A new paradigm in electron microscopy: Automated microstructure analysis utilizing a dynamic segmentation convolutional neutral network
MATERIALS TODAY ADVANCES(2024)
摘要
Over the past half century, the transmission electron microscope enabled insight into the fundamental arrangements and structures of materials. State-of-the-art electron microscopes can acquire large image datasets across multiple imaging modalities. However, the manual annotation process for feature or defect quantification may not be feasible with the modern microscope. Convolutional neural networks emerged to characterize individual microstructural features from an image in a cost-effective, consistent manner. However, many of these neural network approaches rely on thousands to hundreds of thousands of manual annotations of each feature type across hundreds of images to train the network for adequate performance. This work focused on the development and application of a pixel -wise defect detection machine -learning dynamic segmentation convolutional neural network with associated automated acquisition and postprocessing to identify microstructural features rapidly and quantitatively from a small initial dataset incorporating multiple imaging modes. The approach was demonstrated for characterization of superalloy 718 from both single image acquisition on multiple detectors to in -situ evolution captured with a single detector on a standard desktop computer to demonstrate the low barrier to entry required for widespread adoption. Pixel -by -pixel class identification was excellent with strong identification of chemically distinct phases, structurally distinct phases, and defect structures, thus demonstrating the new paradigm of machine learning -assisted characterization.
更多查看译文
关键词
TEM,Microstructure,Machine learning,Automation
AI 理解论文
溯源树
样例
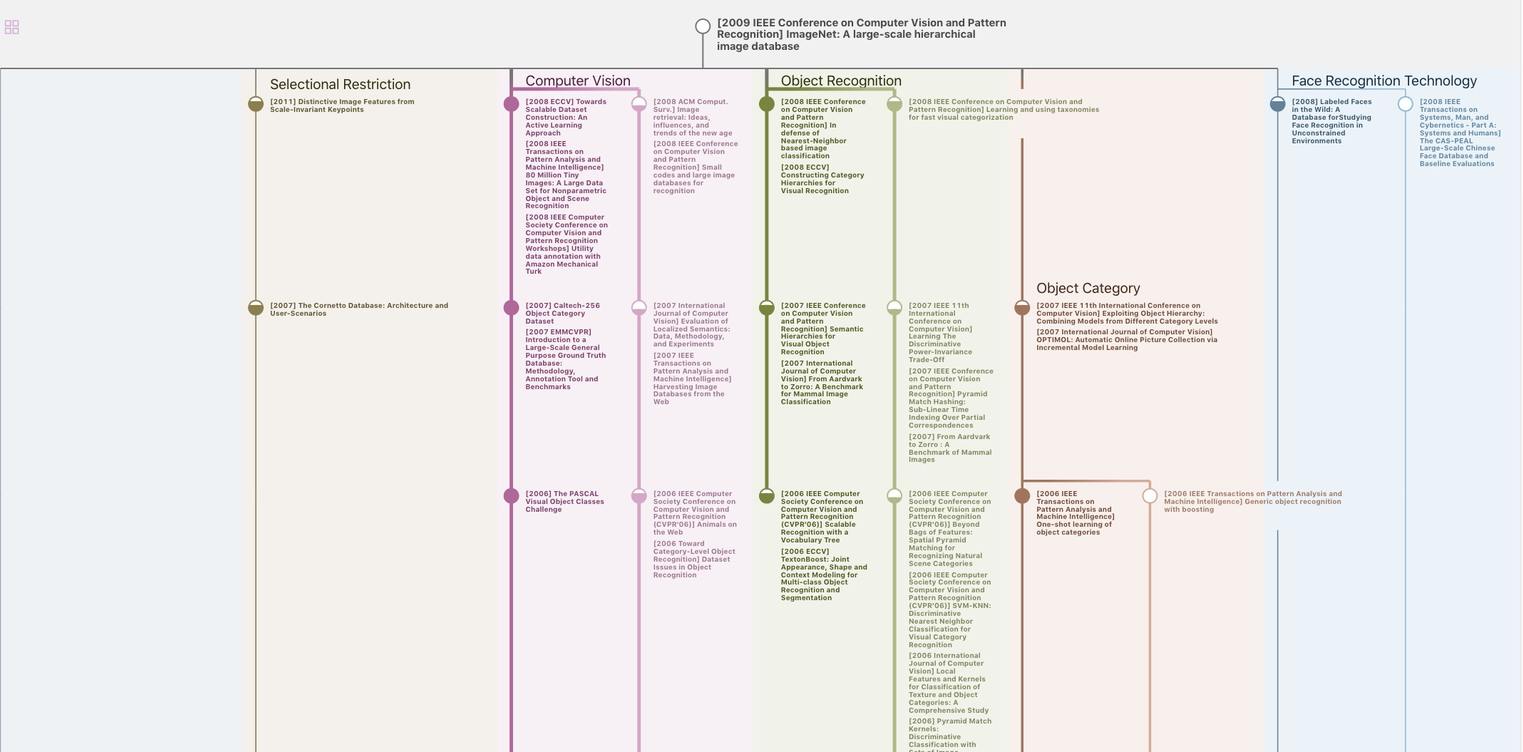
生成溯源树,研究论文发展脉络
Chat Paper
正在生成论文摘要