A Comprehensive Investigation of Active Learning Strategies for Conducting Anti-Cancer Drug Screening
CANCERS(2024)
摘要
Simple Summary Preclinical drug screening experiments for anti-cancer drug discovery typically involve testing candidate drugs against cancer cell lines. This process can be expensive and time consuming since the possible experimental space can be quite huge, involving all of the combinations of candidate cell lines and drugs. Guiding drug screening experiments with active learning strategies could potentially identify promising candidates for successful experimentation. This study investigates various active learning strategies for selecting experiments to generate response data for identifying effective treatments and improving the performance of drug response prediction models. We have demonstrated that most active learning strategies are more efficient than random selection for identifying effective treatments.Abstract It is well-known that cancers of the same histology type can respond differently to a treatment. Thus, computational drug response prediction is of paramount importance for both preclinical drug screening studies and clinical treatment design. To build drug response prediction models, treatment response data need to be generated through screening experiments and used as input to train the prediction models. In this study, we investigate various active learning strategies of selecting experiments to generate response data for the purposes of (1) improving the performance of drug response prediction models built on the data and (2) identifying effective treatments. Here, we focus on constructing drug-specific response prediction models for cancer cell lines. Various approaches have been designed and applied to select cell lines for screening, including a random, greedy, uncertainty, diversity, combination of greedy and uncertainty, sampling-based hybrid, and iteration-based hybrid approach. All of these approaches are evaluated and compared using two criteria: (1) the number of identified hits that are selected experiments validated to be responsive, and (2) the performance of the response prediction model trained on the data of selected experiments. The analysis was conducted for 57 drugs and the results show a significant improvement on identifying hits using active learning approaches compared with the random and greedy sampling method. Active learning approaches also show an improvement on response prediction performance for some of the drugs and analysis runs compared with the greedy sampling method.
更多查看译文
关键词
active learning,machine learning,drug response prediction,drug discovery,cancer
AI 理解论文
溯源树
样例
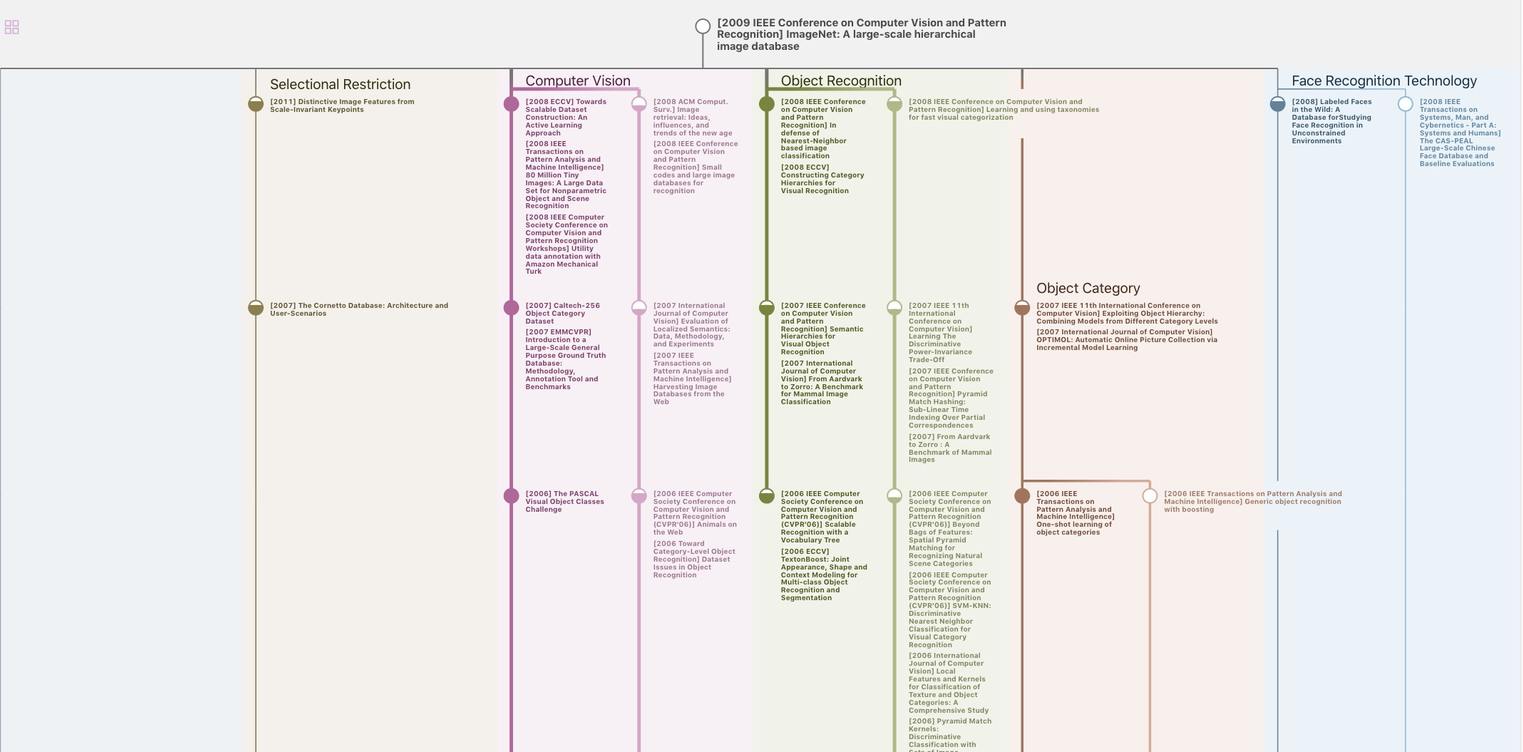
生成溯源树,研究论文发展脉络
Chat Paper
正在生成论文摘要