Sharp Rates in Dependent Learning Theory: Avoiding Sample Size Deflation for the Square Loss
CoRR(2024)
摘要
In this work, we study statistical learning with dependent (β-mixing)
data and square loss in a hypothesis class ℱ⊂ L_Ψ_p
where Ψ_p is the norm f_Ψ_p≜sup_m≥ 1 m^-1/pf_L^m for some p∈ [2,∞]. Our inquiry is motivated by the
search for a sharp noise interaction term, or variance proxy, in learning with
dependent data. Absent any realizability assumption, typical non-asymptotic
results exhibit variance proxies that are deflated multiplicatively by
the mixing time of the underlying covariates process. We show that whenever the
topologies of L^2 and Ψ_p are comparable on our hypothesis class
ℱ – that is, ℱ is a weakly sub-Gaussian class:
f_Ψ_p≲f_L^2^η for some η∈ (0,1] – the
empirical risk minimizer achieves a rate that only depends on the complexity of
the class and second order statistics in its leading term. Our result holds
whether the problem is realizable or not and we refer to this as a near
mixing-free rate, since direct dependence on mixing is relegated to an
additive higher order term. We arrive at our result by combining the above
notion of a weakly sub-Gaussian class with mixed tail generic chaining. This
combination allows us to compute sharp, instance-optimal rates for a wide range
of problems.
to obtain sharp, instance-optimal rates. Examples that satisfy our framework
include sub-Gaussian linear regression, more general smoothly parameterized
function classes, finite hypothesis classes, and bounded smoothness classes.
更多查看译文
AI 理解论文
溯源树
样例
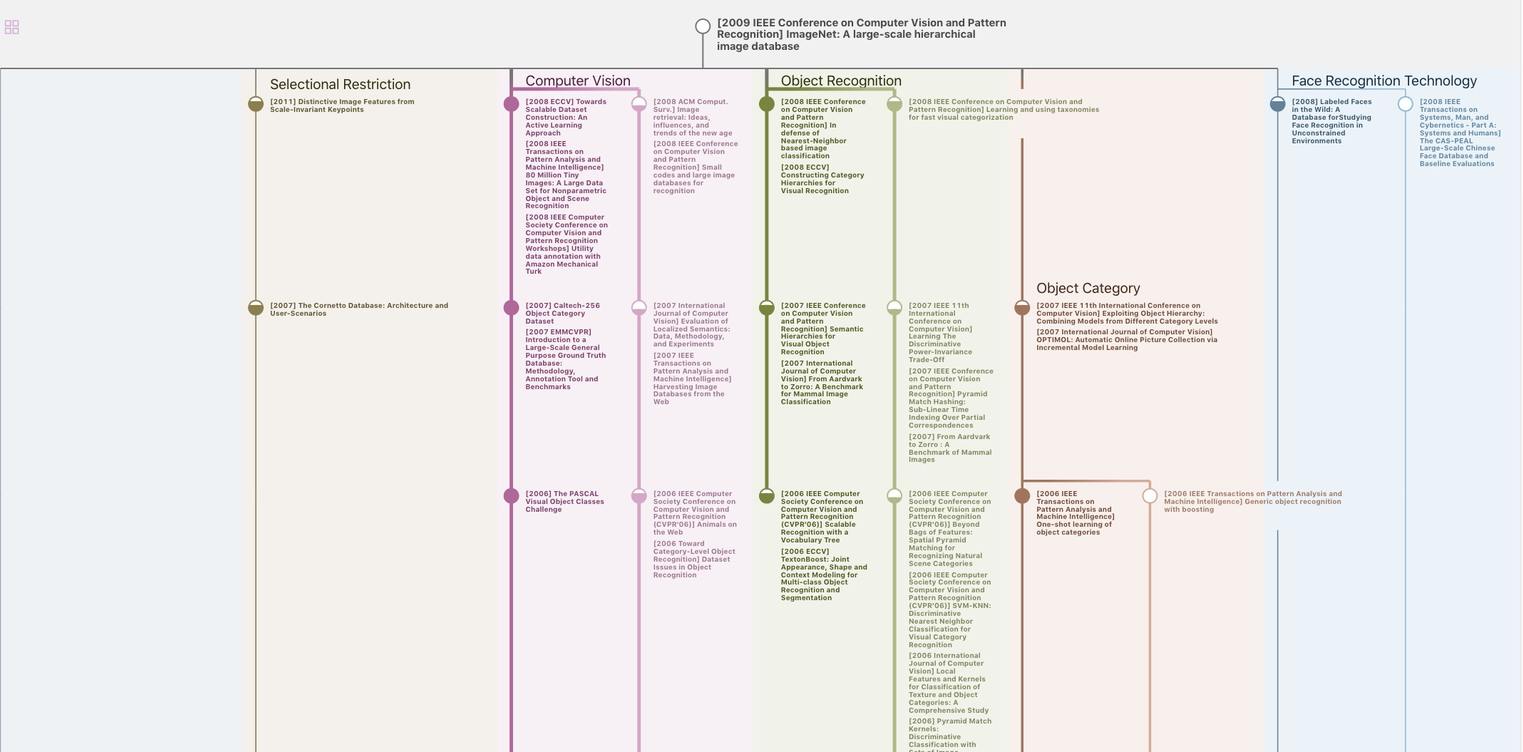
生成溯源树,研究论文发展脉络
Chat Paper
正在生成论文摘要