Dual-Task Network for Terrace and Ridge Extraction: Automatic Terrace Extraction via Multi-Task Learning
REMOTE SENSING(2024)
摘要
Terrace detection and ridge extraction from high-resolution remote sensing imagery are crucial for soil conservation and grain production on sloping land. Traditional methods use low-to-medium resolution images, missing detailed features and lacking automation. Terrace detection and ridge extraction are closely linked, with each influencing the other's outcomes. However, most studies address these tasks separately, overlooking their interdependence. This research introduces a cutting-edge, multi-scale, and multi-task deep learning framework, termed DTRE-Net, designed for comprehensive terrace information extraction. This framework bridges the gap between terrace detection and ridge extraction, executing them concurrently. The network incorporates residual networks, multi-scale fusion modules, and multi-scale residual correction modules to enhance the model's robustness in feature extraction. Comprehensive evaluations against other deep learning-based semantic segmentation methods using GF-2 terraced imagery from two distinct areas were undertaken. The results revealed intersection over union (IoU) values of 85.18% and 86.09% for different terrace morphologies and 59.79% and 73.65% for ridges. Simultaneously, we have confirmed that the connectivity of results is improved when employing multi-task learning for ridge extraction compared to directly extracting ridges. These outcomes underscore DTRE-Net's superior capability in the automation of terrace and ridge extraction relative to alternative techniques.
更多查看译文
关键词
multi-task learning,terrace information extraction,neural networks,high-resolution remote sensing images
AI 理解论文
溯源树
样例
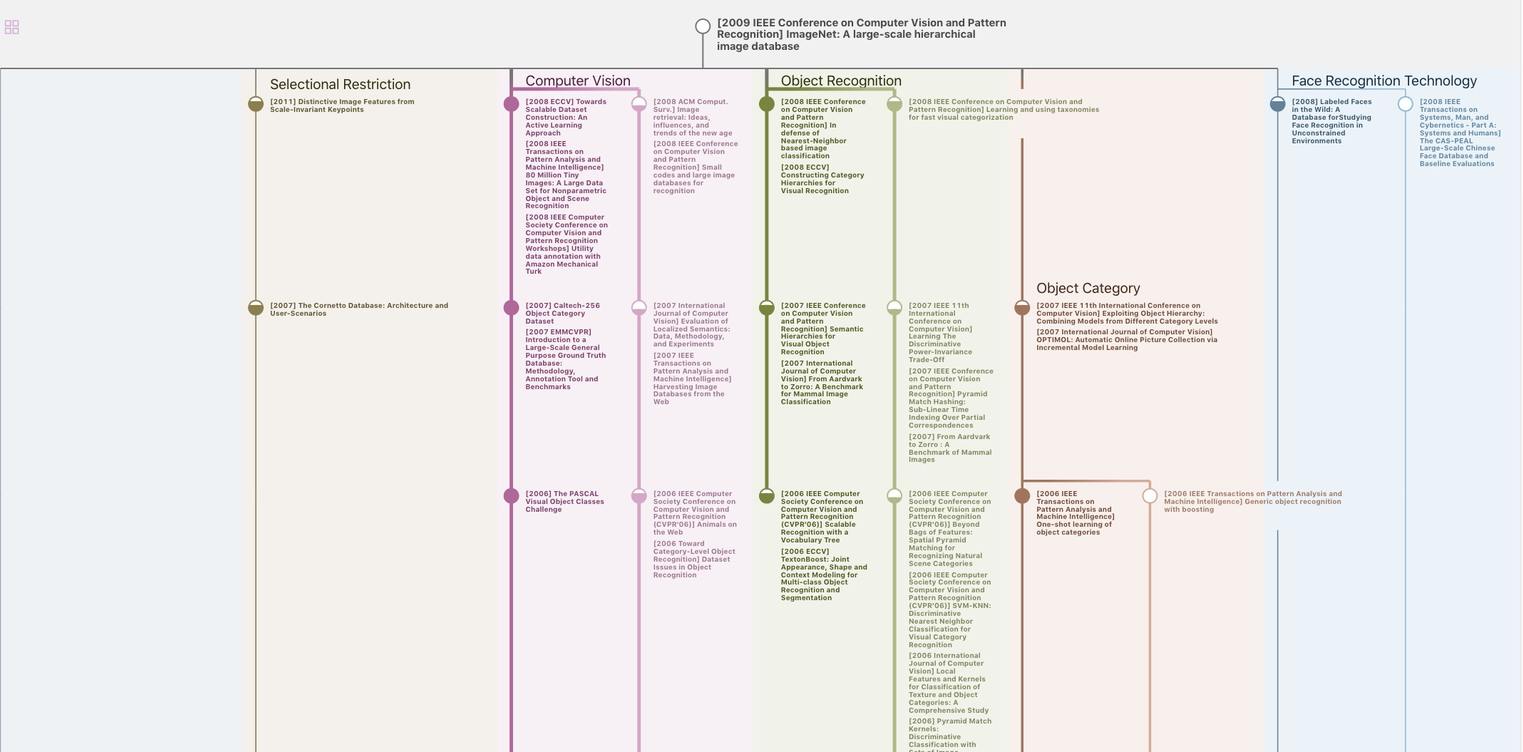
生成溯源树,研究论文发展脉络
Chat Paper
正在生成论文摘要