Comprehensive vulnerability aspect extraction
Applied Intelligence(2024)
摘要
Extracting valuable information from unstructured vulnerability reports constitutes a fundamental task in numerous cybersecurity applications. Existing approaches necessitate the creation of new extraction models and data labeling efforts, also inadvertently leading to duplicated information extraction. Therefore, we devote to extracting almost all valuable aspects at in a single sweep to benefit most downstream tasks. However, comprehensive extraction is challenging, which not only increases the boundaries of the aspects to be located but also reduces the number of learnable words in a vulnerability report. In this paper, we propose the Vul nerability P ortrait A utomatic G enerator (Vul-PAG), designed to facilitate comprehensive vulnerability aspect extraction by capturing and amalgamating word’s multi-view information. It encompasses a split-reorganization mechanism based on the wordpiece mechanism to capture the internal writing feature of words alongside a MidConst task to grasp the syntactic feature of words. Further, we fuse them with the semantic feature output from the context-dependent language model to bolster the word’s representation ability. Furthermore, we present the first-ever dataset crafted for the comprehensive extraction of vulnerability aspects, containing 2200 descriptions and encompassing eight distinct aspects. Extensive experimental results show that Vul-PAG outperforms state-of-the-art methods by 3.47, 2.68, and 3.07 in terms of precision, recall, and F1-score, respectively.
更多查看译文
关键词
Cyber security,Vulnerability reports,Vulnerability aspect extraction,Multi-view information
AI 理解论文
溯源树
样例
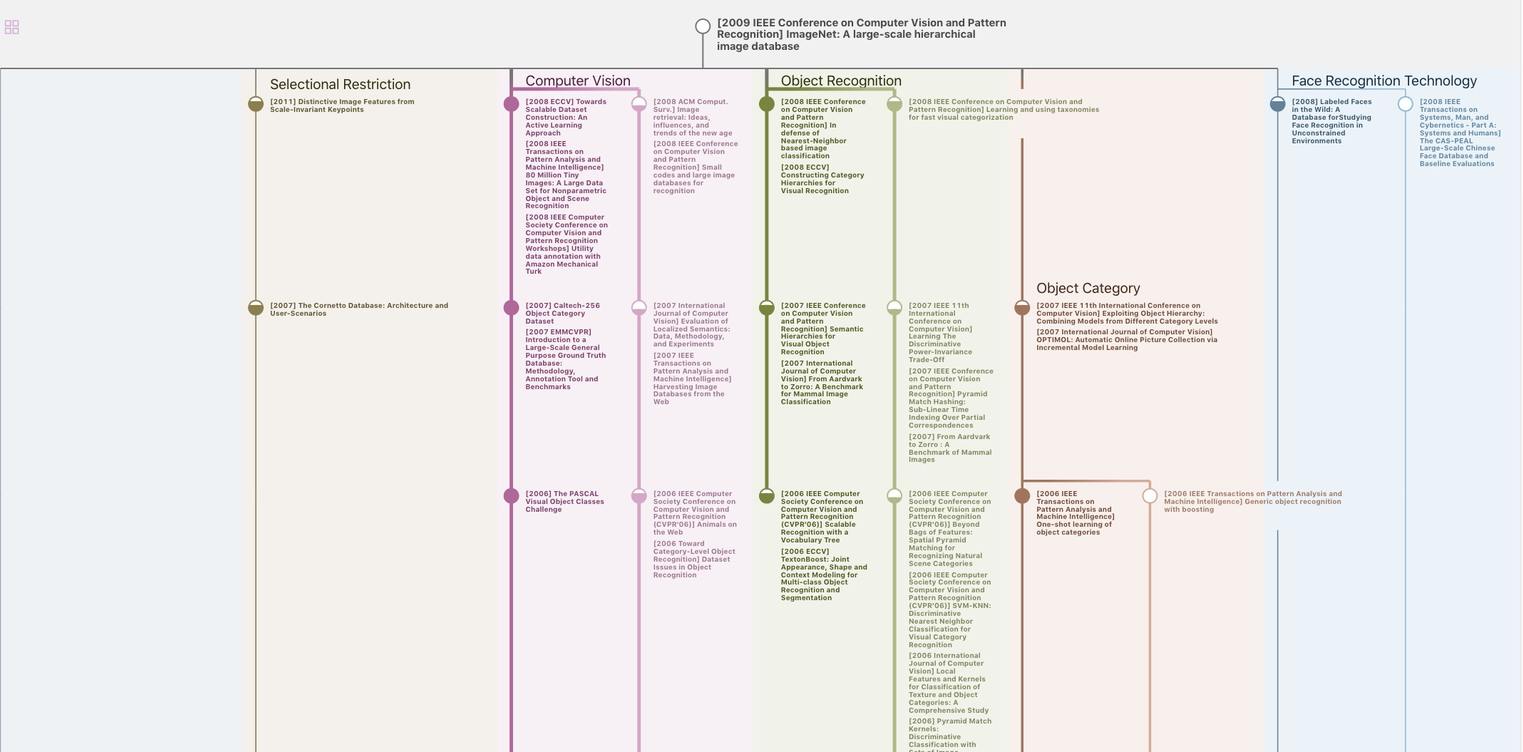
生成溯源树,研究论文发展脉络
Chat Paper
正在生成论文摘要