Convergence for Natural Policy Gradient on Infinite-State Average-Reward Markov Decision Processes
CoRR(2024)
摘要
Infinite-state Markov Decision Processes (MDPs) are essential in modeling and
optimizing a wide variety of engineering problems. In the reinforcement
learning (RL) context, a variety of algorithms have been developed to learn and
optimize these MDPs. At the heart of many popular policy-gradient based
learning algorithms, such as natural actor-critic, TRPO, and PPO, lies the
Natural Policy Gradient (NPG) algorithm. Convergence results for these RL
algorithms rest on convergence results for the NPG algorithm. However, all
existing results on the convergence of the NPG algorithm are limited to
finite-state settings.
We prove the first convergence rate bound for the NPG algorithm for
infinite-state average-reward MDPs, proving a O(1/√(T)) convergence rate,
if the NPG algorithm is initialized with a good initial policy. Moreover, we
show that in the context of a large class of queueing MDPs, the MaxWeight
policy suffices to satisfy our initial-policy requirement and achieve a
O(1/√(T)) convergence rate. Key to our result are state-dependent bounds
on the relative value function achieved by the iterate policies of the NPG
algorithm.
更多查看译文
AI 理解论文
溯源树
样例
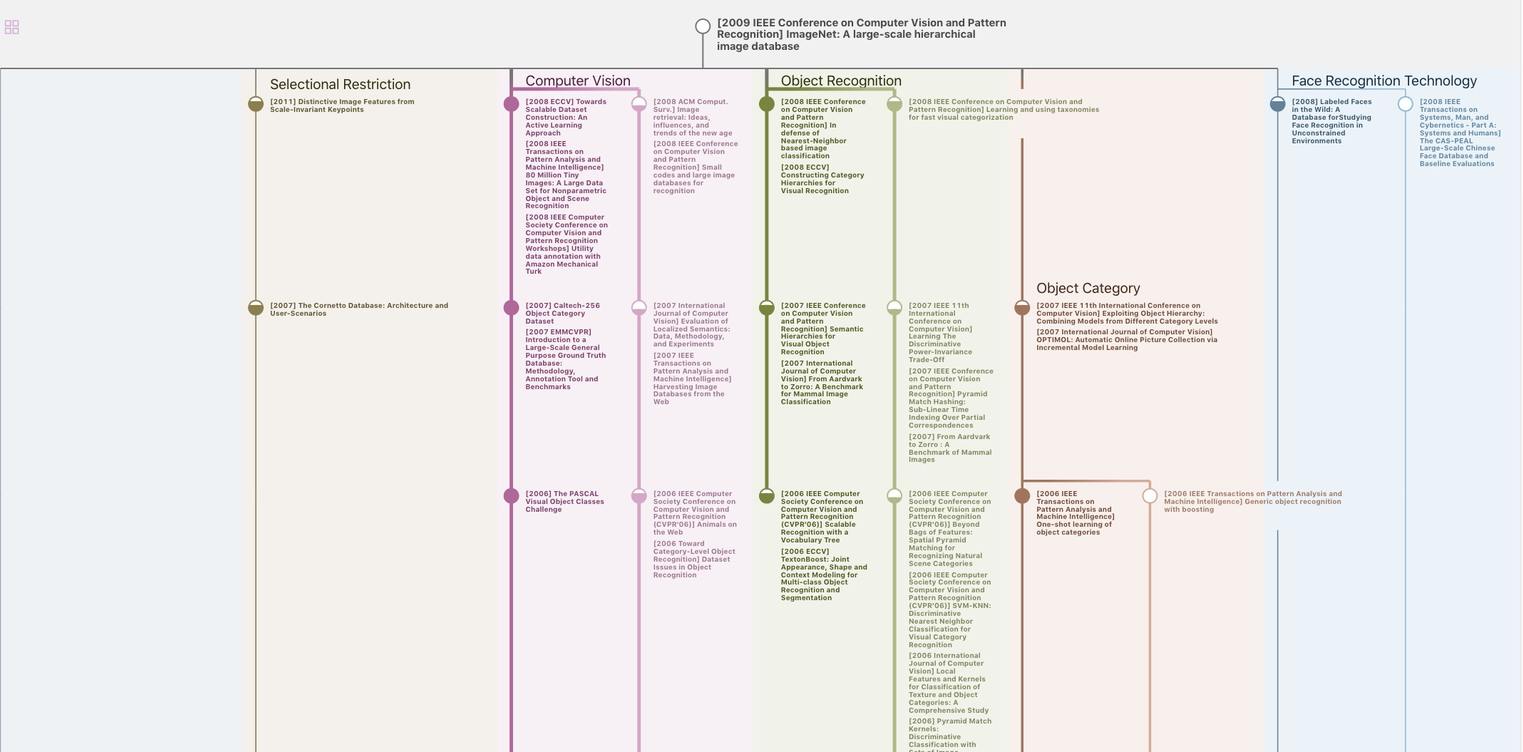
生成溯源树,研究论文发展脉络
Chat Paper
正在生成论文摘要