Harmfulness metrics in digital twins of social network rumors detection in cloud computing environment
Journal of Cloud Computing(2024)
摘要
Social network rumor harm metric is a task to score the harm caused by a rumor by analyzing the spreading range of the rumor, the users affected, the repercussions caused, etc., and then the harm caused by the rumor. Rumor hazard metric models can help rumor detection digital twins to understand and analyze user behaviors and assist social network network managers to make more informed decisions. However, there is a lack of models that can quantify the harm of rumors and automated harm metric models in rumor detection digital twins. To address this issue, this paper proposes an innovative social network rumor harm metric based on rumor propagation knowledge and a large language model (LLM), RSK-T5. The method first completes the joint task of rumor comment stance detection and sentiment analysis to capture critical features of rumor propagation. Then, this knowledge is used in the pre-training process of LLM to improve the model's understanding of rumor propagation patterns. Finally, the fine-tuning phase focuses on the hazard metrics task to improve the generalization energy. We compare with some existing variants of rumor detection methods, and experimental results demonstrate that RSK-T5 achieves the lowest MSE scores on three well-known rumor detection datasets. The ablative learning work demonstrates the effectiveness of RSK-T5's knowledge of two rumor spreads.
更多查看译文
关键词
Rumor hazard metric,Digital twins,Large language model,Rumor propagation,Rumor stance detection,Rumor sentiment analysis,Social network
AI 理解论文
溯源树
样例
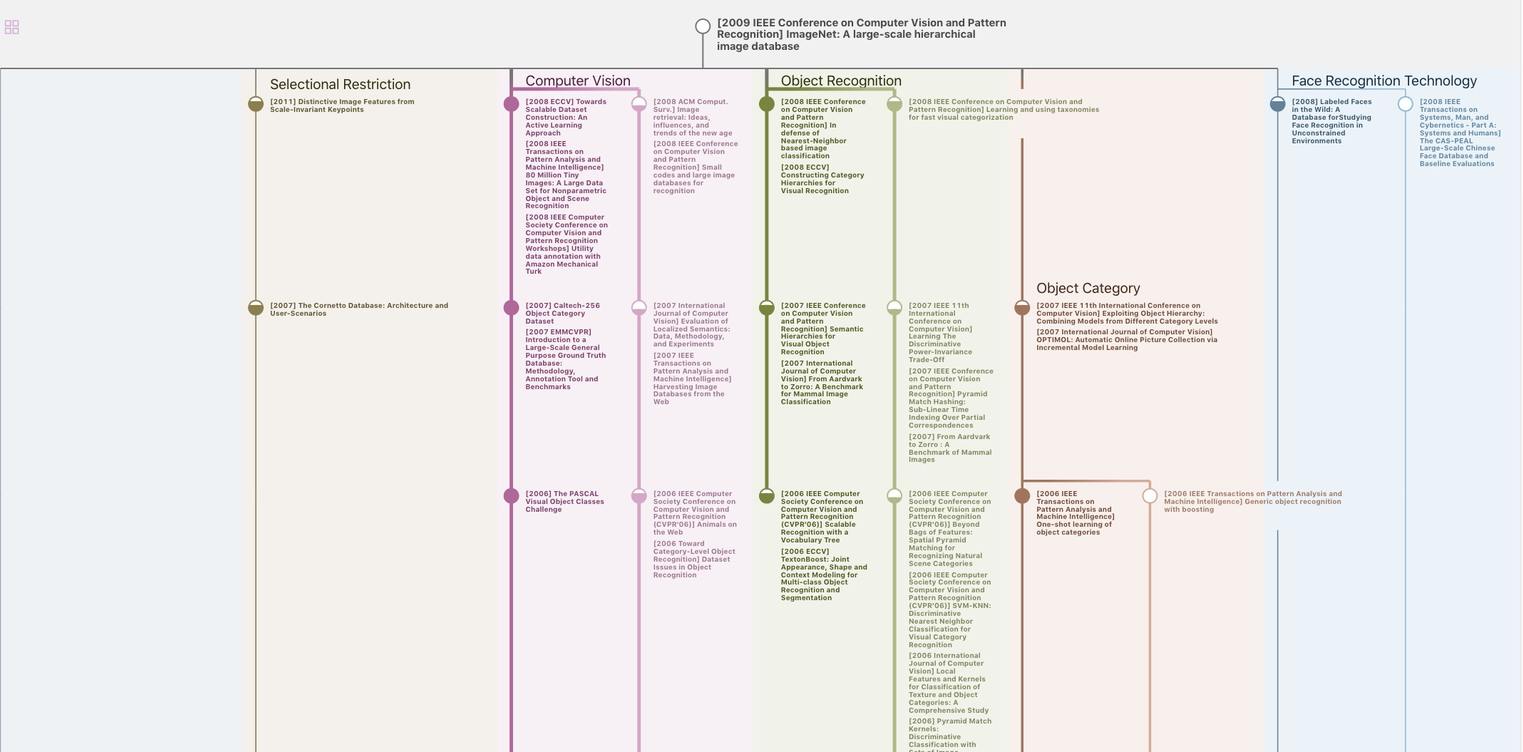
生成溯源树,研究论文发展脉络
Chat Paper
正在生成论文摘要