Attentive Learning Facilitates Generalization of Neural Networks
IEEE TRANSACTIONS ON NEURAL NETWORKS AND LEARNING SYSTEMS(2024)
Abstract
This article studies the generalization of neural networks (NNs) by examining how a network changes when trained on a training sample with or without out-of-distribution (OoD) examples. If the network's predictions are less influenced by fitting OoD examples, then the network learns attentively from the clean training set. A new notion, dataset-distraction stability, is proposed to measure the influence. Extensive CIFAR-10/100 experiments on the different VGG, ResNet, WideResNet, ViT architectures, and optimizers show a negative correlation between the dataset-distraction stability and generalizability. With the distraction stability, we decompose the learning process on the training set $\mathcal{S}$ into multiple learning processes on the subsets of $\mathcal{S}$ drawn from simpler distributions, i.e., distributions of smaller intrinsic dimensions (IDs), and furthermore, a tighter generalization bound is derived. Through attentive learning, miraculous generalization in deep learning can be explained and novel algorithms can also be designed.
MoreTranslated text
Key words
Deep learning generalization,explainable artificial intelligence (AI),learning mechanism,neural networks (NNs)
AI Read Science
Must-Reading Tree
Example
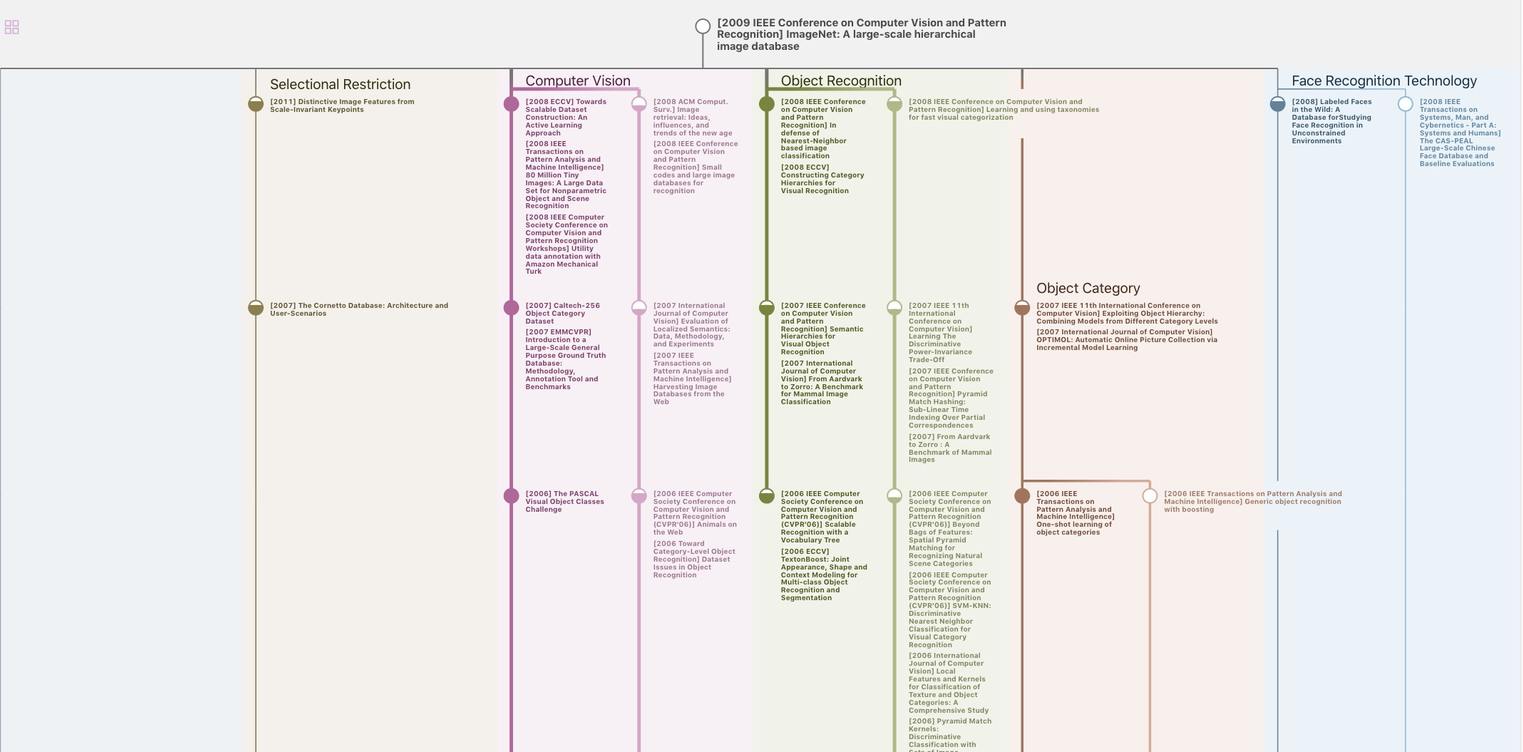
Generate MRT to find the research sequence of this paper
Chat Paper
Summary is being generated by the instructions you defined