Dynamic Spatial-Spectral Feature Optimization-Based Point Cloud Classification
Remote sensing(2024)
摘要
With the development and popularization of LiDAR technology, point clouds are becoming widely used in multiple fields. Point cloud classification plays an important role in segmentation, geometric analysis, and vegetation description. However, existing point cloud classification algorithms have problems such as high computational complexity, a lack of feature optimization, and low classification accuracy. This paper proposes an efficient point cloud classification algorithm based on dynamic spatial-spectral feature optimization. It can eliminate redundant features, optimize features, reduce computational costs, and improve classification accuracy. It achieves feature optimization through three key steps. First, the proposed method extracts spatial, geometric, spectral, and other features from point cloud data. Then, the Gini index and Fisher score are used to calculate the importance and relevance of features, and redundant features are filtered. Finally, feature importance factors are used to dynamically enhance the discriminative power of highly distinguishable features to strengthen their contribution to point cloud classification. Four real-scene datasets from STPLS3D are utilized for experimentation. Compared to the other five algorithms, the proposed algorithm achieves at least a 37.97% improvement in mean intersection over union (mIoU). Meanwhile, the results indicate that the proposed algorithm can achieve high-precision point cloud classification with low computational complexity.
更多查看译文
关键词
LiDAR,point cloud classification,feature fusion,random forest (RF)
AI 理解论文
溯源树
样例
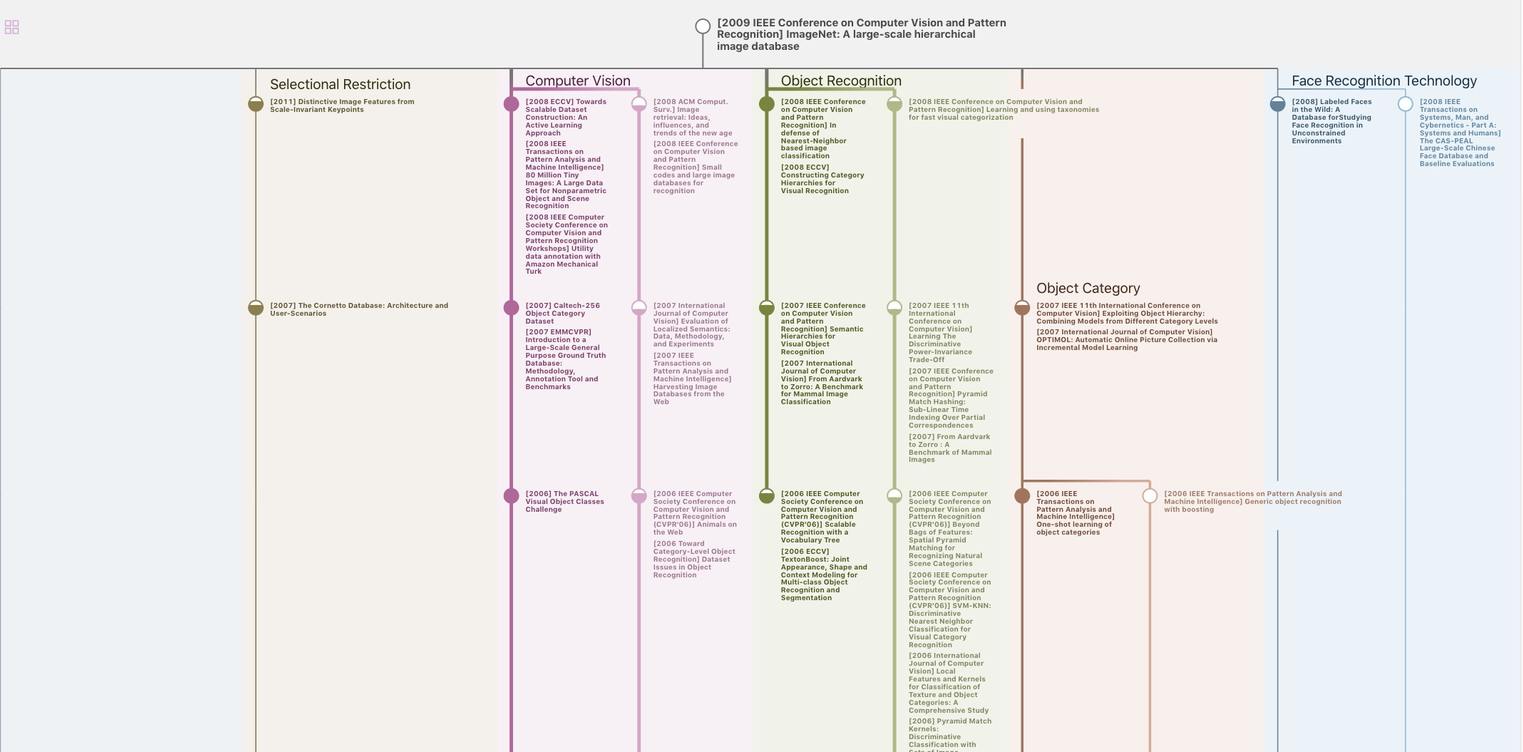
生成溯源树,研究论文发展脉络
Chat Paper
正在生成论文摘要