A Lightweight SAR Ship Detector Using End-to-End Image Preprocessing Network and Channel Feature Guided Spatial Pyramid Pooling
IEEE GEOSCIENCE AND REMOTE SENSING LETTERS(2024)
摘要
Recently, in the field of synthetic aperture radar (SAR) ship detection, deep-learning-based methods have made significant strides in terms of detection accuracy and speed. However, small-scale targets and complex backgrounds remain a formidable obstacle to SAR ship detection. To overcome the aforementioned challenges, this letter proposes LiteSAR-Net, a lightweight SAR ship detector, to enhance ship detection capabilities in SAR imagery. In detail, an end-to-end image preprocessing network (E2IPNet) is proposed to strengthen context information and expand the network's effective receptive field. In addition, to prevent the dilution of semantic information, the channel feature guided spatial pyramid pooling (CFGSPP) is proposed, which can adjust the parameters adaptively based on interchannel information. The proposed LiteSAR-Net achieved an average precision (AP) of 98.61% on the SAR ship detection dataset (SSDD) and 93.33% on the high-resolution SAR images dataset (HRSID), with a parameter of only 5.247 M, outperformed many state-of-the-art (SOTA) detectors.
更多查看译文
关键词
Marine vehicles,Feature extraction,Detectors,Convolution,Radar polarimetry,Kernel,Synthetic aperture radar,Deep learning,lightweight,object detection,ship detection,synthetic aperture radar (SAR)
AI 理解论文
溯源树
样例
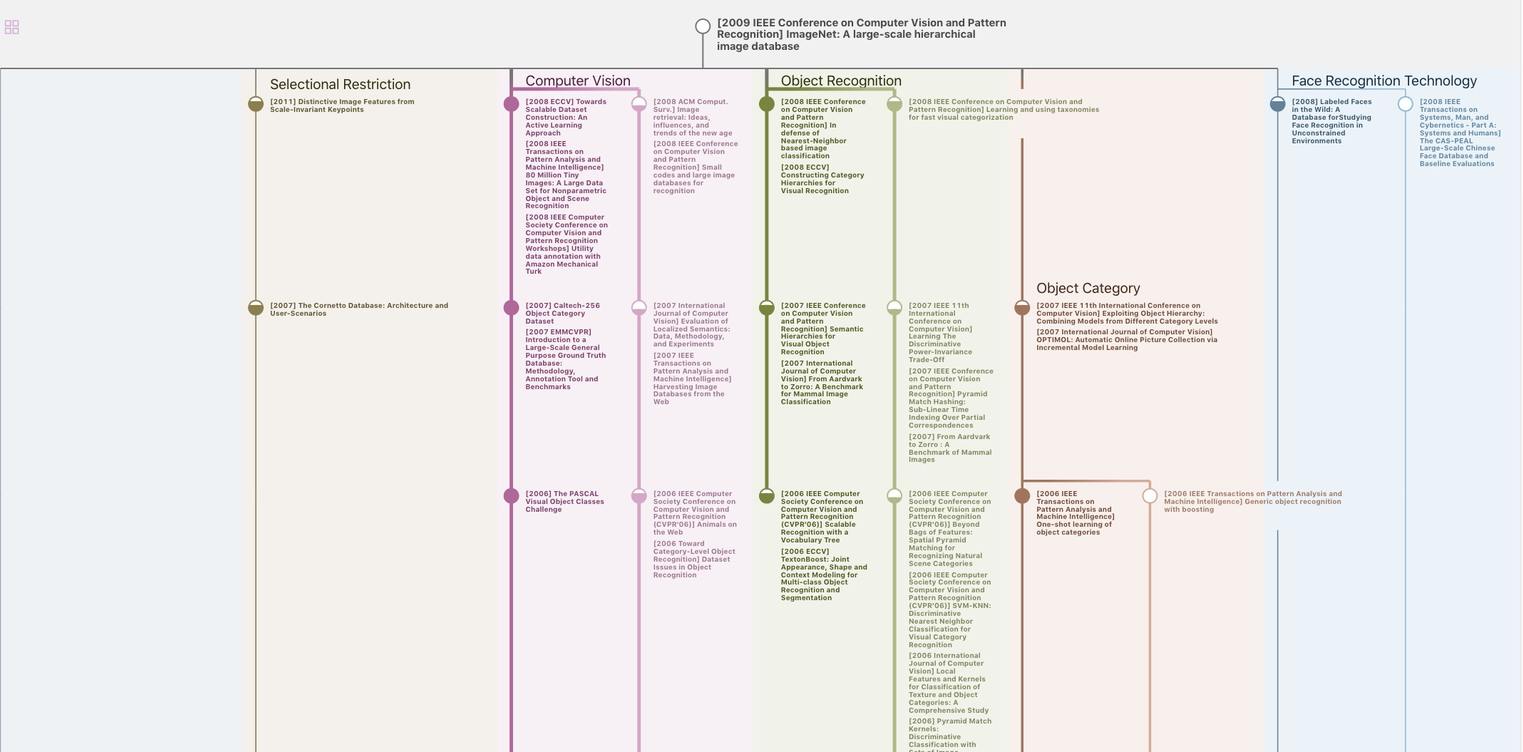
生成溯源树,研究论文发展脉络
Chat Paper
正在生成论文摘要