Integrated Framework for Unsupervised Building Segmentation with Segment Anything Model-Based Pseudo-Labeling and Weakly Supervised Learning
REMOTE SENSING(2024)
摘要
The Segment Anything Model (SAM) has had a profound impact on deep learning applications in remote sensing. SAM, which serves as a prompt-based foundation model for segmentation, exhibits a remarkable capability to "segment anything," including building objects on satellite or airborne images. To facilitate building segmentation without inducing supplementary prompts or labels, we applied a sequential approach of generating pseudo-labels and incorporating an edge-driven model. We first segmented the entire scene by SAM and masked out unwanted objects to generate pseudo-labels. Subsequently, we employed an edge-driven model designed to enhance the pseudo-label by using edge information to reconstruct the imperfect building features. Our model simultaneously utilizes spectral features from SAM-oriented building pseudo-labels and edge features from resultant images from the Canny edge detector and, thus, when combined with conditional random fields (CRFs), shows capability to extract and learn building features from imperfect pseudo-labels. By integrating the SAM-based pseudo-label with our edge-driven model, we establish an unsupervised framework for building segmentation that operates without explicit labels. Our model excels in extracting buildings compared with other state-of-the-art unsupervised segmentation models and even outperforms supervised models when trained in a fully supervised manner. This achievement demonstrates the potential of our model to address the lack of datasets in various remote sensing domains for building segmentation.
更多查看译文
关键词
semantic segmentation,unsupervised building extraction,edge-driven model,pseudo-label
AI 理解论文
溯源树
样例
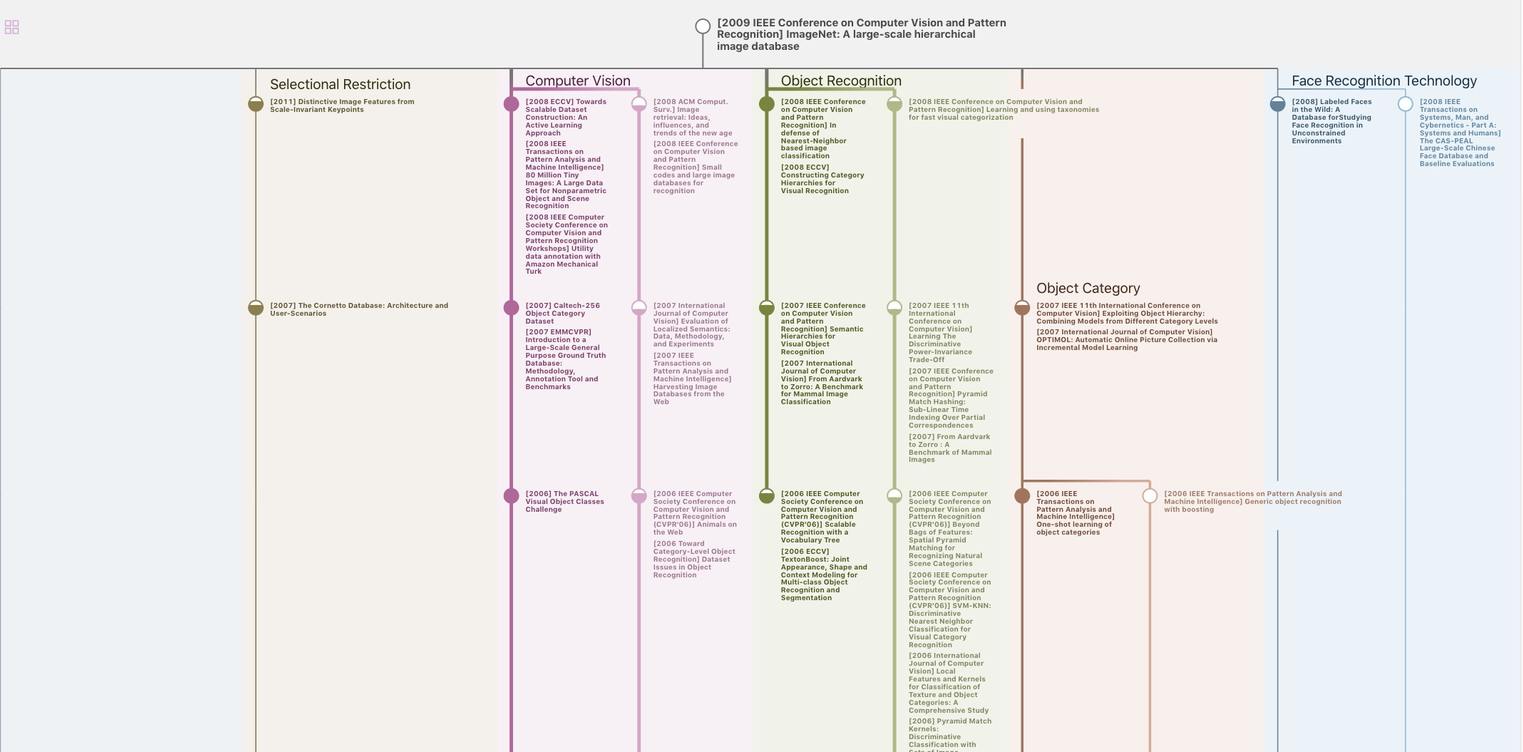
生成溯源树,研究论文发展脉络
Chat Paper
正在生成论文摘要