Disparity Refinement for Stereo Matching of High-Resolution Remote Sensing Images Based on GIS Data
Remote Sensing(2024)
摘要
With the emergence of the Smart City concept, the rapid advancement of urban three-dimensional (3D) reconstruction becomes imperative. While current developments in the field of 3D reconstruction have enabled the generation of 3D products such as Digital Surface Models (DSM), challenges persist in accurately reconstructing shadows, handling occlusions, and addressing low-texture areas in very-high-resolution remote sensing images. These challenges often lead to difficulties in calculating satisfactory disparity maps using existing stereo matching methods, thereby reducing the accuracy of 3D reconstruction. This issue is particularly pronounced in urban scenes, which contain numerous super high-rise and densely distributed buildings, resulting in large disparity values and occluded regions in stereo image pairs, and further leading to a large number of mismatched points in the obtained disparity map. In response to these challenges, this paper proposes a method to refine the disparity in urban scenes based on open-source GIS data. First, we register the GIS data with the epipolar-rectified images since there always exists unignorable geolocation errors between them. Specifically, buildings with different heights present different offsets in GIS data registering; thus, we perform multi-modal matching for each building and merge them into the final building mask. Subsequently, a two-layer optimization process is applied to the initial disparity map based on the building mask, encompassing both global and local optimization. Finally, we perform a post-correction on the building facades to obtain the final refined disparity map that can be employed for high-precision 3D reconstruction. Experimental results on SuperView-1, GaoFen-7, and GeoEye satellite images show that the proposed method has the ability to correct the occluded and mismatched areas in the initial disparity map generated by both hand-crafted and deep-learning stereo matching methods. The DSM generated by the refined disparity reduces the average height error from 2.2 m to 1.6 m, which demonstrates superior performance compared with other disparity refinement methods. Furthermore, the proposed method is able to improve the integrity of the target structure and present steeper building facades and complete roofs, which are conducive to subsequent 3D model generation.
更多查看译文
关键词
open-source GIS data,optical remote sensing images,urban scene,disparity refinement
AI 理解论文
溯源树
样例
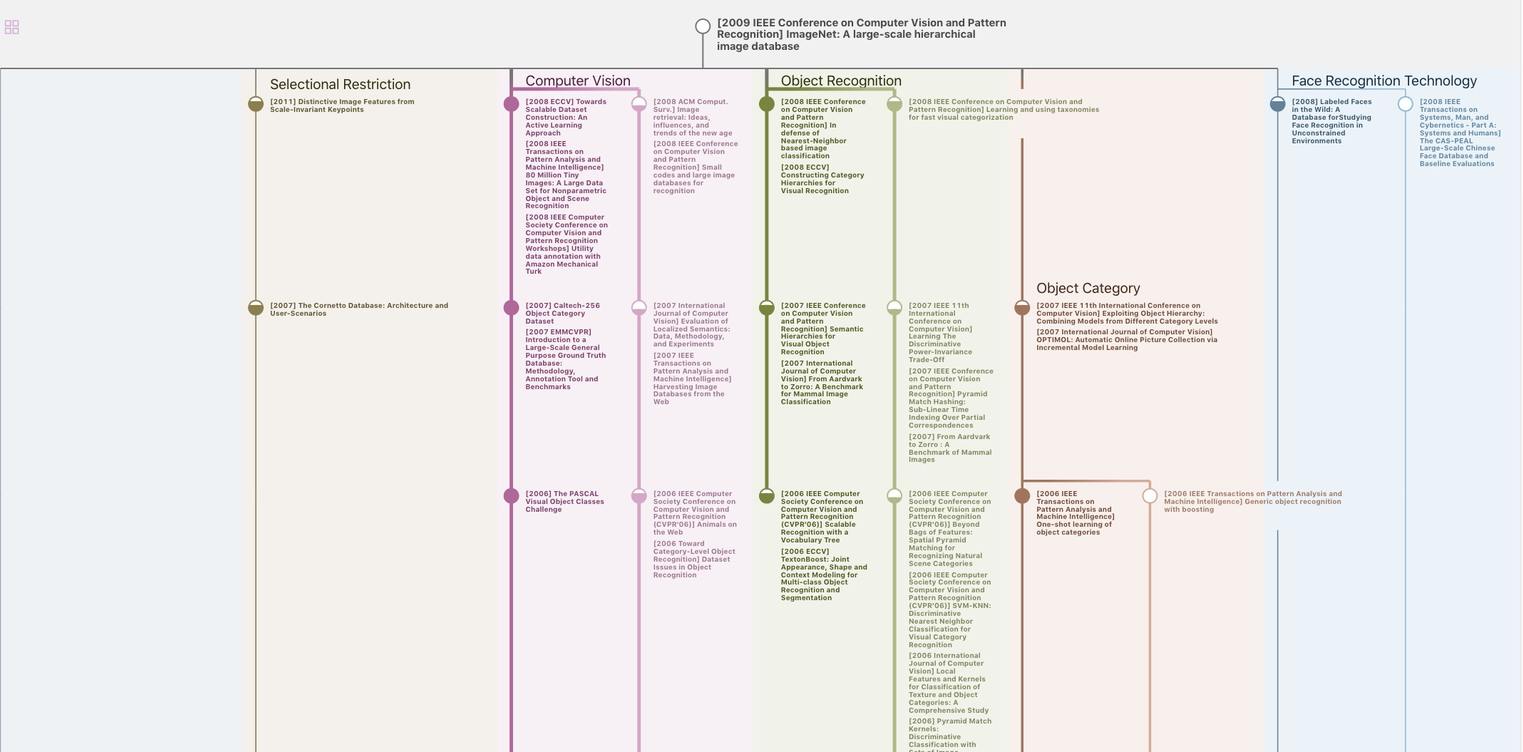
生成溯源树,研究论文发展脉络
Chat Paper
正在生成论文摘要