Multi-omics assists genomic prediction of maize yield with machine learning approaches
Molecular Breeding(2024)
摘要
With the improvement of high-throughput technologies in recent years, large multi-dimensional plant omics data have been produced, and big-data-driven yield prediction research has received increasing attention. Machine learning offers promising computational and analytical solutions to interpret the biological meaning of large amounts of data in crops. In this study, we utilized multi-omics datasets from 156 maize recombinant inbred lines, containing 2496 single nucleotide polymorphisms (SNPs), 46 image traits (i-traits) from 16 developmental stages obtained through an automatic phenotyping platform, and 133 primary metabolites. Based on benchmark tests with different types of prediction models, some machine learning methods, such as Partial Least Squares (PLS), Random Forest (RF), and Gaussian process with Radial basis function kernel (GaussprRadial), achieved better prediction for maize yield, albeit slight difference for method preferences among i-traits, genomic, and metabolic data. We found that better yield prediction may be caused by various capabilities in ranking and filtering data features, which is found to be linked with biological meaning such as photosynthesis-related or kernel development-related regulations. Finally, by integrating multiple omics data with the RF machine learning approach, we can further improve the prediction accuracy of grain yield from 0.32 to 0.43. Our research provides new ideas for the application of plant omics data and artificial intelligence approaches to facilitate crop genetic improvements.
更多查看译文
关键词
Yield prediction,Multi-omics,Machine learning,Crop breeding
AI 理解论文
溯源树
样例
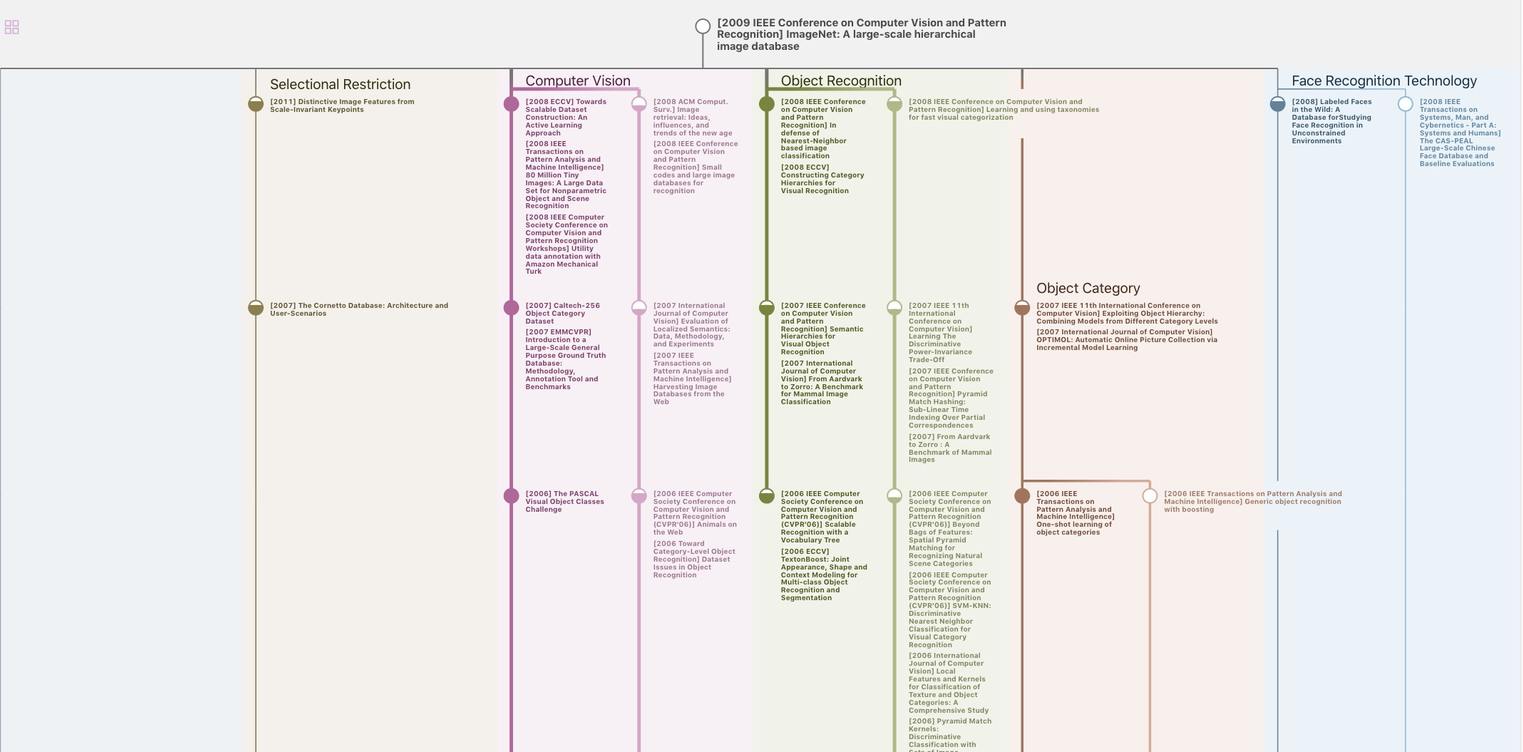
生成溯源树,研究论文发展脉络
Chat Paper
正在生成论文摘要