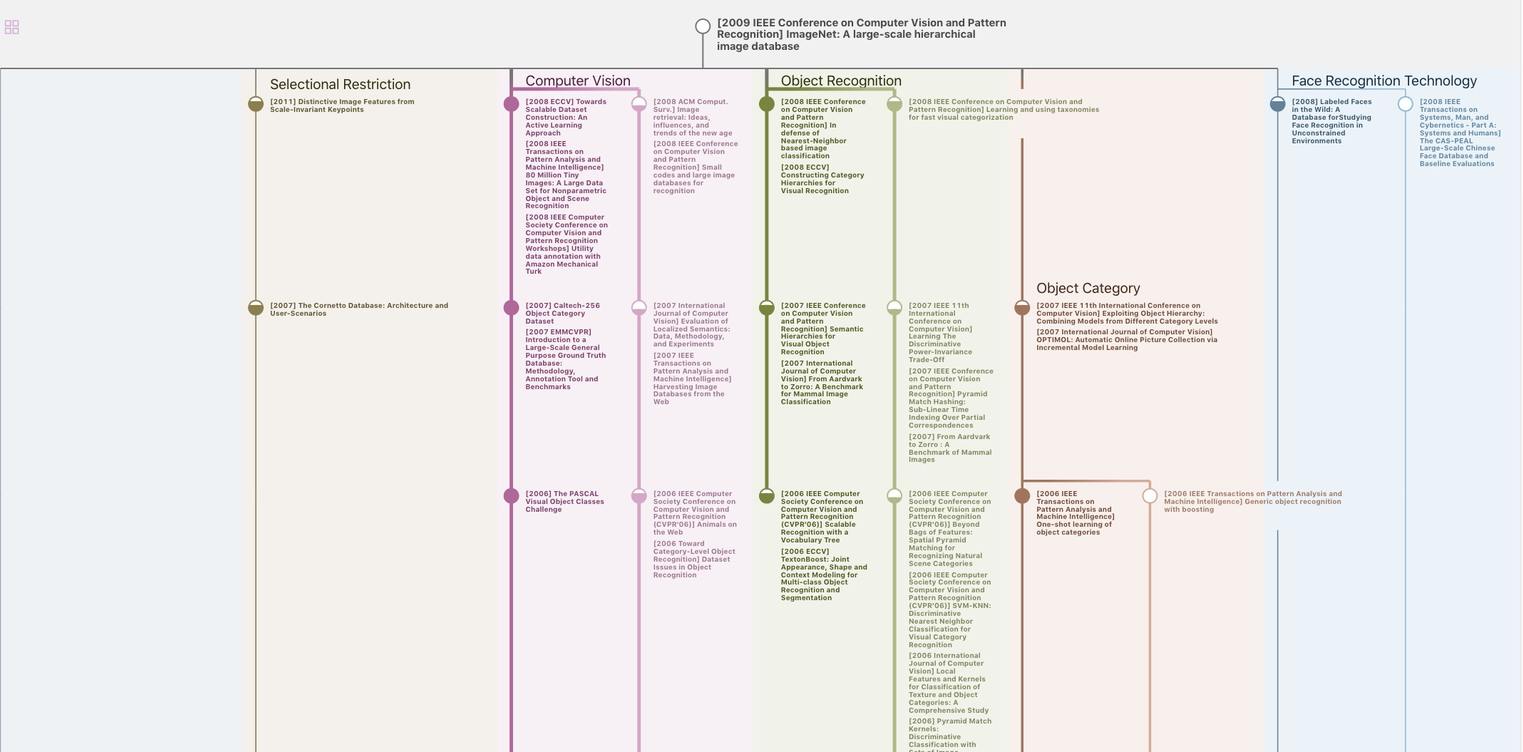
生成溯源树,研究论文发展脉络
Chat Paper
正在生成论文摘要